CBAM: Convolutional Block Attention Module
Computer Vision – ECCV 2018: 15th European Conference, Munich, Germany, September 8–14, 2018, Proceedings, Part VII(2018)
摘要
We propose Convolutional Block Attention Module (CBAM), a simple yet effective attention module for feed-forward convolutional neural networks. Given an intermediate feature map, our module sequentially infers attention maps along two separate dimensions, channel and spatial, then the attention maps are multiplied to the input feature map for adaptive feature refinement. Because CBAM is a lightweight and general module, it can be integrated into any CNN architectures seamlessly with negligible overheads and is end-to-end trainable along with base CNNs. We validate our CBAM through extensive experiments on ImageNet-1K, MS~COCO detection, and VOC~2007 detection datasets. Our experiments show consistent improvements in classification and detection performances with various models, demonstrating the wide applicability of CBAM. The code and models will be publicly available.
更多查看译文
关键词
Object recognition,Attention mechanism,Gated convolution
AI 理解论文
溯源树
样例
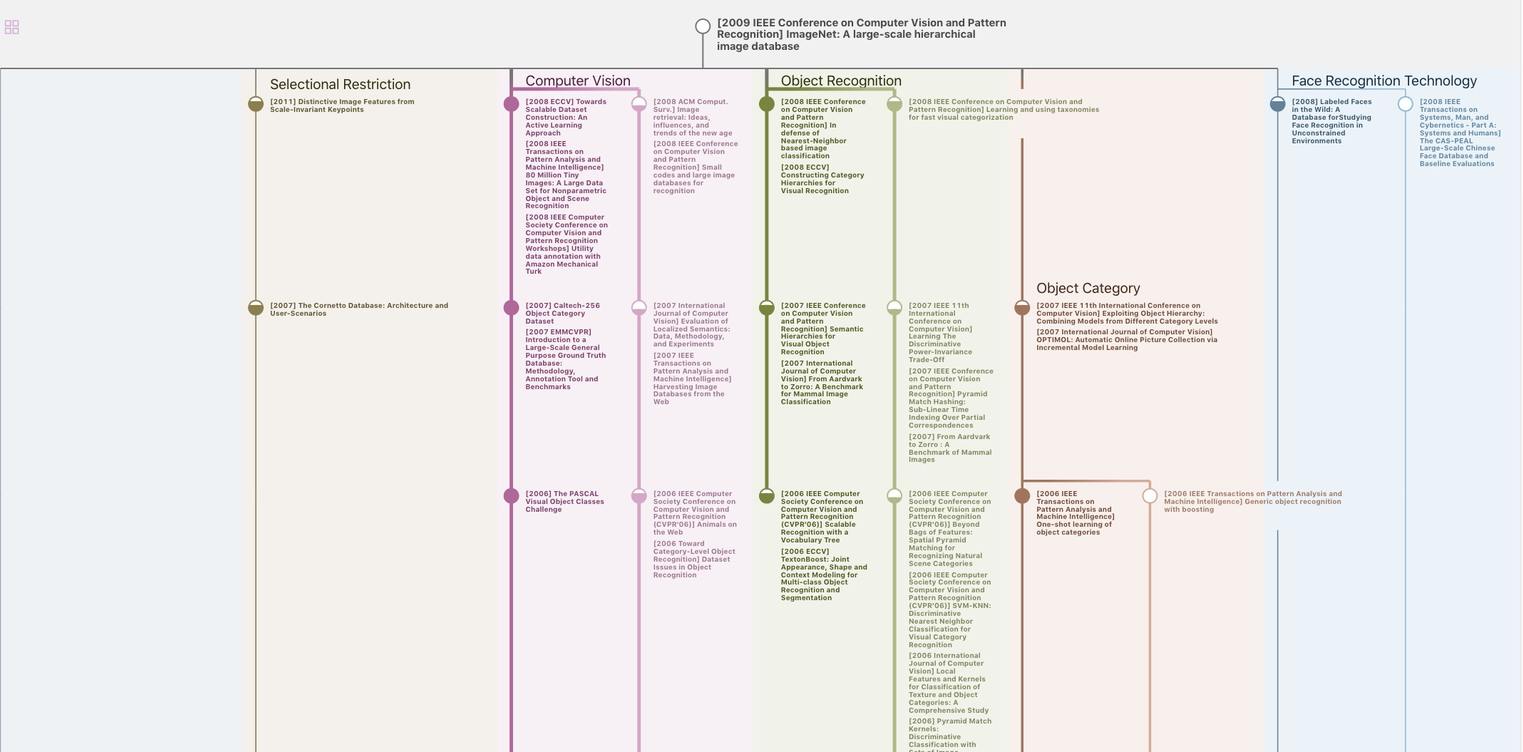
生成溯源树,研究论文发展脉络
Chat Paper
正在生成论文摘要