DeepSol: a deep learning framework for sequence-based protein solubility prediction.
BIOINFORMATICS(2018)
摘要
Motivation: Protein solubility plays a vital role in pharmaceutical research and production yield. For a given protein, the extent of its solubility can represent the quality of its function, and is ultimately defined by its sequence. Thus, it is imperative to develop novel, highly accurate in silico sequence-based protein solubility predictors. In this work we propose, DeepSol, a novel Deep Learning-based protein solubility predictor. The backbone of our framework is a convolutional neural network that exploits k-mer structure and additional sequence and structural features extracted from the protein sequence. Results: DeepSol outperformed all known sequence-based state-of-the-art solubility prediction methods and attained an accuracy of 0.77 and Matthew's correlation coefficient of 0.55. The superior prediction accuracy of DeepSol allows to screen for sequences with enhanced production capacity and can more reliably predict solubility of novel proteins. Availability and implementation: DeepSol's best performing models and results are publicly deposited at https://doi. org/10.5281/zenodo.1162886 (Khurana and Mall, 2018). Contact: skhurana@mit.eduorrmall@hbku.edu.qa Supplementary information: Supplementary data are available at Bioinformatics online.
更多查看译文
AI 理解论文
溯源树
样例
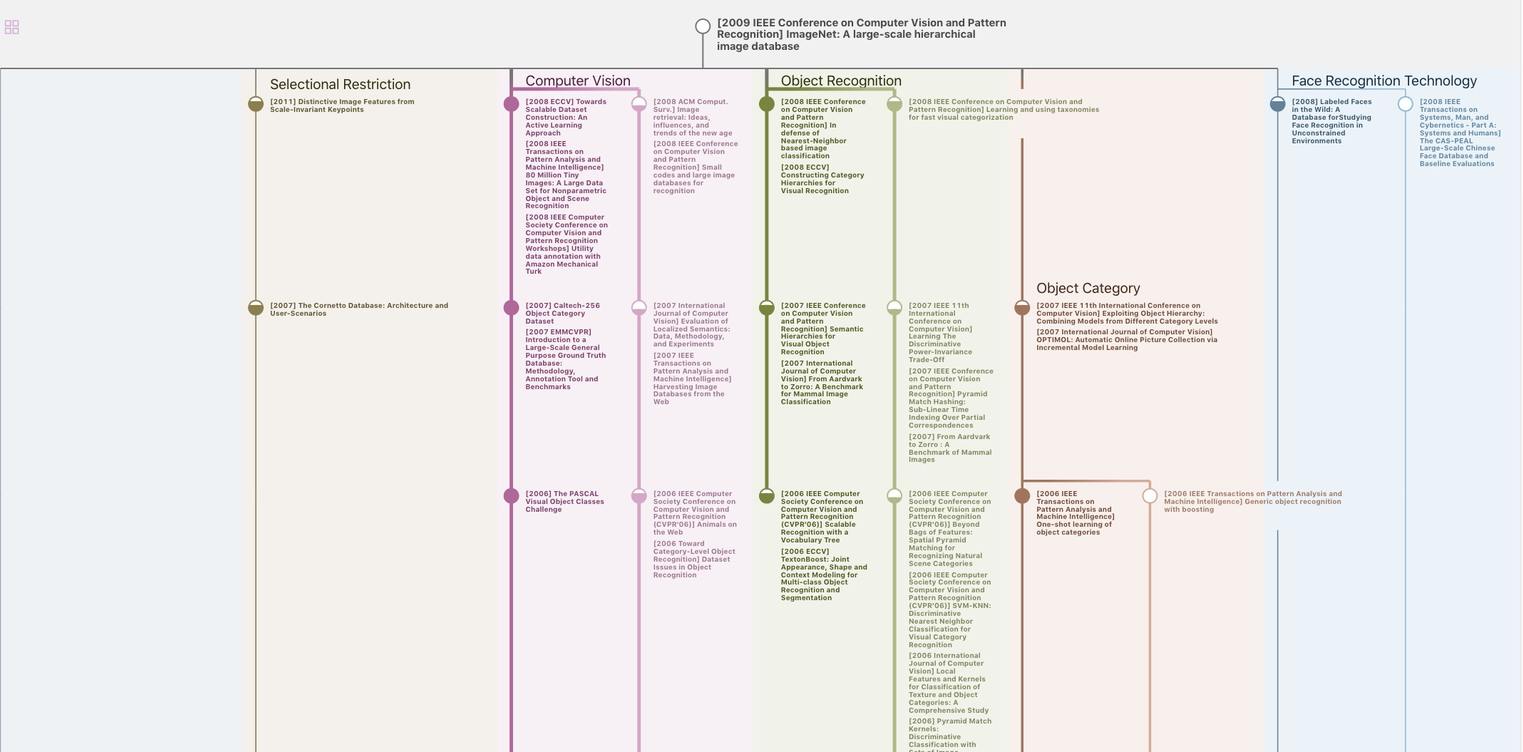
生成溯源树,研究论文发展脉络
Chat Paper
正在生成论文摘要