Joint Linear Regression and Nonnegative Matrix Factorization Based on Self-Organized Graph for Image Clustering and Classification.
IEEE ACCESS(2018)
摘要
Nonnegative matrix factorization (NMF) technique has been developed successfully to represent the intuitively meaningful feature of data. A suitable representation can faithfully preserve the intrinsic structure of data. Due to the fact that it introduces the label information, semi-supervised NMF has been demonstrated more advantageous in image representation than original NMF. However, previous semi-supervised NMF variants construct a label indicator matrix only for tagging the labeled data and not being optimized together with the matrix factorization. It is short of label propagation and fails to work for predicting the attribution of data. Moreover, the transductive semi-supervised NMF variants cannot dispose the prediction of unseen data, restricting the application of NMF. In this paper, a joint optimization framework of linear regression and NMF (LR-NMF) based on the self-organized graph is proposed for a completed task which simultaneously takes into account image representation and attribution prediction. By minimizing the proposed objective, three interactive threads are running: decomposing the data into nonnegative basis matrix and the corresponding representation, linear regression using the nonnegative representation, and label propagation based on the self-organized graph which is defined in the feature space. The products of LR-NMF can be viewed as extracting nonnegative feature for clustering, meanwhile, they can be used to solve the out-of-sample problem for classification. Extensive clustering and classification experiments on the digit, face, and object challenging data sets are presented to show the efficacy of the proposed LR-NMF algorithm.
更多查看译文
关键词
Nonnegative matrix factorization,linear regression,self-organized graph,semi-supervised clustering and classification
AI 理解论文
溯源树
样例
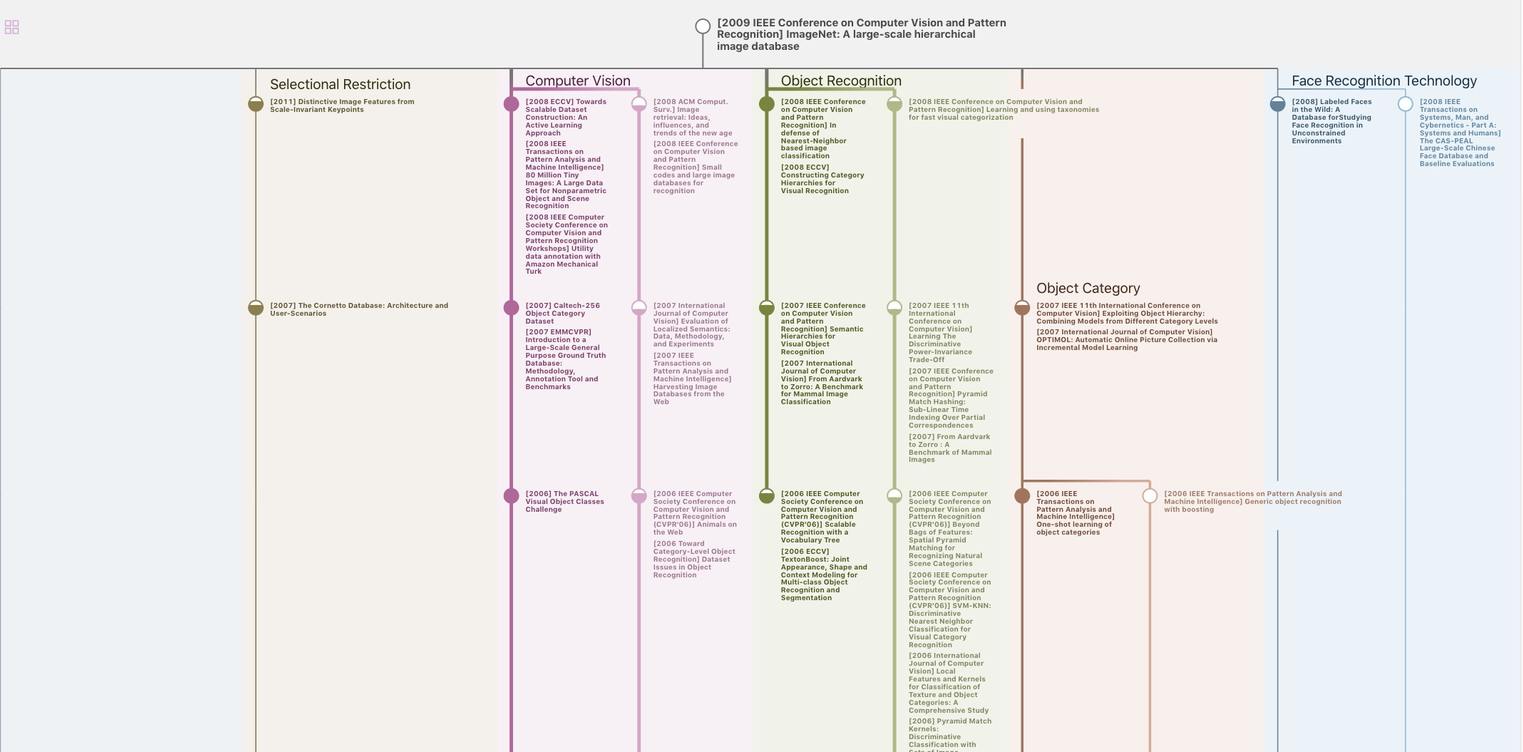
生成溯源树,研究论文发展脉络
Chat Paper
正在生成论文摘要