The universal consistency of extreme learning machine.
Neurocomputing(2018)
摘要
Extreme learning machine (ELM) can be considered as a single-hidden layer feedforward neural network (FNN)-type learning system, whose input weights and hidden layer biases are randomly assigned, while output weights need tuning. In the framework of regression, a fundamental problem of ELM learning is whether the ELM estimator is universally consistent, that is, whether it can approximate arbitrary regression function to any accuracy, provided the number of training samples is sufficiently large. The aim of this paper is two-fold. One is to verify the strongly universal consistency of the ELM estimator, and the other is to present a sufficient and the necessary condition for the activation function, where the corresponding ELM estimator is strongly universally consistent. The obtained results underlie the feasibility of ELM and provide a theoretical guidance of the selection of activation functions in ELM learning.
更多查看译文
关键词
Extreme learning machine,Neural networks,Universal consistency,Activation function
AI 理解论文
溯源树
样例
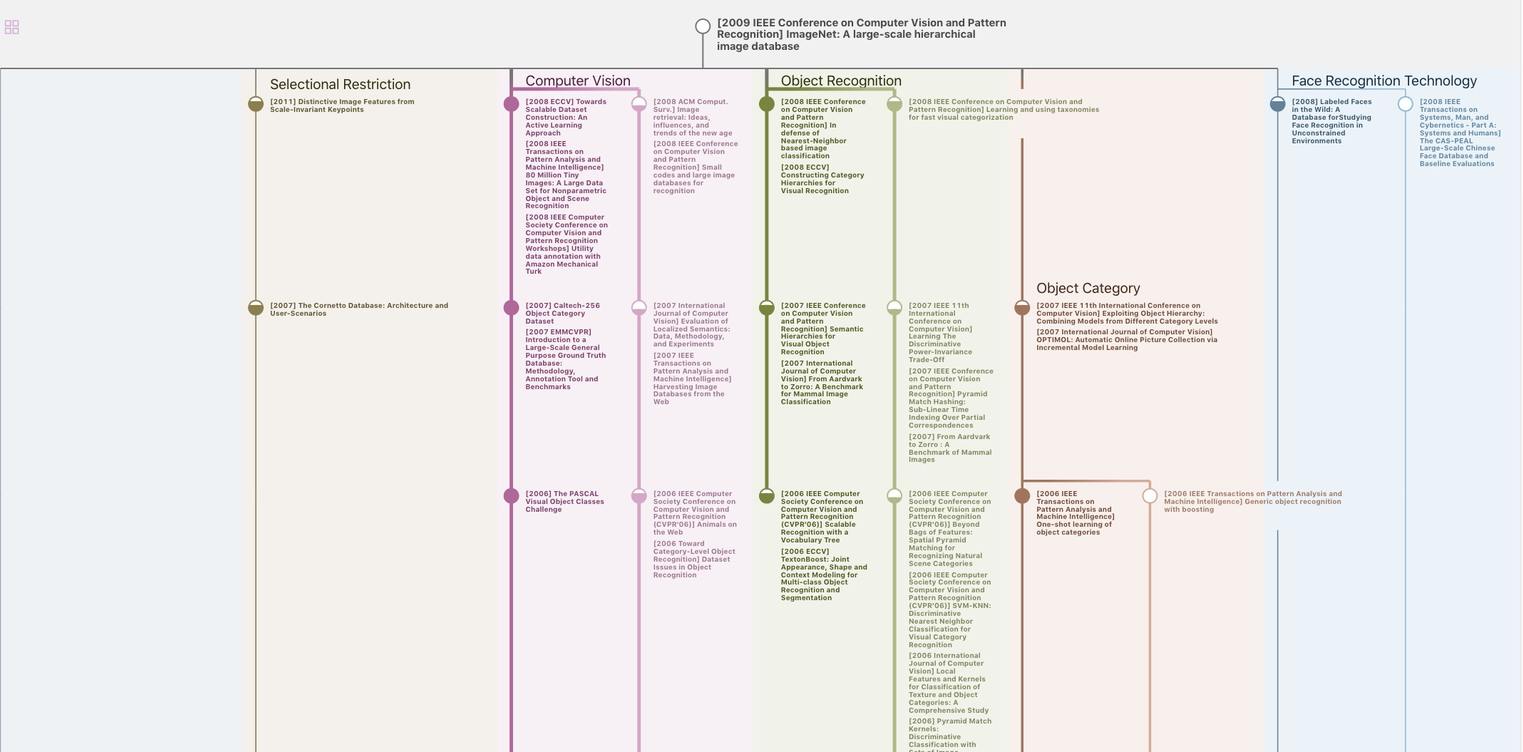
生成溯源树,研究论文发展脉络
Chat Paper
正在生成论文摘要