Adaptive Incentive Selection For Crowdsourcing Contests
PROCEEDINGS OF THE 17TH INTERNATIONAL CONFERENCE ON AUTONOMOUS AGENTS AND MULTIAGENT SYSTEMS (AAMAS' 18)(2018)
摘要
The success of crowdsourcing projects relies critically on motivating the crowd to contribute. One particularly effective method for incentivising participants to perform tasks is to run contests. However, there are numerous ways to implement such contests in specific projects (that vary in how performance is evaluated, how to reward, and the sizes of the prizes). Additionally, with a given financial budget and a time limit, choosing incentives that maximise the total outcome (e.g., the total number of completed tasks) is not trivial, as their effectiveness in a specific project is usually unknown in advance. Therefore, we introduce algorithms to select such incentives effectively using budgeted multi-armed bandits. To do that, we first introduce the incentive selection problem, then formalise it as a 2d-budgeted multi-armed bandit, where each arm corresponds to an incentive (i.e., a contest with a specific structure). We then propose the HAIS and Stepped e-first algorithms to solve the incentive selection problem. The two algorithms are shown to be effective on simulations with synthetic data. Stepped e-first performs well, but requires a situation-specific parameter to be tuned appropriately (which may be difficult in settings with little prior experience). In contrast to this, HAIS performs better in most cases without depending significantly on the parameter tuning.
更多查看译文
关键词
incentive, crowdsourcing contest, budgeted MAB
AI 理解论文
溯源树
样例
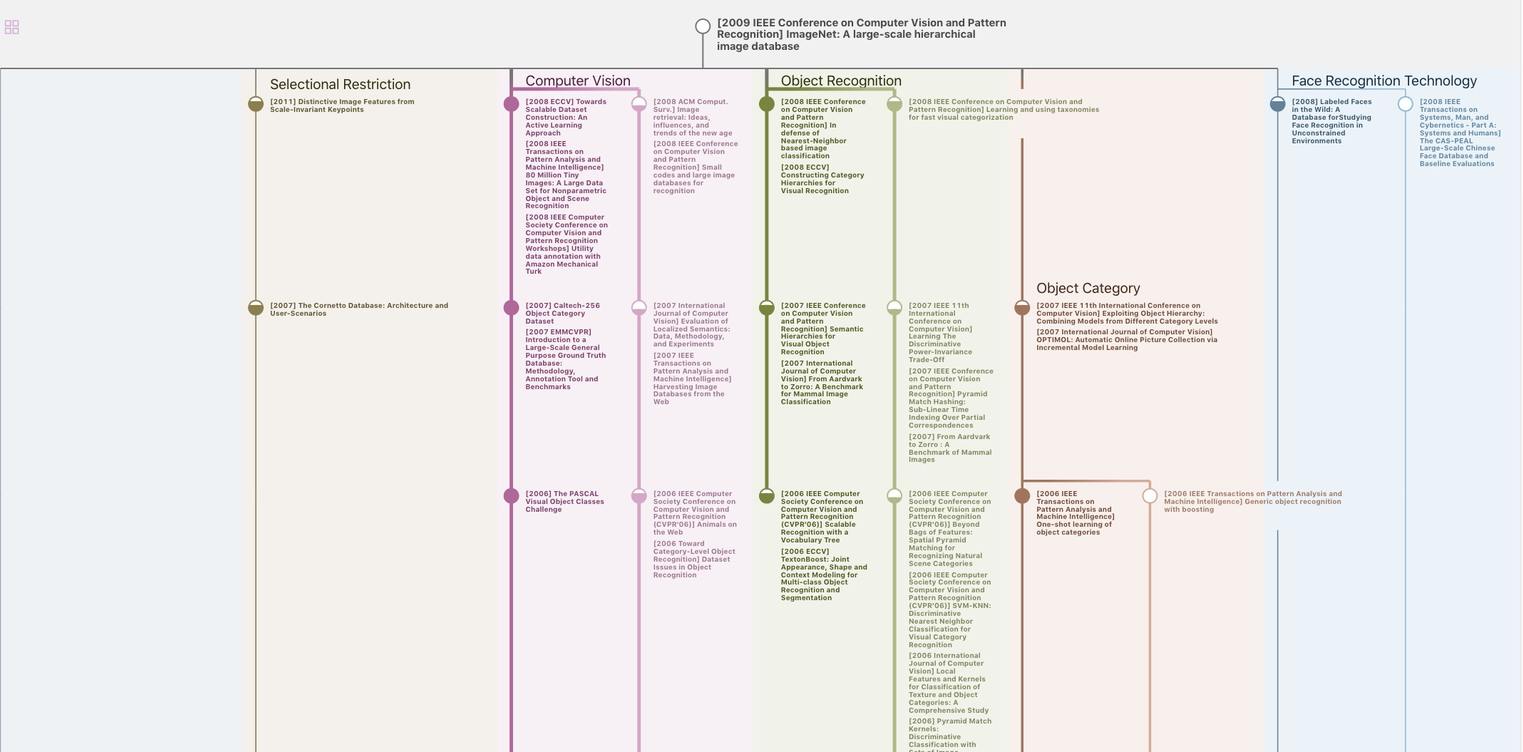
生成溯源树,研究论文发展脉络
Chat Paper
正在生成论文摘要