Explanations That Are Intrinsic To Recommendations
PROCEEDINGS OF THE 26TH CONFERENCE ON USER MODELING, ADAPTATION AND PERSONALIZATION (UMAP'18)(2018)
摘要
Explanations can give credibility to recommendations and help users to make better choices. In current recommender systems, explanation is a step that comes after recommendation. In this paper, we describe an approach that turns recommender systems on their head. In our approach, which we call Recommendation-by-Explanation (r-by-e), the system constructs a reason, or explanation, for recommending each candidate item; then it recommends those candidate items that have the best explanations. By unifying recommendation and explanation, r-by-e finds relevant recommendations with explanations that have a high degree of fidelity.We present the results of an offline experiment using a movie recommendation dataset. We show that r-by-e achieves higher precision than a comparable recommender, while both produce recommendations with roughly equal levels of diversity and serendipity.We also present the results of deploying a web-based system through which we have conducted two user trials. In one trial, we evaluate recommendation quality. Participants in this trial found r-by-e's recommendations to be more diverse, serendipitous and relevant than those of the competitor system. In another trial, we evaluate explanation quality. We used a re-rating task: users rated recommendations initially in the case where they were given only the explanation and not the identity of the movie, and then re-rated in the case where they were given information about the recommended movie. We found a stronger correlation between the pairs of ratings in the case of r-by-e. This suggests that r-by-e's explanations allow users to make more accurate judgments about the quality of recommended items.
更多查看译文
关键词
Explanation, Recommendation, User Trial
AI 理解论文
溯源树
样例
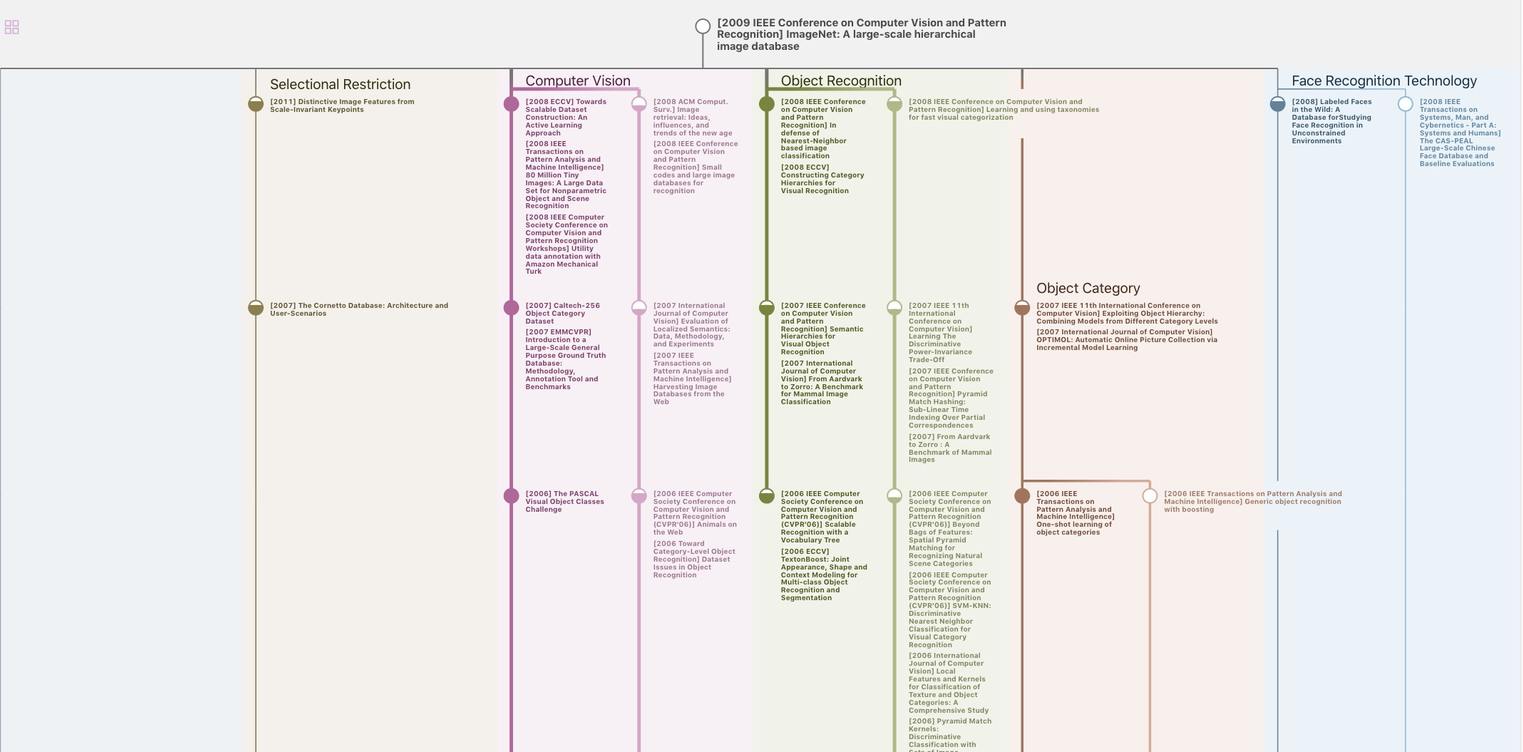
生成溯源树,研究论文发展脉络
Chat Paper
正在生成论文摘要