Predicting Accuracy On Large Datasets From Smaller Pilot Data
PROCEEDINGS OF THE 56TH ANNUAL MEETING OF THE ASSOCIATION FOR COMPUTATIONAL LINGUISTICS, VOL 2(2018)
摘要
Because obtaining training data is often the most difficult part of an NLP or ML project, we develop methods for predicting how much data is required to achieve a desired test accuracy by extrapolating results from systems trained on a small pilot training dataset. We model how accuracy varies as a function of training size on subsets of the pilot data, and use that model to predict how much training data would be required to achieve the desired accuracy. We introduce a new performance extrapolation task to evaluate how well different extrapolations predict system accuracy on larger training sets. We show that details of hyperparameter optimisation and the extrapolation models can have dramatic effects in a document classification task. We believe this is an important first step in developing methods for estimating the resources required to meet specific engineering performance targets.
更多查看译文
AI 理解论文
溯源树
样例
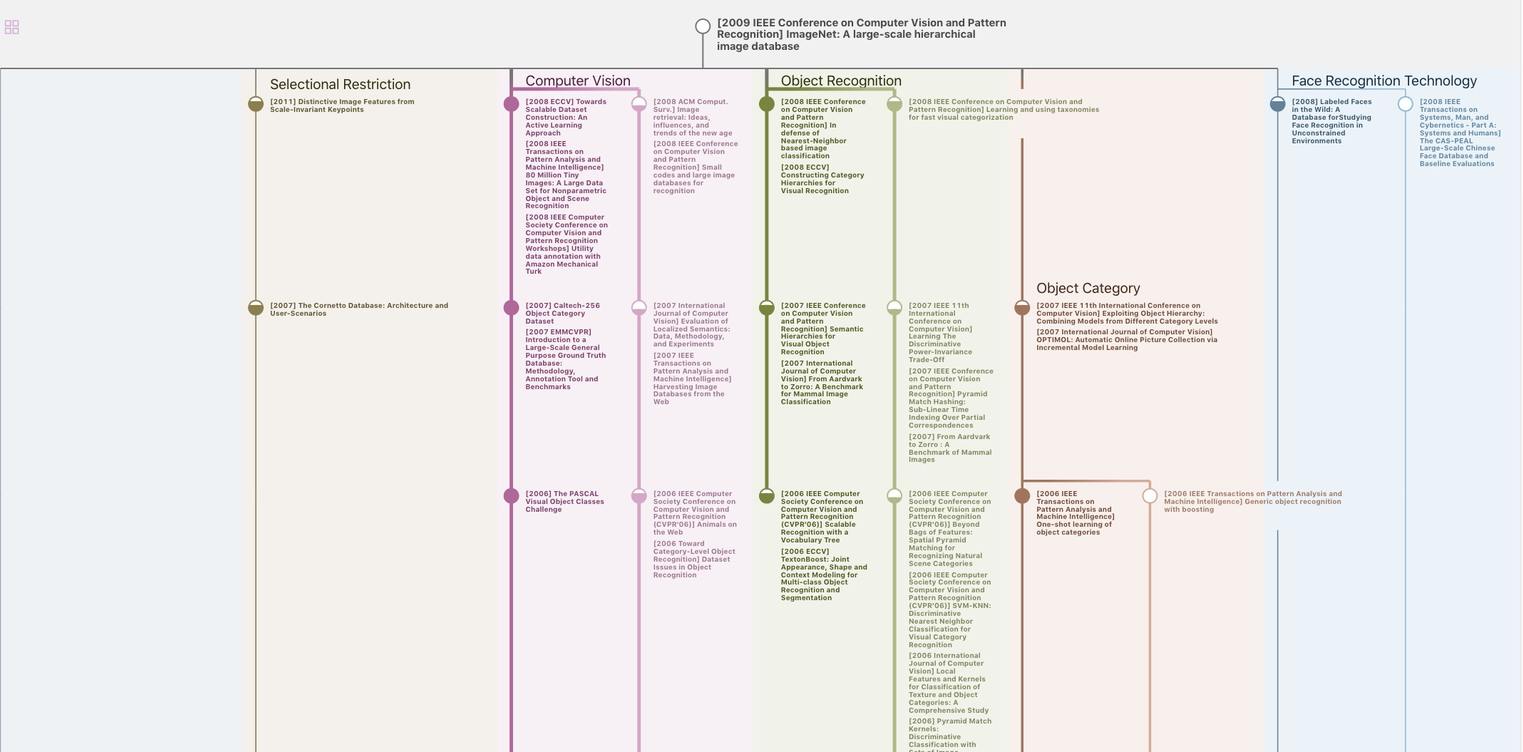
生成溯源树,研究论文发展脉络
Chat Paper
正在生成论文摘要