Faster Policy Adaptation In Environments With Exogeneity: A State Augmentation Approach
PROCEEDINGS OF THE 17TH INTERNATIONAL CONFERENCE ON AUTONOMOUS AGENTS AND MULTIAGENT SYSTEMS (AAMAS' 18)(2018)
摘要
The reinforcement learning literature typically assumes fixed state transition functions for the sake of tractability. However, in many real-world tasks, the state transition function changes over time, and this change may be governed by exogenous variables outside of the control loop. This can make policy learning difficult. In this paper, we propose a new algorithm to address the aforementioned challenge by embedding the state transition functions at different timestamps into a Reproducing Kernel Hilbert Space; the exogenous variable, as the cause of the state transition evolution, is estimated by projecting the embeddings into the subspace that preserves maximum variance. By augmenting the observable state vector with the estimated exogenous variable, standard RL algorithms such as Q-learning are able to learn faster and better. Experiments with both synthetic and real data demonstrate the superiority of our proposed algorithm over standard and advanced variants of Q-learning algorithms in dynamic environments.
更多查看译文
关键词
Reinforcement learning, Q-learning, environments with exogeneity
AI 理解论文
溯源树
样例
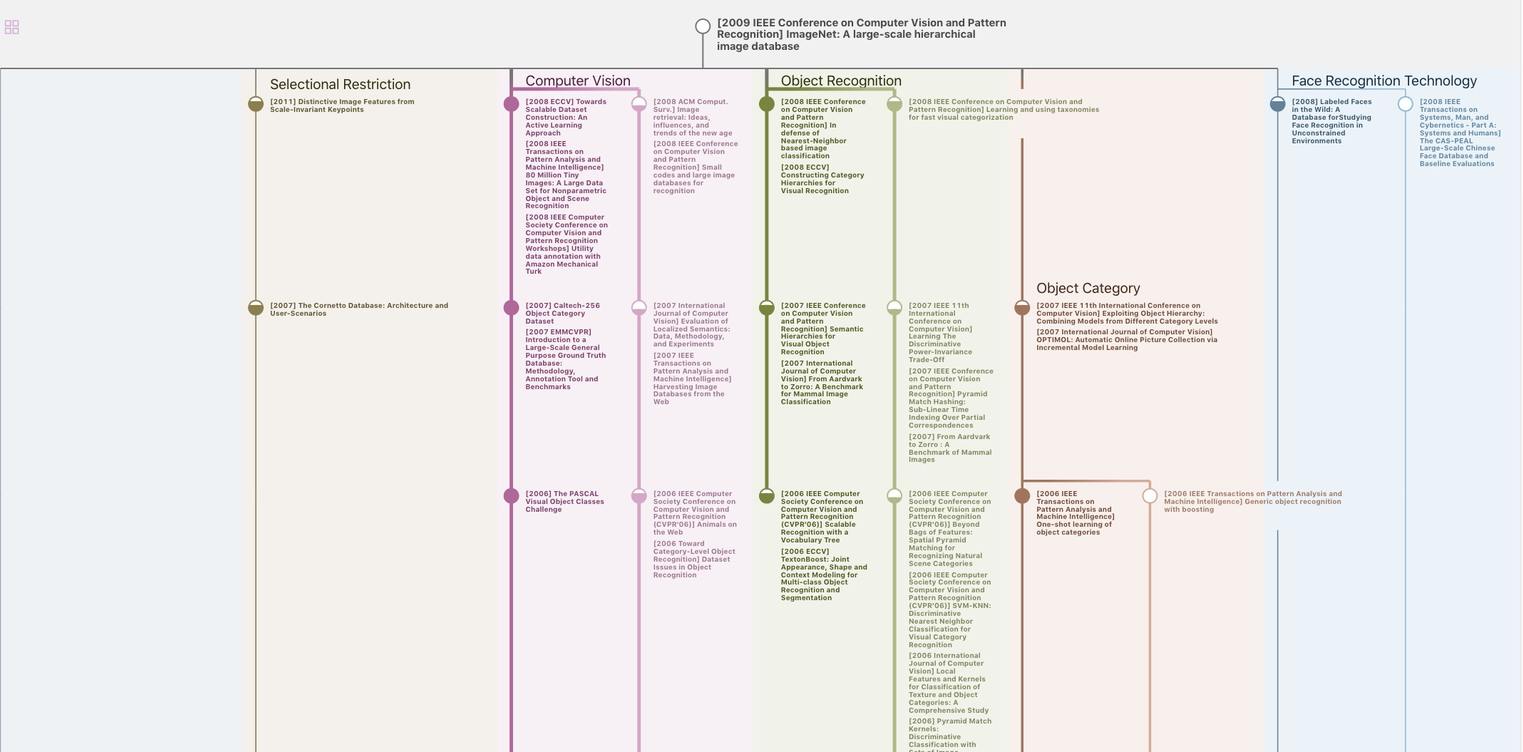
生成溯源树,研究论文发展脉络
Chat Paper
正在生成论文摘要