Performance Characterization of Spark Workloads on Shared NUMA Systems
2018 IEEE Fourth International Conference on Big Data Computing Service and Applications (BigDataService)(2018)
摘要
As the adoption of Big Data technologies becomes the norm in an increasing number of scenarios, there is also a growing need to optimize them for modern processors. Spark has gained momentum over the last few years among companies looking for high performance solutions that can scale out across different cluster sizes. At the same time, modern processors can be connected to large amounts of physical memory, in the range of up to few terabytes. This opens an enormous range of opportunities for runtimes and applications that aim to improve their performance by leveraging low latencies and high bandwidth provided by RAM. The result is that there are several examples today of applications that have started pushing the in-memory computing paradigm to accelerate tasks. To deliver such a large physical memory capacity, hardware vendors have leveraged Non-Uniform Memory Architectures (NUMA). This paper explores how Spark-based workloads are impacted by the effects of NUMA-placement decisions, how different Spark configurations result in changes in delivered performance, how the characteristics of the applications can be used to predict workload collocation conflicts, and how to improve performance by collocating workloads in scale-up nodes. We explore several workloads run on top of the IBM Power8 processor, and provide manual strategies that can leverage performance improvements up to 40% on Spark workloads when using smart processor-pinning and workload collocation strategies.
更多查看译文
关键词
Performance,Modeling,Characterization,Memory,NUMA,Spark,Benchmark
AI 理解论文
溯源树
样例
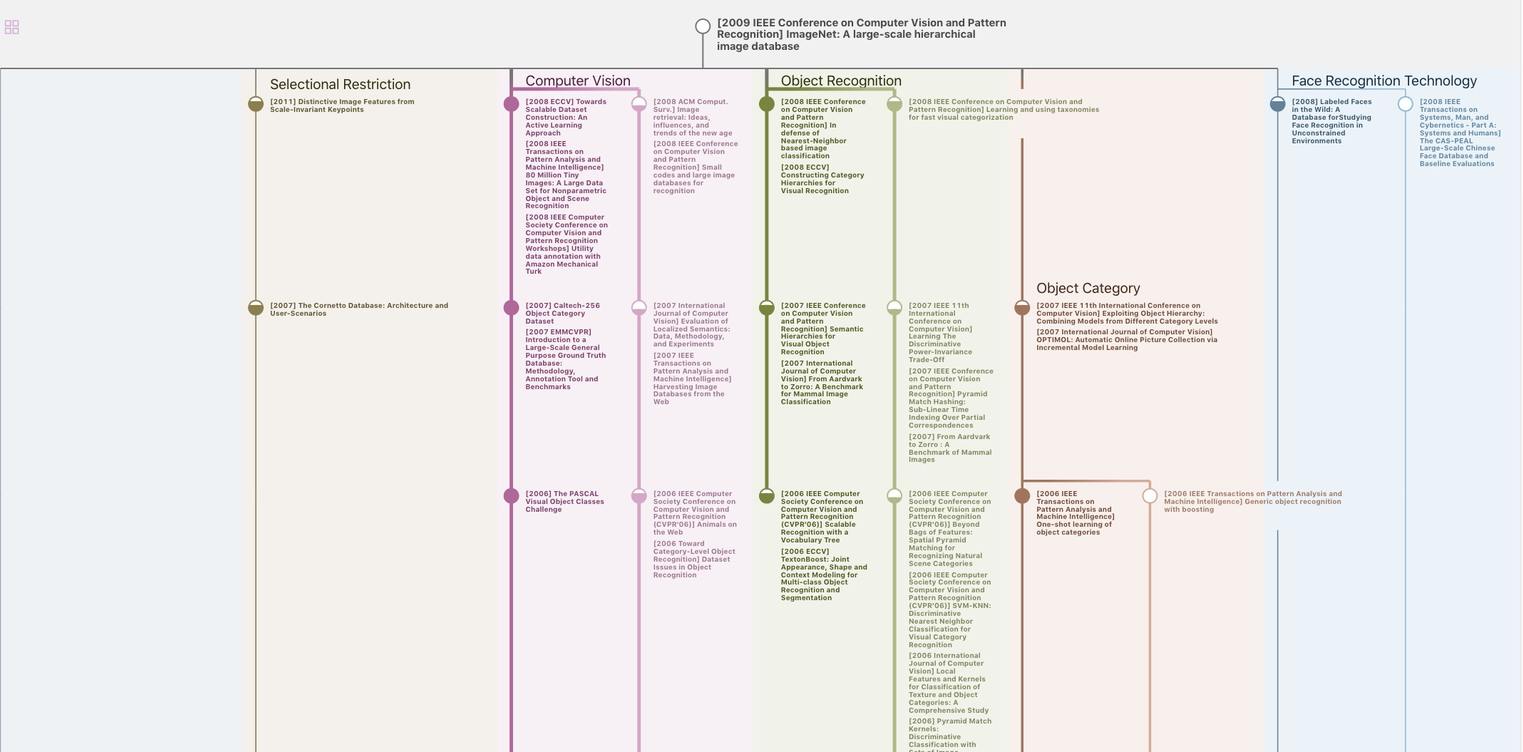
生成溯源树,研究论文发展脉络
Chat Paper
正在生成论文摘要