Automated diagnostic of virtualized service performance degradation.
IEEE IFIP Network Operations and Management Symposium(2018)
摘要
Service assurance for cloud applications is a challenging task and is an active area of research for academia and industry. One promising approach is to utilize machine learning for service quality prediction and fault detection so that suitable mitigation actions can be executed. In our previous work, we have shown how to predict service-level metrics in real-time just from operational data gathered at the server side. This gives the service provider early indications on whether the platform can support the current load demand. This paper provides the logical next step where we extend our work by proposing an automated detection and diagnostic capability for the performance faults manifesting themselves in cloud and datacenter environments. This is a crucial task to maintain the smooth operation of running services and minimizing downtime. We demonstrate the effectiveness of our approach which exploits the interpretative capabilities of Self-Organizing Maps (SOMs) to automatically detect and localize different performance faults for cloud services.
更多查看译文
关键词
machine learning,service quality,system statistics,video streaming,fault detection,fault localization
AI 理解论文
溯源树
样例
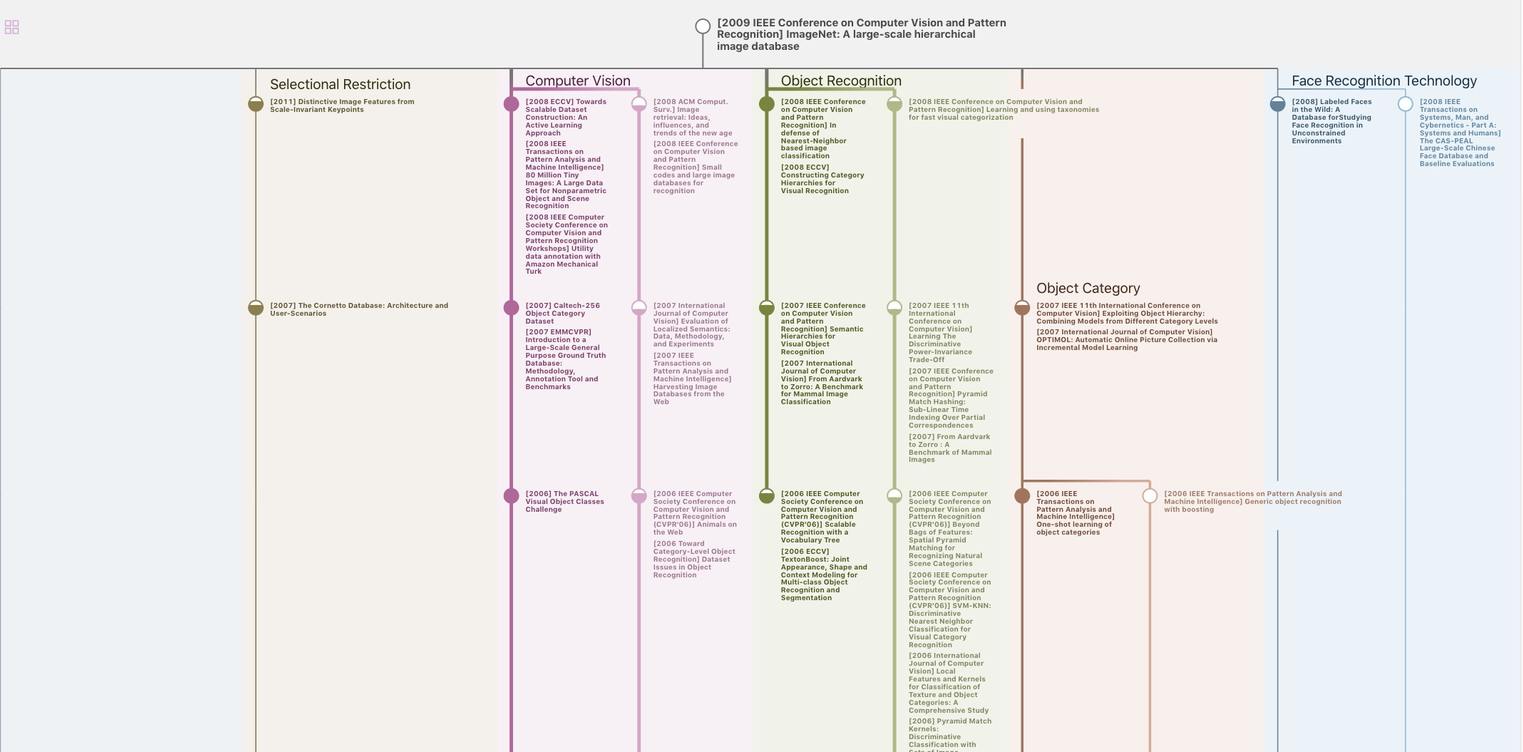
生成溯源树,研究论文发展脉络
Chat Paper
正在生成论文摘要