Divide and conquer: neuroevolution for multiclass classification.
GECCO(2018)
摘要
Neuroevolution is a powerful and general technique for evolving the structure and weights of artificial neural networks. Though neuroevolutionary approaches such as NeuroEvolution of Augmenting Topologies (NEAT) have been successfully applied to various problems including classification, regression, and reinforcement learning problems, little work has explored application of these techniques to larger-scale multiclass classification problems. In this paper, NEAT is evaluated in several multiclass classification problems, and then extended via two ensemble approaches: One-vs-All and One-vs-One. These approaches decompose multiclass classification problems into a set of binary classification problems, in which each binary problem is solved by an instance of NEAT. These ensemble models exhibit reduced variance and increasingly superior accuracy as the number of classes increases. Additionally, higher accuracy is achieved early in training, even when artificially constrained for the sake of fair comparison with standard NEAT. However, because the approach can be trivially distributed, it can be applied quickly at large scale to solve real problems. In fact, these approaches are incorporated into DarwinTM, an enterprise automatic machine learning solution that also incorporates various other algorithmic enhancements to NEAT. The resulting complete system has proven robust to a wide variety of client datasets.
更多查看译文
关键词
Neuroevolution, Ensembling, Neural Networks
AI 理解论文
溯源树
样例
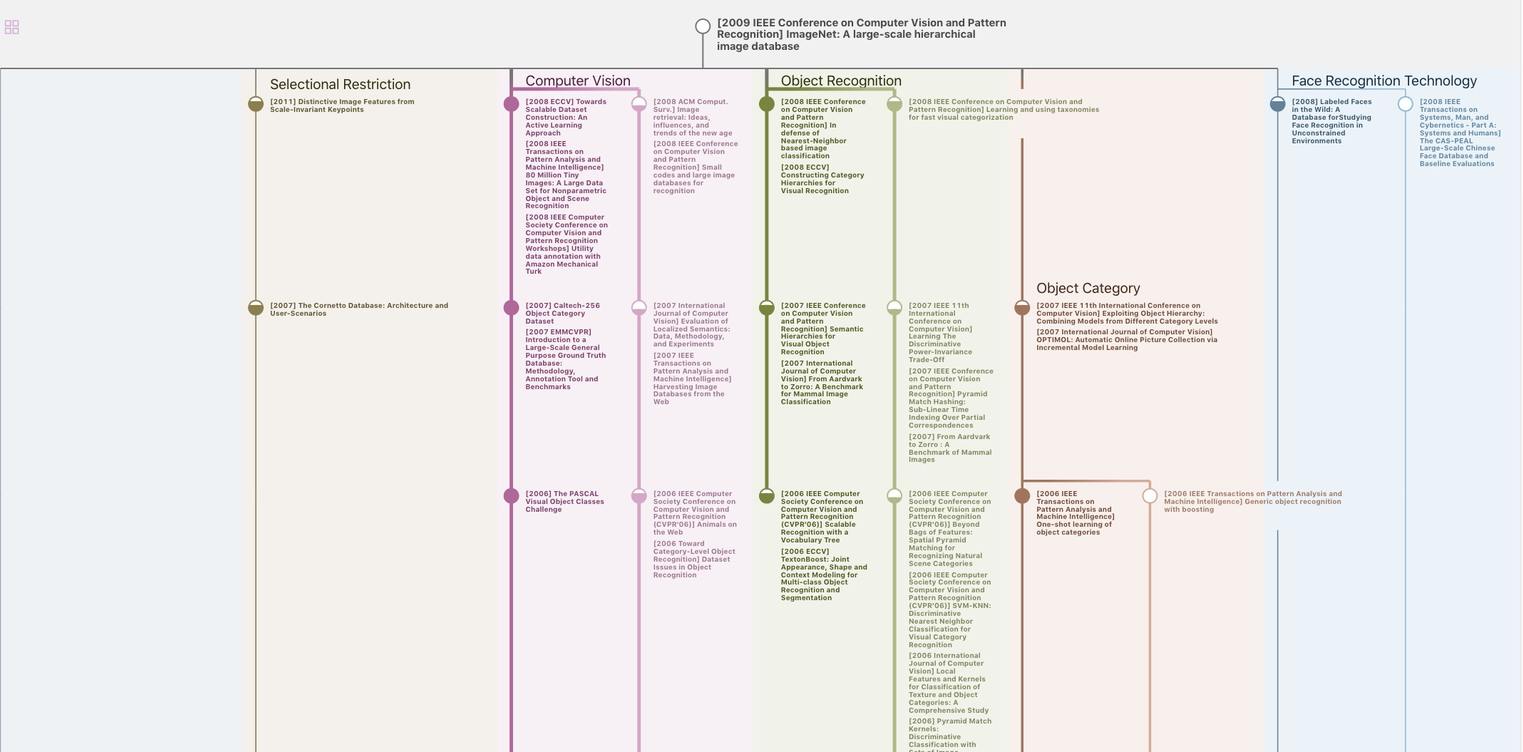
生成溯源树,研究论文发展脉络
Chat Paper
正在生成论文摘要