Sequential Test For The Lowest Mean: From Thompson To Murphy Sampling
ADVANCES IN NEURAL INFORMATION PROCESSING SYSTEMS 31 (NIPS 2018)(2018)
摘要
Learning the minimum/maximum mean among a finite set of distributions is a fundamental sub-task in planning, game tree search and reinforcement learning. We formalize this learning task as the problem of sequentially testing how the minimum mean among a finite set of distributions compares to a given threshold. We develop refined non-asymptotic lower bounds, which show that optimality mandates very different sampling behavior for a low vs high true minimum. We show that Thompson Sampling and the intuitive Lower Confidence Bounds policy each nail only one of these cases. We develop a novel approach that we call Murphy Sampling. Even though it entertains exclusively low true minima, we prove that MS is optimal for both possibilities. We then design advanced self-normalized deviation inequalities, fueling more aggressive stopping rules. We complement our theoretical guarantees by experiments showing that MS works best in practice.
更多查看译文
关键词
reinforcement learning,lower bounds,thompson sampling,finite set,game tree search,ms works
AI 理解论文
溯源树
样例
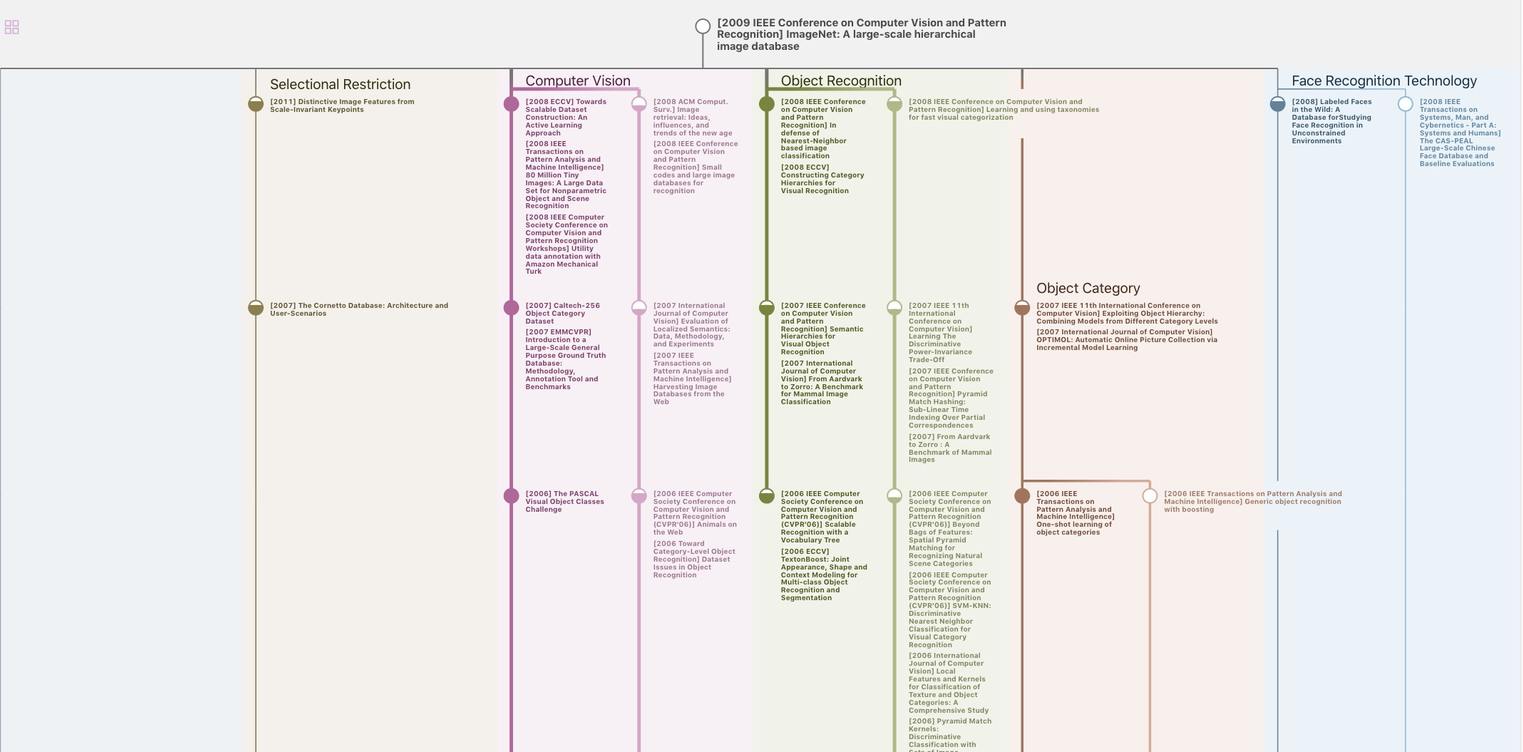
生成溯源树,研究论文发展脉络
Chat Paper
正在生成论文摘要