Feature learning based on visual similarity triplets in medical image analysis: A case study of emphysema in chest CT scans
INTRAVASCULAR IMAGING AND COMPUTER ASSISTED STENTING AND LARGE-SCALE ANNOTATION OF BIOMEDICAL DATA AND EXPERT LABEL SYNTHESIS(2018)
摘要
Supervised feature learning using convolutional neural networks (CNNs) can provide concise and disease relevant representations of medical images. However, training CNNs requires annotated image data. Annotating medical images can be a time-consuming task and even expert annotations are subject to substantial inter- and intra-rater variability. Assessing visual similarity of images instead of indicating specific pathologies or estimating disease severity could allow non-experts to participate, help uncover new patterns, and possibly reduce rater variability. We consider the task of assessing emphysema extent in chest CT scans. We derive visual similarity triplets from visually assessed emphysema extent and learn a low dimensional embedding using CNNs. We evaluate the networks on 973 images, and show that the CNNs can learn disease relevant feature representations from derived similarity triplets. To our knowledge this is the first medical image application where similarity triplets has been used to learn a feature representation that can be used for embedding unseen test images
更多查看译文
关键词
visual similarity triplets,emphysema,medical image
AI 理解论文
溯源树
样例
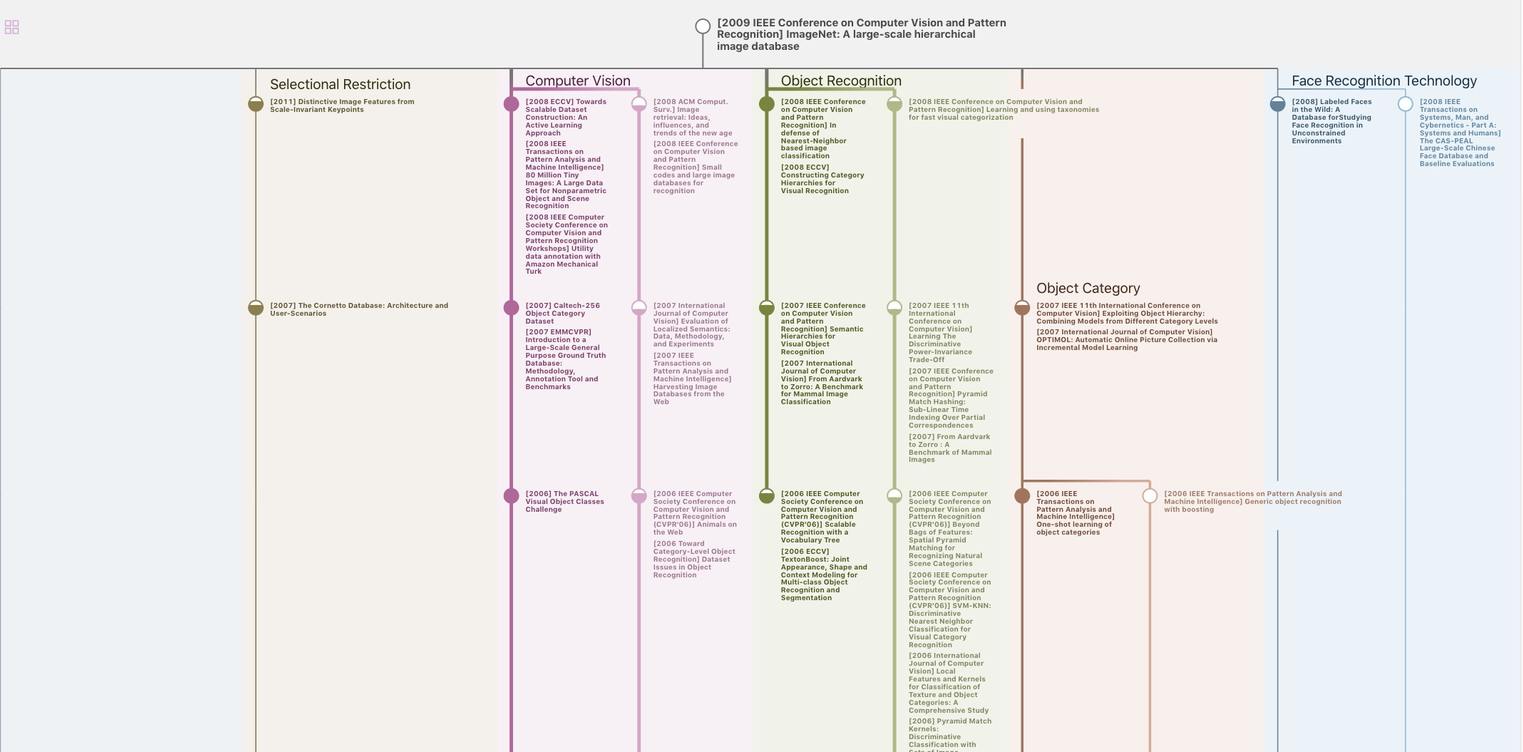
生成溯源树,研究论文发展脉络
Chat Paper
正在生成论文摘要