Autonomous Model Management via Reinforcement Learning.
AAAI Workshops(2018)
摘要
Concept drift - a change, either sudden or gradual, in the underlying properties of data - is one of the most prevalent challenges to maintaining high-performing learned models over time in autonomous systems. In the face of concept drift, one can hope that the old model is sufficiently representative despite concept drift. Alternatively, one can discard the old data and retrain a new model with (often limited) new data, or use transfer learning to combine the old data with the new to create an updated model. Which of these three options is chosen affects not only near-term decisions, but also future modeling actions. In this abstract, we model response to concept drift as a sequential decision making problem and formally frame it as a Markov Decision Process. Our reinforcement learning approach to the problem shows promising results balancing cost with performance in maintaining model accuracy.
更多查看译文
AI 理解论文
溯源树
样例
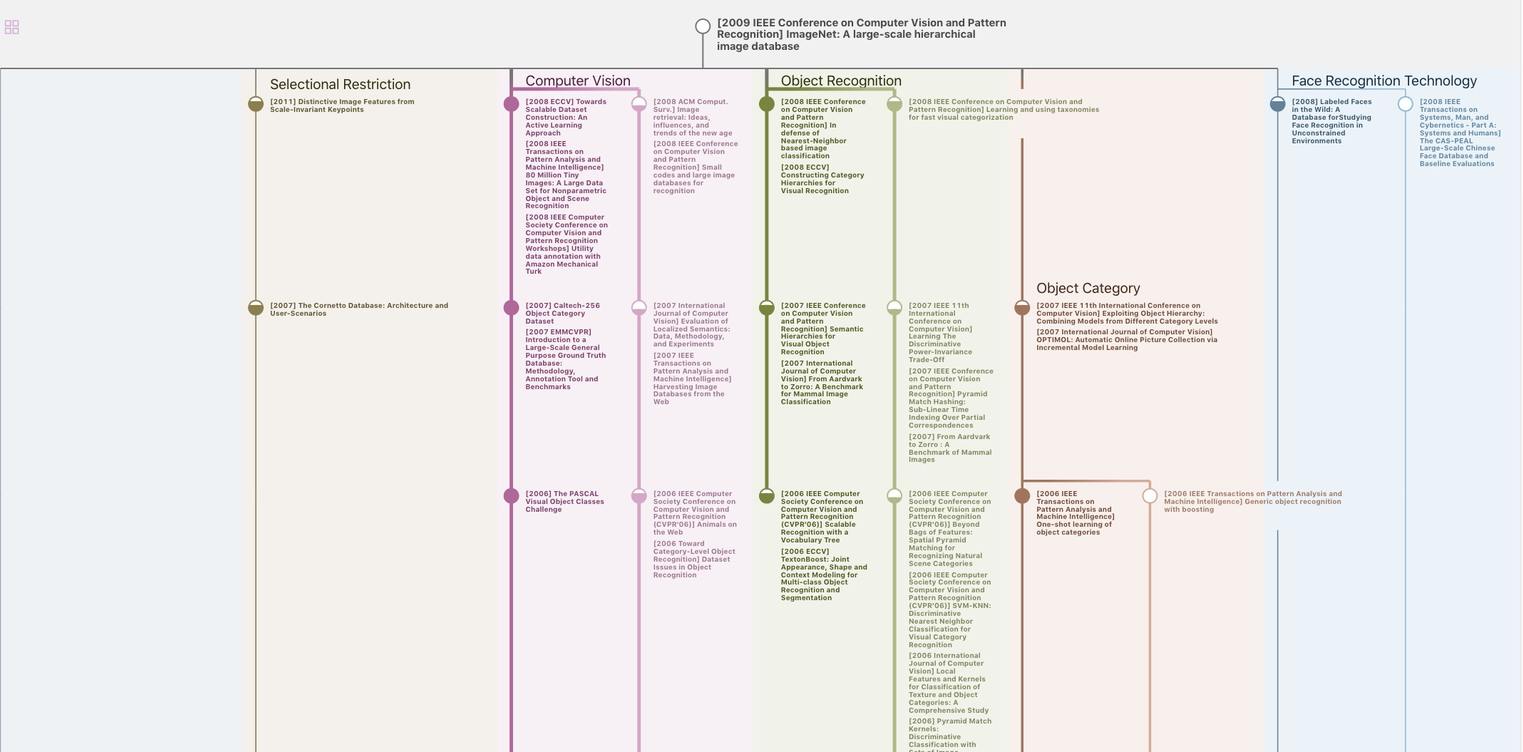
生成溯源树,研究论文发展脉络
Chat Paper
正在生成论文摘要