ISIFT: extracting incremental results from SIFT.
MMSys '18: 9th ACM Multimedia Systems Conference Amsterdam Netherlands June, 2018(2018)
摘要
In computer vision, scale-invariant feature transform (SIFT) remains one of the most commonly used algorithms for feature extraction, but its high computational cost makes it hard to deploy in real-time applications. In this paper, we introduce a novel technique to restructure the inter-octave and intra-octave dependencies of SIFT's keypoint detection and description processes, allowing it to be stopped early and produce approximate results in proportion to the time for which it was allowed to run. If our algorithm is run to completion (about 0.7% longer than traditional SIFT), its results and SIFT's converge. Unlike previous approaches to real-time SIFT, we require no special hardware and make no compromises in keypoint quality, making our technique ideal for real-time and near-real-time applications on resource-constrained systems. We use standard data sets and metrics to analyze the performance of our algorithm and the quality of the generated keypoints.
更多查看译文
关键词
SIFT, feature extraction, real-time systems, adaptive systems, multimedia IoT
AI 理解论文
溯源树
样例
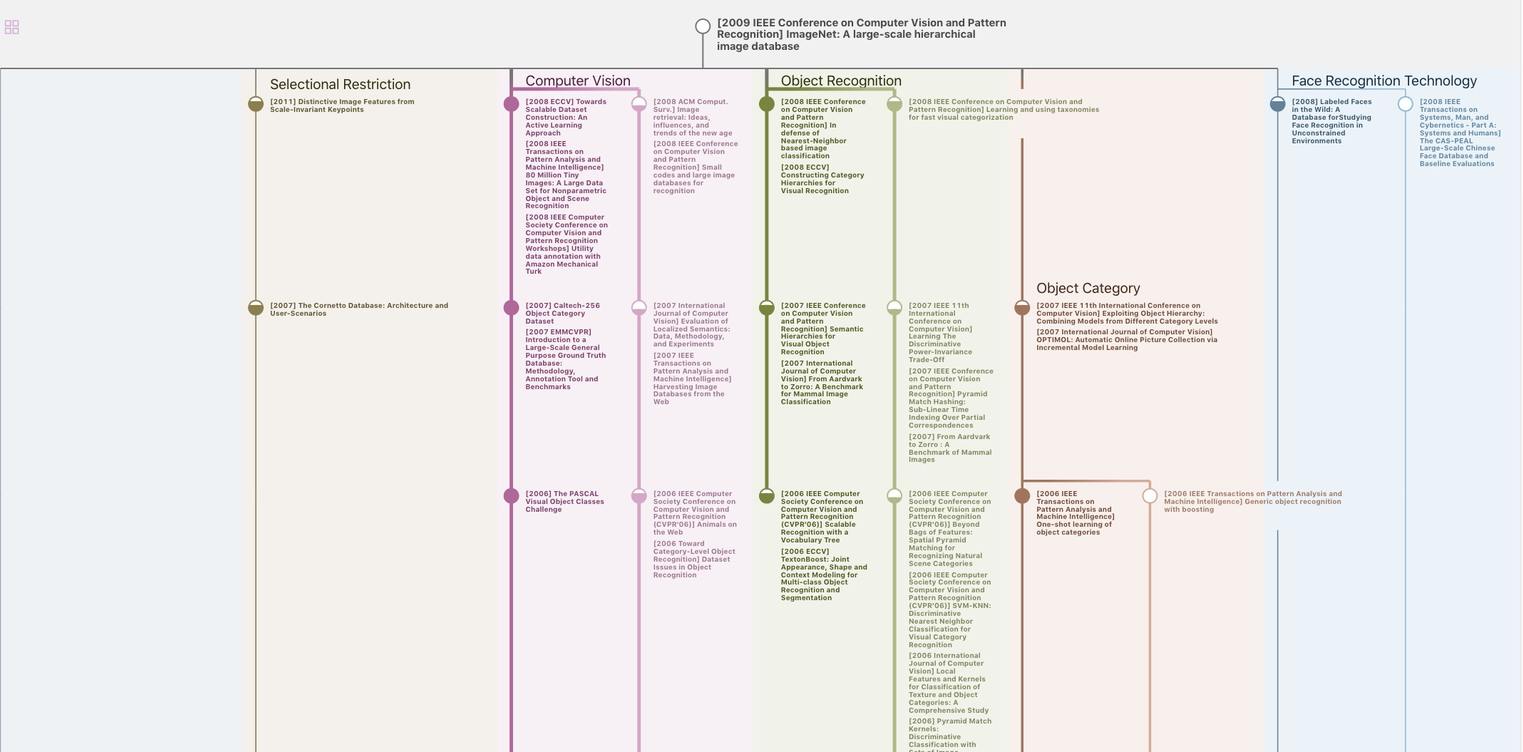
生成溯源树,研究论文发展脉络
Chat Paper
正在生成论文摘要