Topology2Vec: Topology Representation Learning For Data Center Networking.
IEEE ACCESS(2018)
摘要
The use of machine learning (ML) algorithms to conduct prediction or analysis tasks in a data center networking (DCN) environment is gaining increasing attention today. Recent research in traffic prediction, abnormal traffic monitoring, and routing selection has led to significant progress by making full use of historical data and improved ML models. However, such approaches face challenges when dealing with graphical data. These approaches have limited capabilities to explore the information that is hiding in the network's topological structure. To solve these challenges, we study the problem of representation learning in DCN topologies. To serve as a bridge, we proposed a novel method, "Topology2Vec," to learn the network topology and represent the nodes using low-dimensional vectors, which is useful in many topology-related tasks. Both network structure and performance are considered in our method to ensure that the representation can adapt to different requirements. To evaluate the effectiveness, we demonstrate this method in a controller placement problem as a typical use case using topological data from real-world data centers. The experiments show that the use of "Topology2Vec" as a premise has produced better results in terms of network latency.
更多查看译文
关键词
Data center networking,network representation learning,controller placement
AI 理解论文
溯源树
样例
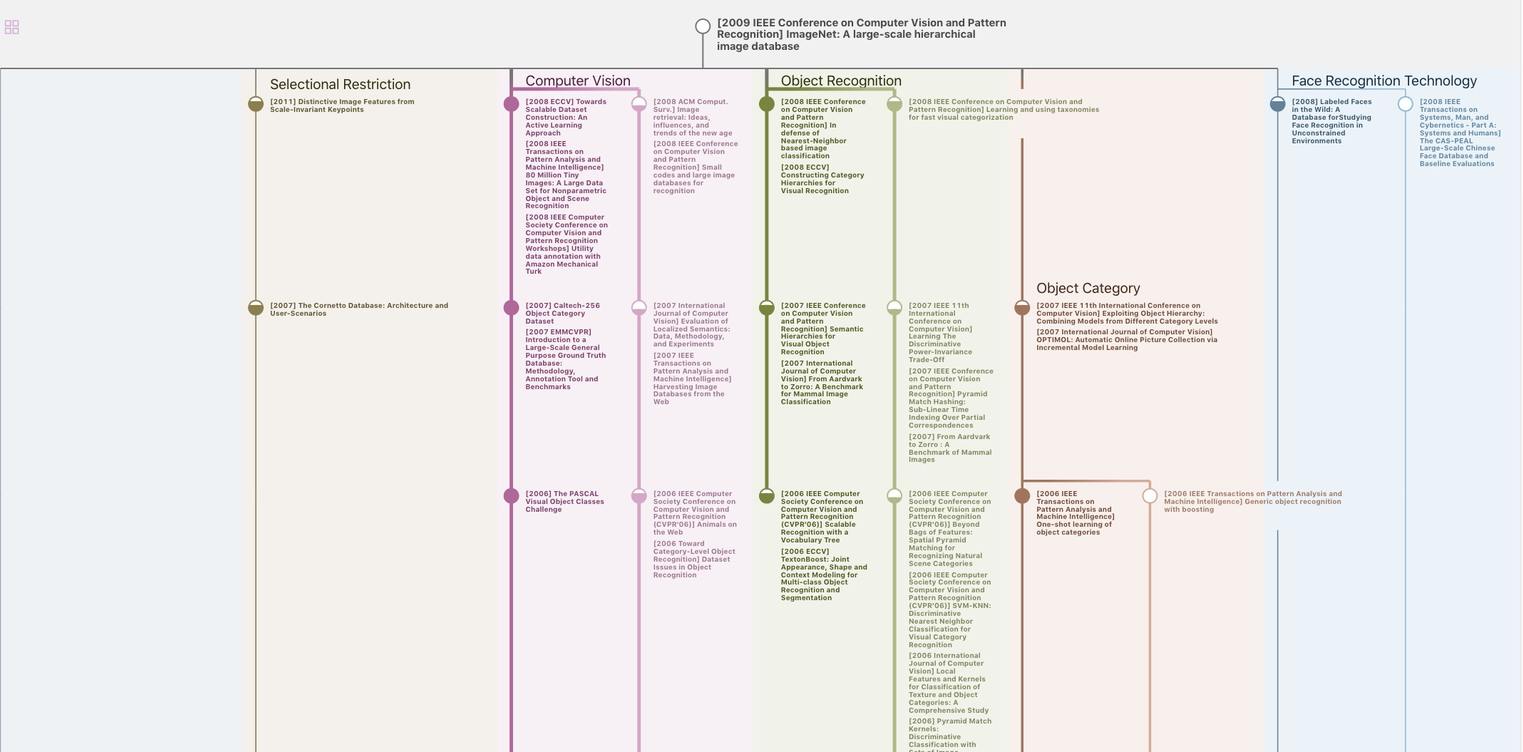
生成溯源树,研究论文发展脉络
Chat Paper
正在生成论文摘要