Sparse Channel Estimation for Massive MIMO-OFDM Systems Over Time-Varying Channels.
IEEE ACCESS(2018)
摘要
For downlink orthogonal frequency division multiplexing-based massive multiple-inputmultiple-output transmission over the time-varying channel, channel estimation is very challenging due to numerous channel coefficients to be estimated. To track this problem, this paper proposes a novel sparse channel estimation scheme, exploiting the sparsity in the delay domain and the high correlation in the spatial domain. By utilizing the basis expansion model (BEM) to model the time variation and the generalized-spatial BEM to model the spatial correlation, we are able to significantly reduce the number of the coefficients to be estimated. Then, the channel estimation is formulated into a compressive sensing problem with a quasiblock-sparse matrix to be recovered. Motivated by the quasi-block sparsity, a novel quasi-block simultaneous orthogonal matching pursuit (QBSO) algorithm is developed to recover the channel, which seeks nonzero channel tap positions at the first stage and calculates the channel coefficients at the second stage. Moreover, an adaptive-QBSO algorithm is further designed to improve the recovery accuracy, where the measurement matrix is adaptively designed based on the estimated virtual angle of departure, and thus, the sparsity representation can be strengthened. We also discuss the design of pilot pattern including pilot values and positions. Simulation results are provided to validate the effectiveness of our proposed channel estimation scheme.
更多查看译文
关键词
Time-varying channel estimation,massive MIMO,compressive sensing,generalized-spatial basis expansion model,pilot design
AI 理解论文
溯源树
样例
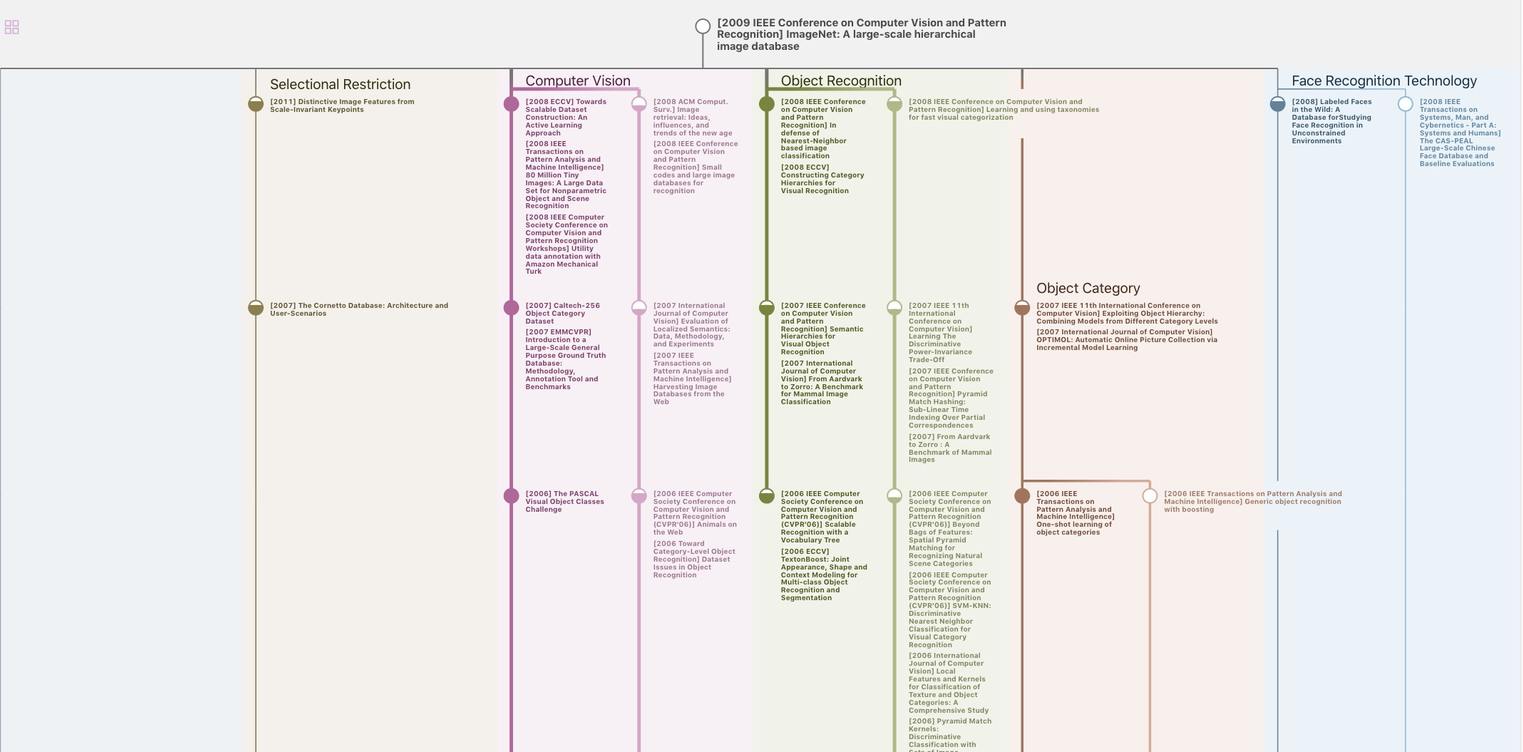
生成溯源树,研究论文发展脉络
Chat Paper
正在生成论文摘要