Ensemble Learning For Overall Power Conversion Efficiency Of The All-Organic Dye-Sensitized Solar Cells
IEEE ACCESS(2018)
摘要
Ensemble learning breaks the bottleneck of weak learners and is usually significantly more accurate than base learners. The overall power conversion efficiency of all-organic dye-sensitized solar cells is difficult to obtain by either calculations or experiments. To achieve high-accuracy models, various ensemble learning methods are investigated. Three types of global ensemble models, including homogeneous and heterogeneous ensembles, are constructed, which outperformed the best single base learner, a support vector machine model (MAE: 0.52; Q(2): 0.76); in particular, a novel local heterogeneous ensemble model (MAE: 0.34 and Q(2): 0.91) achieved high accuracy and generalization. This paper shows ensemble learning model is capable of exploring complicated quantitative structure activity relationship, where the features are distant from targets.
更多查看译文
关键词
Dye-sensitized solar cell,global/local learning ensemble,heterogeneous/homogeneous learning ensemble,machine learning,power conversion efficiency,quantitative structure activity relationship
AI 理解论文
溯源树
样例
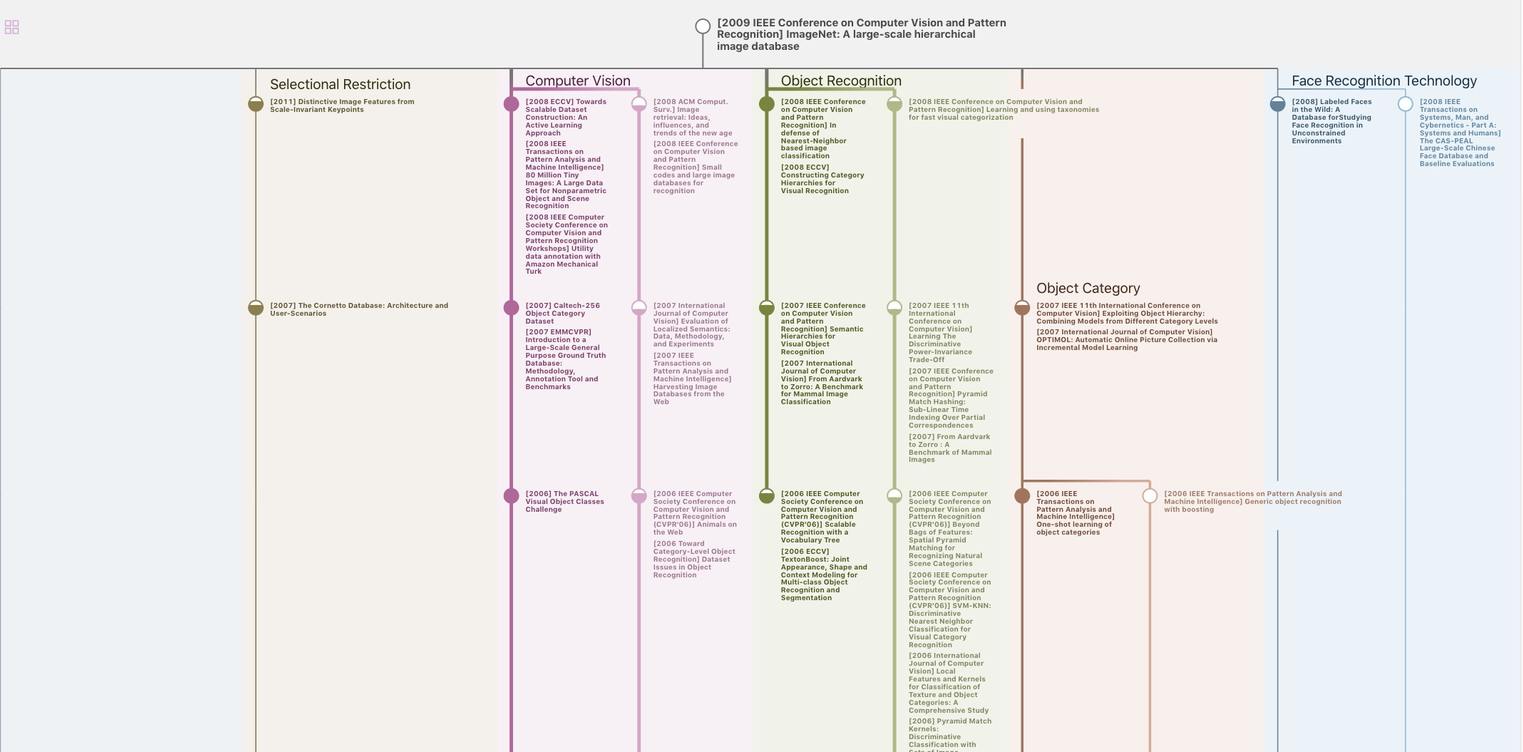
生成溯源树,研究论文发展脉络
Chat Paper
正在生成论文摘要