Scalable Importance Sampling Estimation of Gaussian Mixture Posteriors in Bayesian Networks
International journal of approximate reasoning(2018)
摘要
In this paper we propose a scalable importance sampling algorithm for computing Gaussian mixture posteriors in conditional linear Gaussian Bayesian networks. Our contribution is based on using a stochastic gradient ascent procedure taking as input a stream of importance sampling weights, so that a mixture of Gaussians is dynamically updated with no need to store the full sample. The algorithm has been designed following a Map/Reduce approach and is therefore scalable with respect to computing resources. The implementation of the proposed algorithm is available as part of the AMIDST open-source toolbox for scalable probabilistic machine learning (http://www.amidsttoolbox.com).
更多查看译文
关键词
Importance sampling,Bayesian networks,Conditional linear Gaussian models,Scalable inference,Gaussian mixtures
AI 理解论文
溯源树
样例
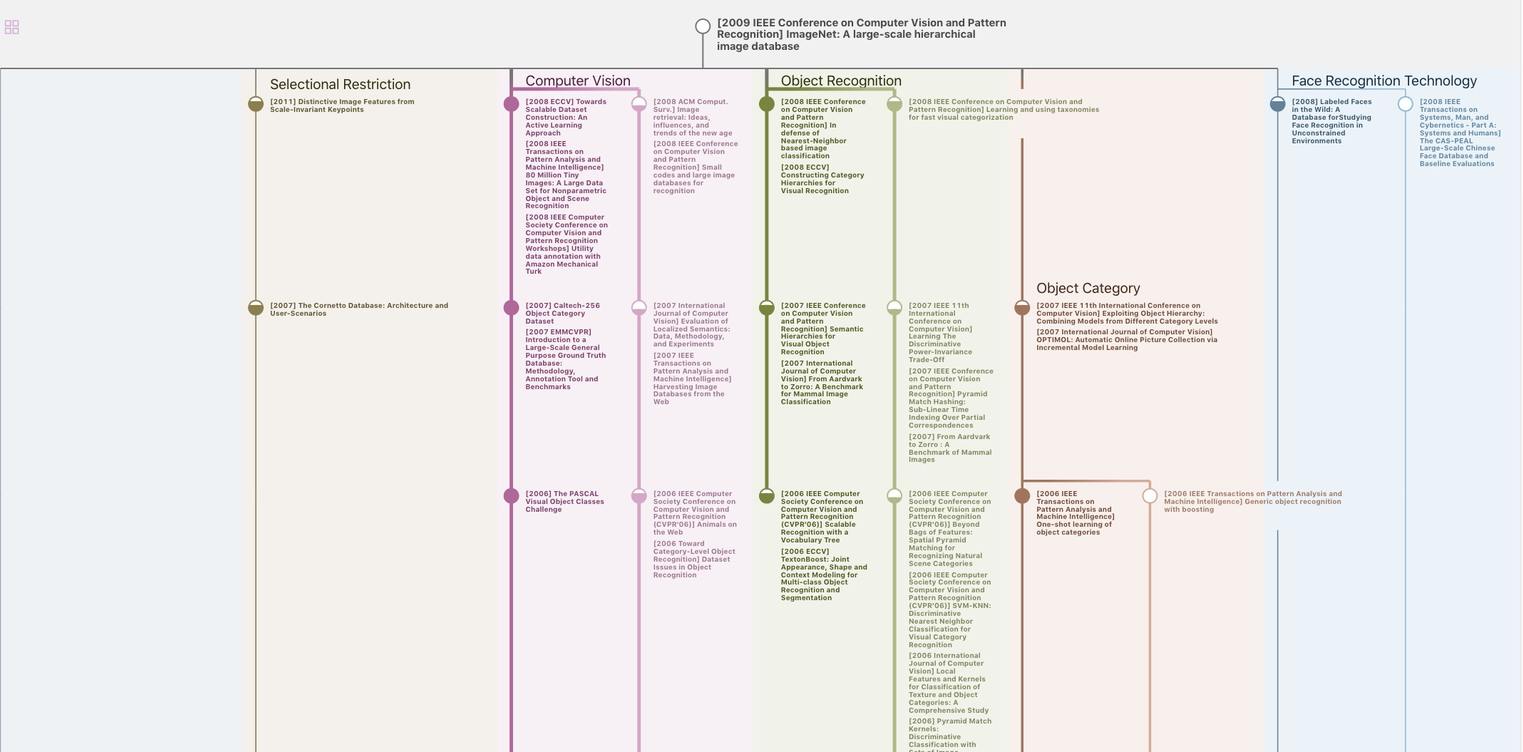
生成溯源树,研究论文发展脉络
Chat Paper
正在生成论文摘要