An adaptable deep learning system for optical character verification in retail food packaging
2018 IEEE Conference on Evolving and Adaptive Intelligent Systems (EAIS)(2018)
摘要
Retail food packages contain various types of information such as food name, ingredients list and use by dates. Such information is critical to ensure proper distribution of products to the market and eliminate health risks due to erroneous mislabelling. The latter is considerably detrimental to both consumers and suppliers alike. In this paper, an adaptable deep learning based system is proposed and tested across various possible scenarios: a) for the identification of blurry images and/or missing information from food packaging photos. These were captured during the validation process in supply chains; b) for deep neural network adaptation. This was achieved through a novel methodology that utilises facets of the same convolutional neural network architecture. Latent variables were extracted from different datasets and used as input into a Λ-means clustering and Λ-nearest neighbour classification algorithm, to compute a new set of centroids which better adapts to the target dataset's distribution. Furthermore, visualisation and analysis of network adaptation provides insight into how higher accuracy was achieved when compared to the original deep neural network. The proposed system performed very well in the conducted experiments, showing that it can be deployed in real-world supply chains, for automating the verification process, cutting down costs and eliminating errors that could prove risky for public health.
更多查看译文
关键词
deep learning,convolutional neural networks,clustering,trained representations,adaptation,optical character verification,retail food packages
AI 理解论文
溯源树
样例
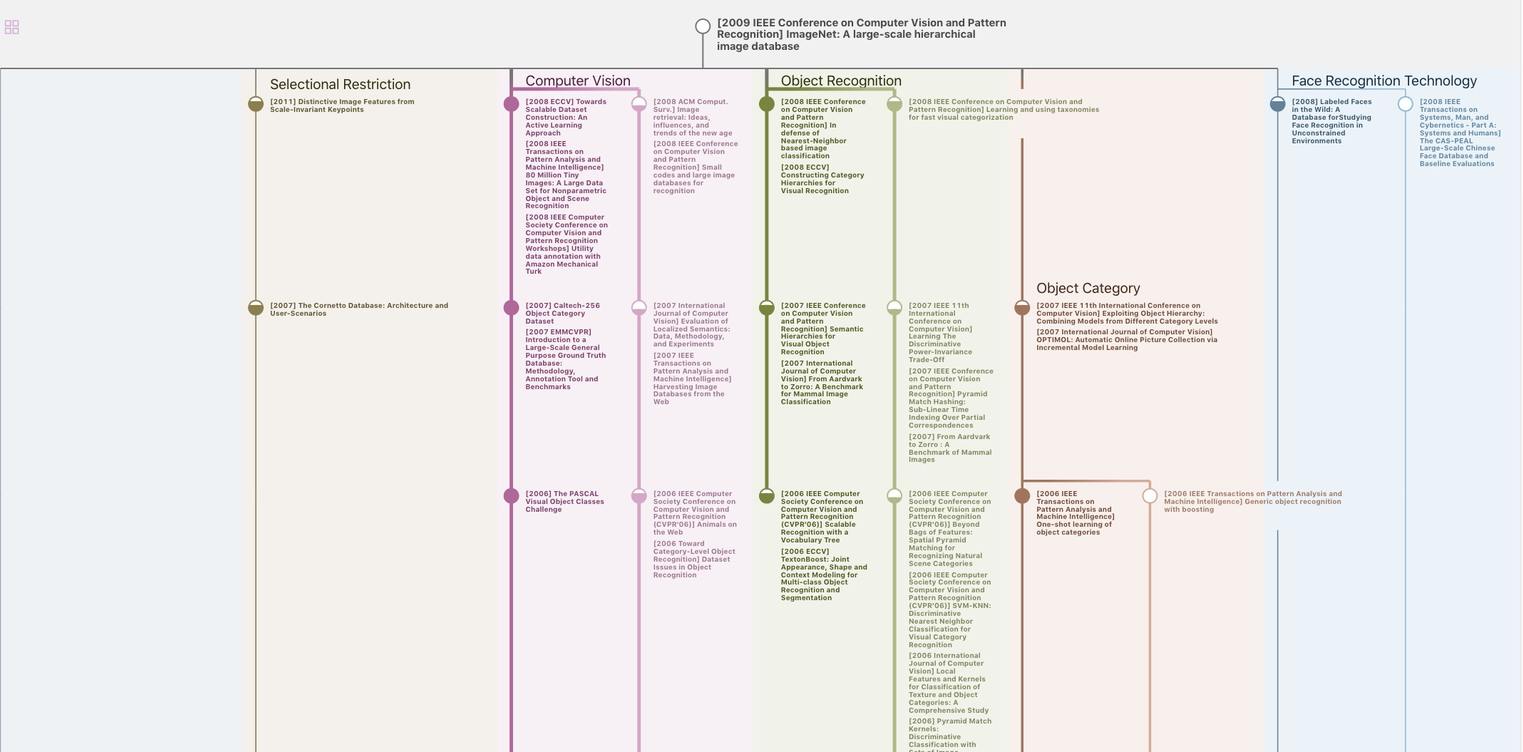
生成溯源树,研究论文发展脉络
Chat Paper
正在生成论文摘要