An Improved CNN Steganalysis Architecture Based on "Catalyst Kernels" and Transfer Learning.
ICDEc(2018)
摘要
In recent years, the interest of using model architectures based on the Convolutional Neural Networks in steganalysis has been rapidly increasing. Regarding the success of deep learning in the field of imaging analysis. Previous approaches have focused on proposing complex architectures with large sizes of images rather than simple models. In this work, we propose a more adjustable flexible architecture with the use of small size images. The robustness of our method is based on using a set of High Pass Filters (HPF) to extract the residual noise on one hand and exploiting the concept of Transfer Learning ensuring the propagation of optimal weights on the other hand. Three state-of-the-art steganographic algorithms in the spatial domain: WOW, S-UNIWARD, and HUGO are used to evaluate the effectiveness of our classification method. Our proposed technique shows an accelerated convergence for the low payloads and provides a better detection accuracy for the high payloads with a classification accuracy rate crossing 96%.
更多查看译文
关键词
improved cnn steganalysis architecture,catalyst kernels”,transfer learning
AI 理解论文
溯源树
样例
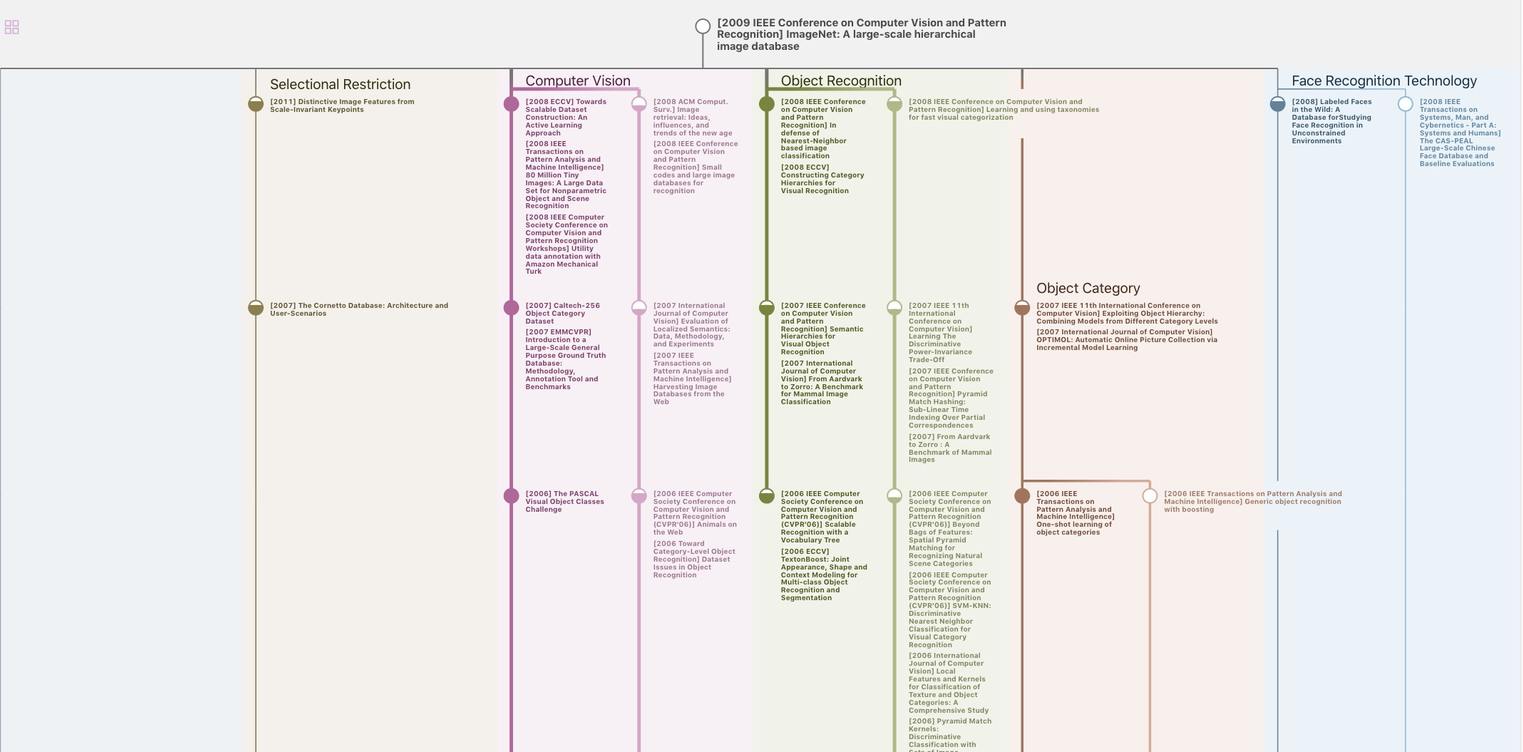
生成溯源树,研究论文发展脉络
Chat Paper
正在生成论文摘要