DeepCotton: in-field cotton segmentation using deep fully convolutional network.
JOURNAL OF ELECTRONIC IMAGING(2017)
摘要
Automatic ground-based in-field cotton (IFC) segmentation is a challenging task in precision agriculture, which has not been well addressed. Nearly all the existing methods rely on hand-crafted features. Their limited discriminative power results in unsatisfactory performance. To address this, a coarse-to-fine cotton segmentation method termed "DeepCotton" is proposed. It contains two modules, fully convolutional network (FCN) stream and interference region removal stream. First, FCN is employed to predict initially coarse map in an endto-end manner. The convolutional networks involved in FCN guarantee powerful feature description capability, simultaneously, the regression analysis ability of neural network assures segmentation accuracy. To our knowledge, we are the first to introduce deep learning to IFC segmentation. Second, our proposed "UP" algorithm composed of unary brightness transformation and pairwise region comparison is used for obtaining interference map, which is executed to refine the coarse map. The experiments on constructed IFC dataset demonstrate that our method outperforms other state-of-the-art approaches, either in different common scenarios or single/ multiple plants. More remarkable, the "UP" algorithm greatly improves the property of the coarse result, with the average amplifications of 2.6%, 2.4% on accuracy and 8.1%, 5.5% on intersection over union for common scenarios and multiple plants, separately. (C) 2017 SPIE and IS&T
更多查看译文
关键词
cotton segmentation,computer vision,fully convolutional network,interference region removal
AI 理解论文
溯源树
样例
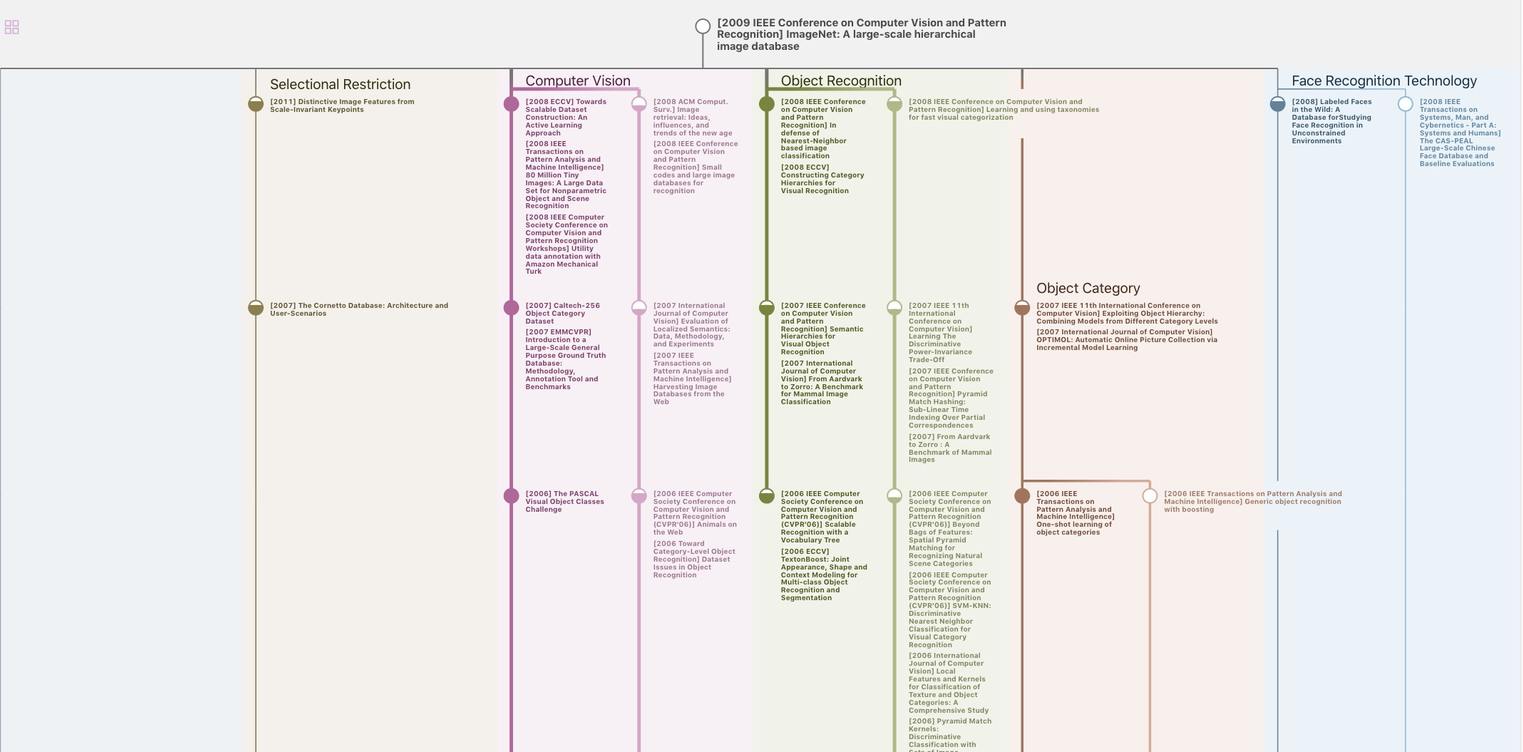
生成溯源树,研究论文发展脉络
Chat Paper
正在生成论文摘要