A Geometric Least Squares Method For Peer Assessment
PROCEEDINGS OF THE 17TH INTERNATIONAL CONFERENCE ON AUTONOMOUS AGENTS AND MULTIAGENT SYSTEMS (AAMAS' 18)(2018)
摘要
In the peer assessment problem, a set of agents give evaluations to each other, and we are going to combine these peer assessments together to construct an overall evaluation. In this paper, we propose a geometric least squares method (GLS) to find an aggregate scoring overall agents for the peer assessment problem. Our method is based on the following observation. Since each agent has a missing score that should be given by itself, we consider the missing score as a variable and then each agent can be regarded as a line in an n-dimensional vector space. The final aggregate scores of the agents can be regarded as points on a line vector, called the projection vector. Thus, we treat the peer assessment problem as an optimization problem of selecting a projection vector with minimum total squared distance to all the lines representing the agents. We will see that this aggregate method has some advantages compared with the simple average method. One advantage is that, when the scores given by each agent (even ignoring the magnitude of the agent) are close to a groundtruth, the new method finds the groundtruth with the highest expectation.
更多查看译文
关键词
Social Choice, Score Aggregation, Peer Assessment
AI 理解论文
溯源树
样例
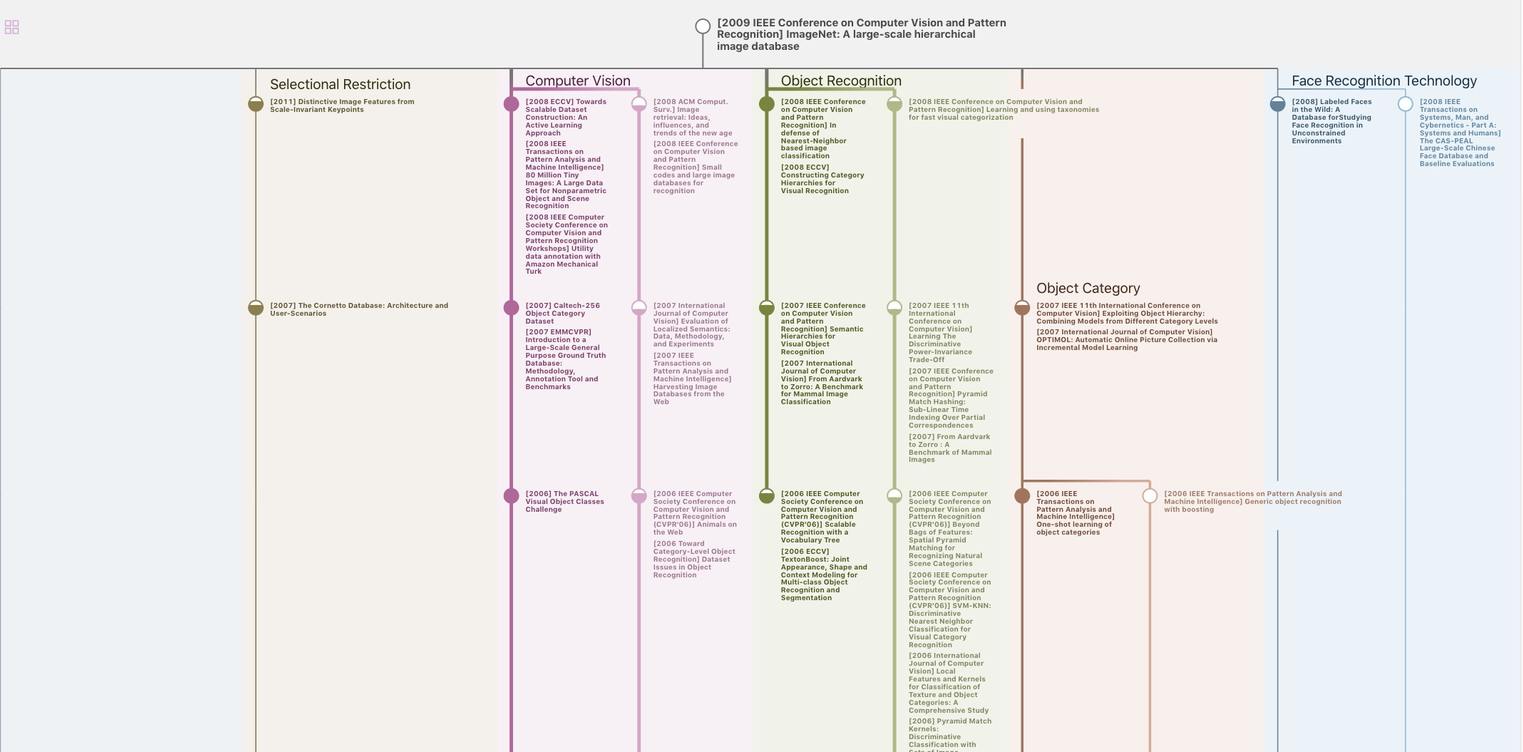
生成溯源树,研究论文发展脉络
Chat Paper
正在生成论文摘要