Studying MOEAs dynamics and their performance using a three compartmental model.
GECCO (Companion)(2018)
摘要
The road to a better design of multi- and many-objective evolutionary algorithms requires a deeper understanding of their behavior. A step on this road has recently been taken with the proposal of compartmental models to study population dynamics. In this work, we push this step further by introducing a new set of features that we link with algorithm performance. By tracking the number of newly discovered Pareto Optimal (PO) solutions, the previously-found PO solutions and the remaining non-PO solutions, we can track the algorithm progression. By relating these features with a performance measure, such as the hypervolume, we can analyze their relevance for algorithm comparison. This study considers out-of-the-box implementations of recognized multi- and many-objective optimizers belonging to popular classes such as conventional Pareto dominance, extensions of dominance, indicator, and decomposition based approaches. In order to generate training data for the compartmental models, we consider multiple instances of MNK-landscapes with different numbers of objectives.
更多查看译文
关键词
moeas dynamics,compartmental model
AI 理解论文
溯源树
样例
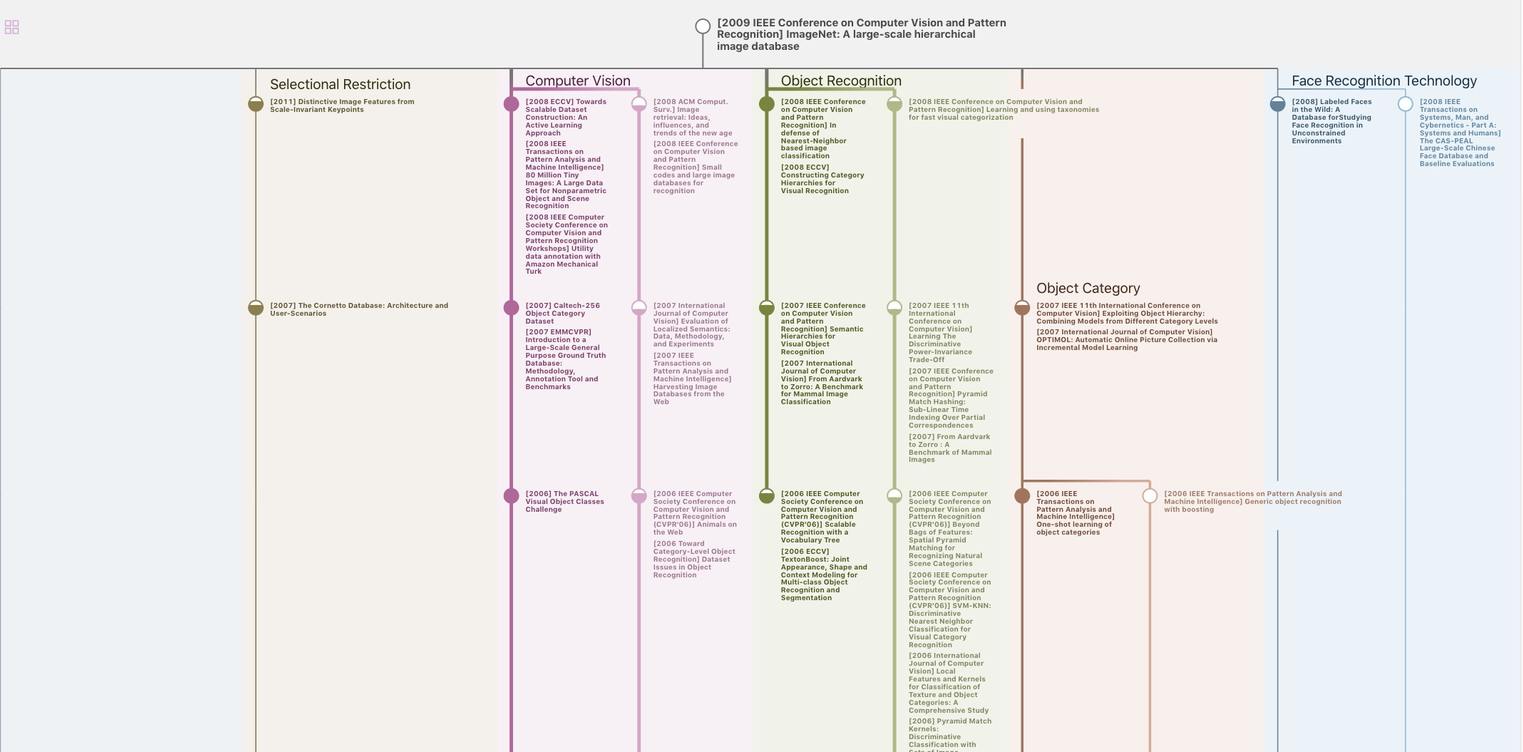
生成溯源树,研究论文发展脉络
Chat Paper
正在生成论文摘要