Investigation of the latent space of stock market patterns with genetic programming.
GECCO(2018)
摘要
We suggest a use of genetic programming for transformation from a vector space to an understandable graph representation, which is part of a project to inspect the latent space in matrix factorization. Given a relation matrix, we can apply standard techniques such as non-negative matrix factorization to extract low dimensional latent space in vector representation. While the vector representation of the latent space is useful, it is not intuitive and hard to interpret. The transformation with the help of genetic programming allows us to better understand the underlying latent structure. Applying the method in the context of a stock market, we show that it is possible to recover the tree representation of technical patterns from a relation matrix. Leveraging the properties of the vector representations, we are able to find patterns that correspond to cluster centers of technical patterns. We further investigate the geometry of the latent space.
更多查看译文
关键词
matrix factorization, latent space models, technical patterns, genetic programming
AI 理解论文
溯源树
样例
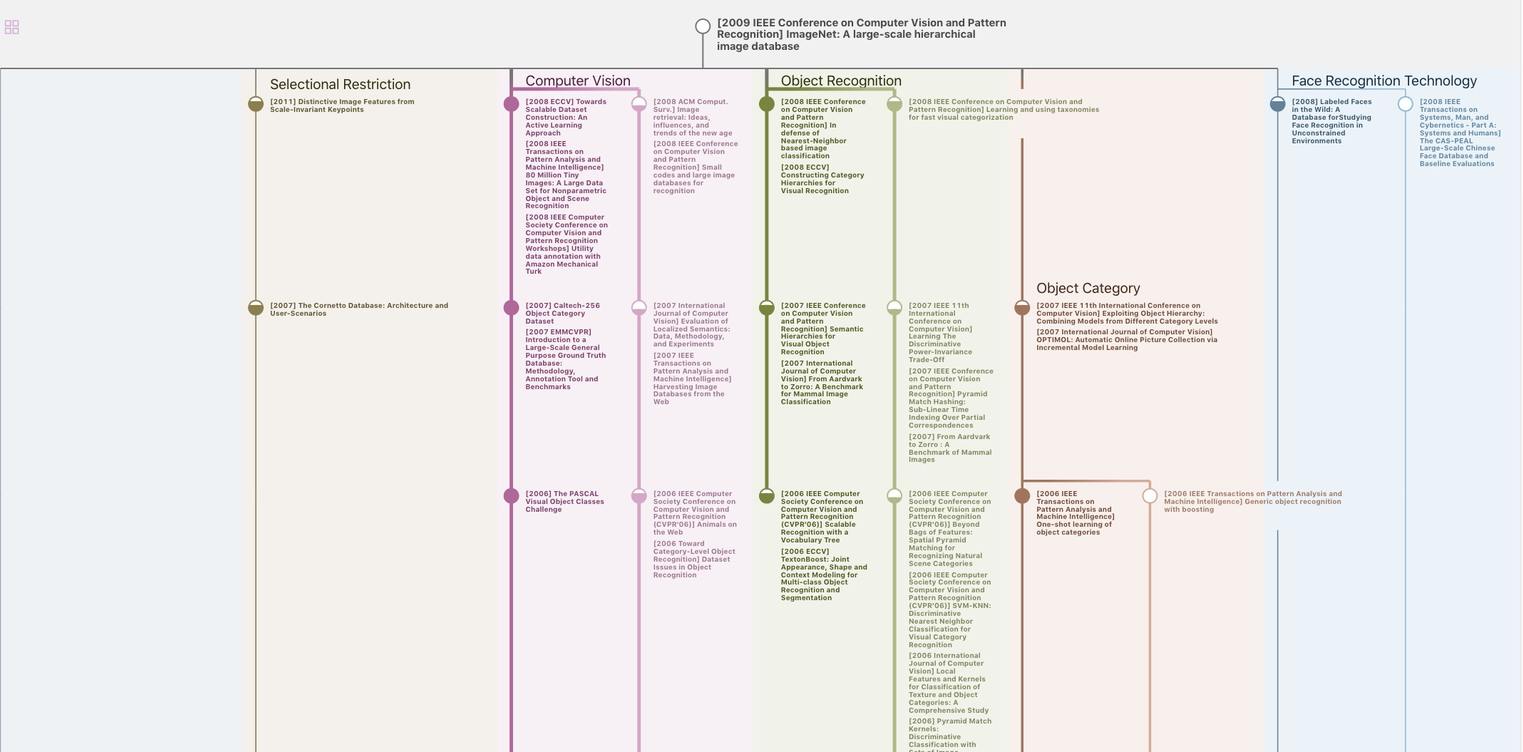
生成溯源树,研究论文发展脉络
Chat Paper
正在生成论文摘要