LARC: Learning Activity-Regularized Overlapping Communities Across Time.
KDD(2018)
摘要
Communities are essential building blocks of complex networks enjoying significant research attention in terms of modeling and detection algorithms. Common across models is the premise that node pairs that share communities are likely to interact more strongly. Moreover, in the most general setting a node may be a member of multiple communities, and thus, interact with more than one cohesive group of other nodes. If node interactions are observed over a long period and aggregated into a single static network, the communities may be hard to discern due to their in-network overlap. Alternatively, if interactions are observed over short time periods, the communities may be only partially observable. How can we detect communities at an appropriate temporal resolution that resonates with their natural periods of activity? We propose LARC, a general framework for joint learning of the overlapping community structure and the periods of activity of communities, directly from temporal interaction data. We formulate the problem as an optimization task coupling community fit and smooth temporal activation over time. To the best of our knowledge, the tensor version of LARC is the first tensor-based community detection method to introduce such smoothness constraints. We propose efficient algorithms for the problem, achieving a $2.6x$ quality improvement over all baselines for high temporal resolution datasets, and consistently detecting better-quality communities for different levels of data aggregation and varying community overlap. In addition, LARC elucidates interpretable temporal patterns of community activity corresponding to botnet attacks, transportation change points and public forum interaction trends, while being computationally practical---few minutes on large real datasets. Finally, LARC provides a comprehensive \em unsupervised parameter estimation methodology yielding high accuracy and rendering it easy-to-use for practitioners.
更多查看译文
关键词
Dynamic graphs,overlapping community detection,tensor factorization,community activation
AI 理解论文
溯源树
样例
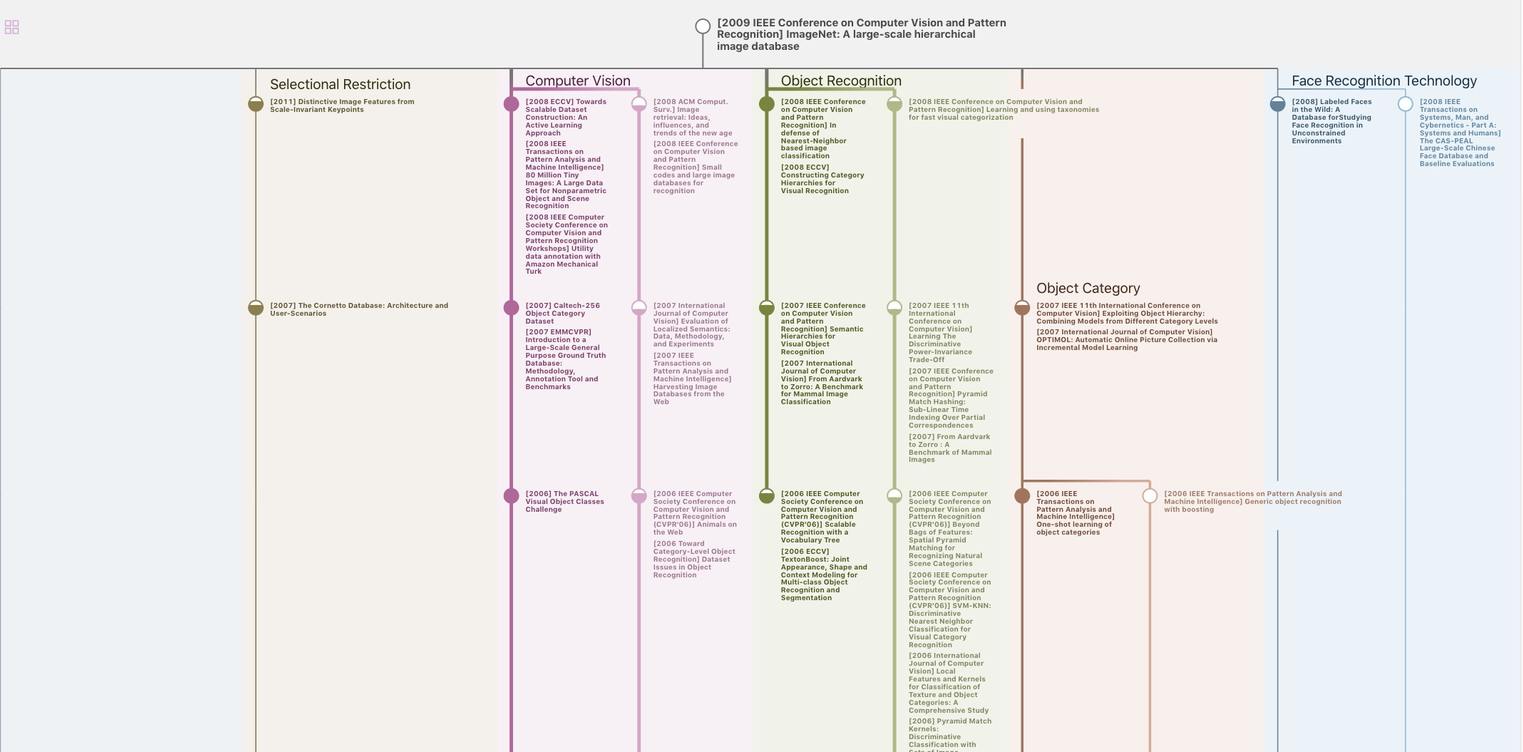
生成溯源树,研究论文发展脉络
Chat Paper
正在生成论文摘要