Iterative subset selection for feature drifting data streams.
SAC 2018: Symposium on Applied Computing Pau France April, 2018(2018)
摘要
Feature selection has been studied and shown to improve classifier performance in standard batch data mining but is mostly unexplored in data stream mining. Feature selection becomes even more important when the relevant subset of features changes over time, as the underlying concept of a data stream drifts. This specific kind of drift is known as feature drift and requires specific techniques not only to determine which features are the most important but also to take advantage of them. This paper presents a novel method of feature subset selection specialized for dealing with the occurrence of feature drifts called Iterative Subset Selection (ISS), which splits the feature selection process into two stages by first ranking the features, and then iteratively selecting features from the ranking. Applying our feature selection method together with Naive Bayes or k-Nearest Neighbour as a classifier, results in compelling accuracy improvements, compared to prior work.
更多查看译文
关键词
Data Stream Mining, Feature Selection, Concept Drift, Embedded Feature Selection, Iterative Subset Selection
AI 理解论文
溯源树
样例
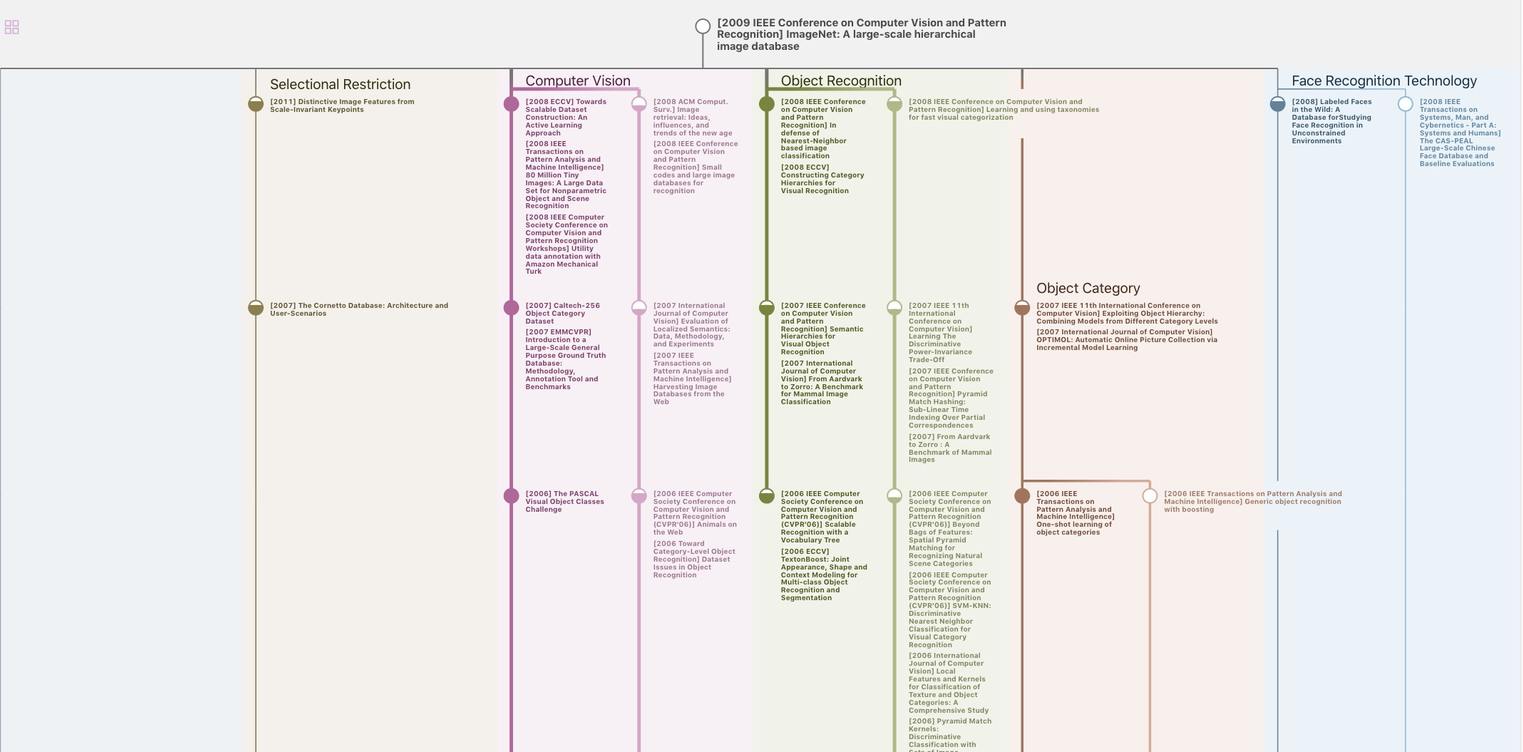
生成溯源树,研究论文发展脉络
Chat Paper
正在生成论文摘要