Similarity-Adaptive Latent Low-Rank Representation For Robust Data Representation
PRICAI 2018: TRENDS IN ARTIFICIAL INTELLIGENCE, PT I(2018)
摘要
We propose a novel Similarity-Adaptive Latent Low-Rank Representation (SA-LatLRR) model for the robust representation and subspace recovery. SA-LatLRR inherits all merits of recent LatLRR, and further improves it by enhancing the representations. SA-LatLRR aims at decomposing given data into a principal feature part encoded by the Frobenius-norm based coefficients, a similarity-adaptive salient feature part and a sparse error part. Specifically, our SA-LatLRR incorporates a reconstructive error minimization term over coefficients and salient features, which can clearly preserve the neighborhood information of salient features adaptively. The added regularization can also encourage the coefficients to be block-diagonal and discriminative, as the shared coefficients could minimize the reconstruction errors over both original data and salient features at the same time, where the embedded salient features contain less noise and unfavorable features than the original data. Moreover, to make salient features more informative and robust to noise, SA-LatLRR imposes the sparse L2,1-norm and low-rank constraints on the projection jointly so that the features are more notable and discriminative. The Frobenius-norm based principal feature part can also make the coefficients coding process very efficient. Extensive comparison results demonstrate the validity of our SA-LatLRR.
更多查看译文
关键词
Similarity-adaptive latent LRR, Robust data representation, Salient feature extraction, Classification
AI 理解论文
溯源树
样例
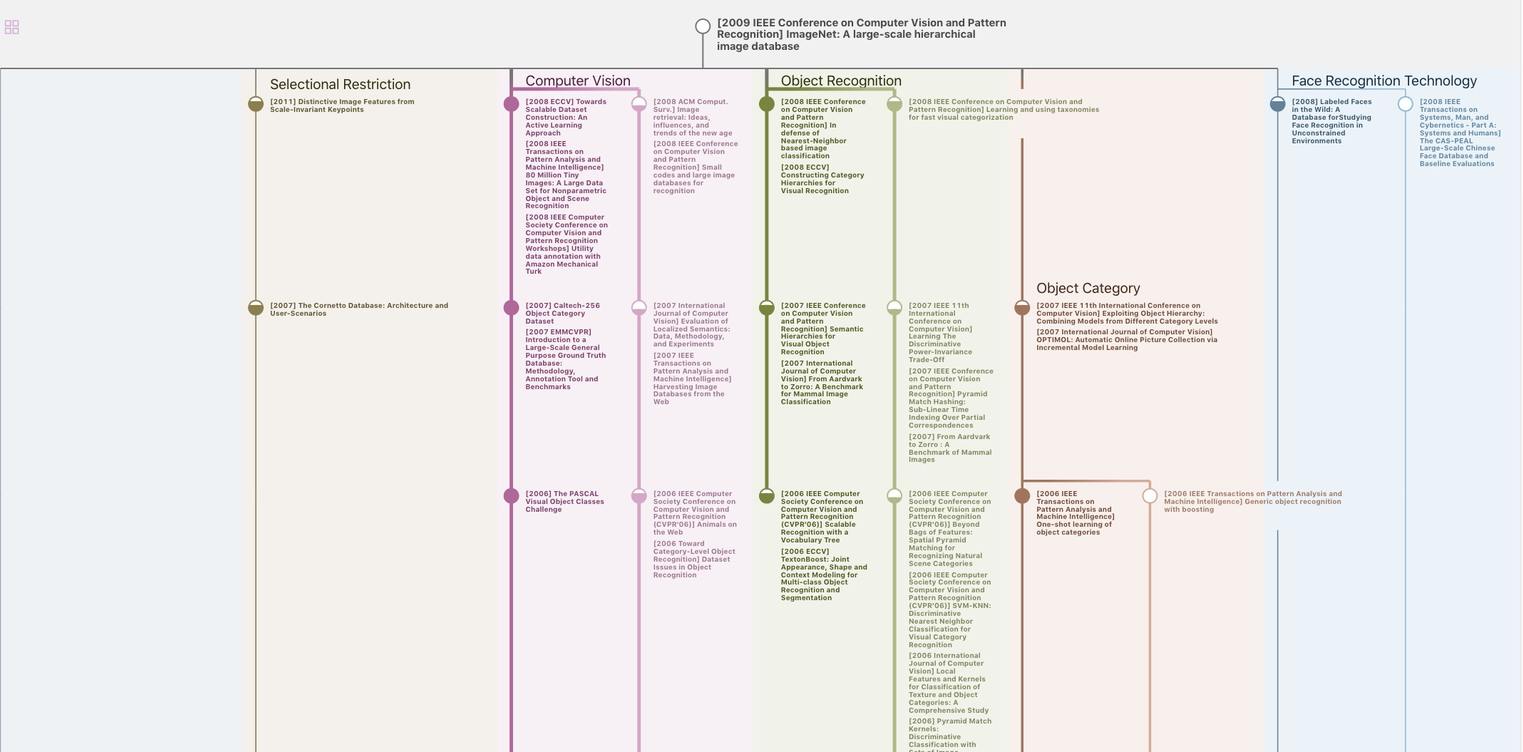
生成溯源树,研究论文发展脉络
Chat Paper
正在生成论文摘要