Machine Learning Based Uplink Transmission Power Prediction for LTE and Upcoming 5G Networks using Passive Downlink Indicators
2018 IEEE 88th Vehicular Technology Conference (VTC-Fall)(2020)
摘要
Energy-aware system design is an important optimization task for static and mobile Internet of Things (IoT)-based sensor nodes, especially for highly resource-constrained vehicles such as mobile robotic systems. For 4G/5G-based cellular communication systems, the effective transmission power of uplink data transmissions is of crucial importance for the overall system power consumption. Unfortunately, this information is usually hidden within off-the-shelf modems and mobile handsets and can therefore not be exploited for enabling green communication. Moreover, the dynamic transmission power control behavior of the mobile device is not even explicitly modeled in most of the established simulation frameworks. In this paper, we present a novel machine learning-based approach for forecasting the resulting uplink transmission power used for data transmissions based on the available passive network quality indicators and application-level information. The model is derived from comprehensive field measurements of drive tests performed in a public cellular network and can be parameterized for integrating all measurements a given target platform is able to provide into the prediction process. In a comparison of three different machine learning methods, Random-Forest models thoroughly performed best with a mean average error of 3.166 dB. As the absolute sum of errors converges towards zero and falls below 1 dB after 28 predictions in average, the approach is well-suited for long-term power estimations.
更多查看译文
关键词
application-level information,comprehensive field measurements,public cellular network,prediction process,Random-Forest models,long-term power estimations,uplink transmission power prediction,passive downlink indicators,energy-aware system design,static Internet,mobile Internet,highly resource-constrained vehicles,mobile robotic systems,4G/5G-based cellular communication systems,effective transmission power,uplink data transmissions,system power consumption,off-the-shelf modems,mobile handsets,green communication,dynamic transmission power control behavior,mobile device,machine learning methods,uplink transmission power,passive network quality indicators,mobile Internet of Things-based sensor nodes
AI 理解论文
溯源树
样例
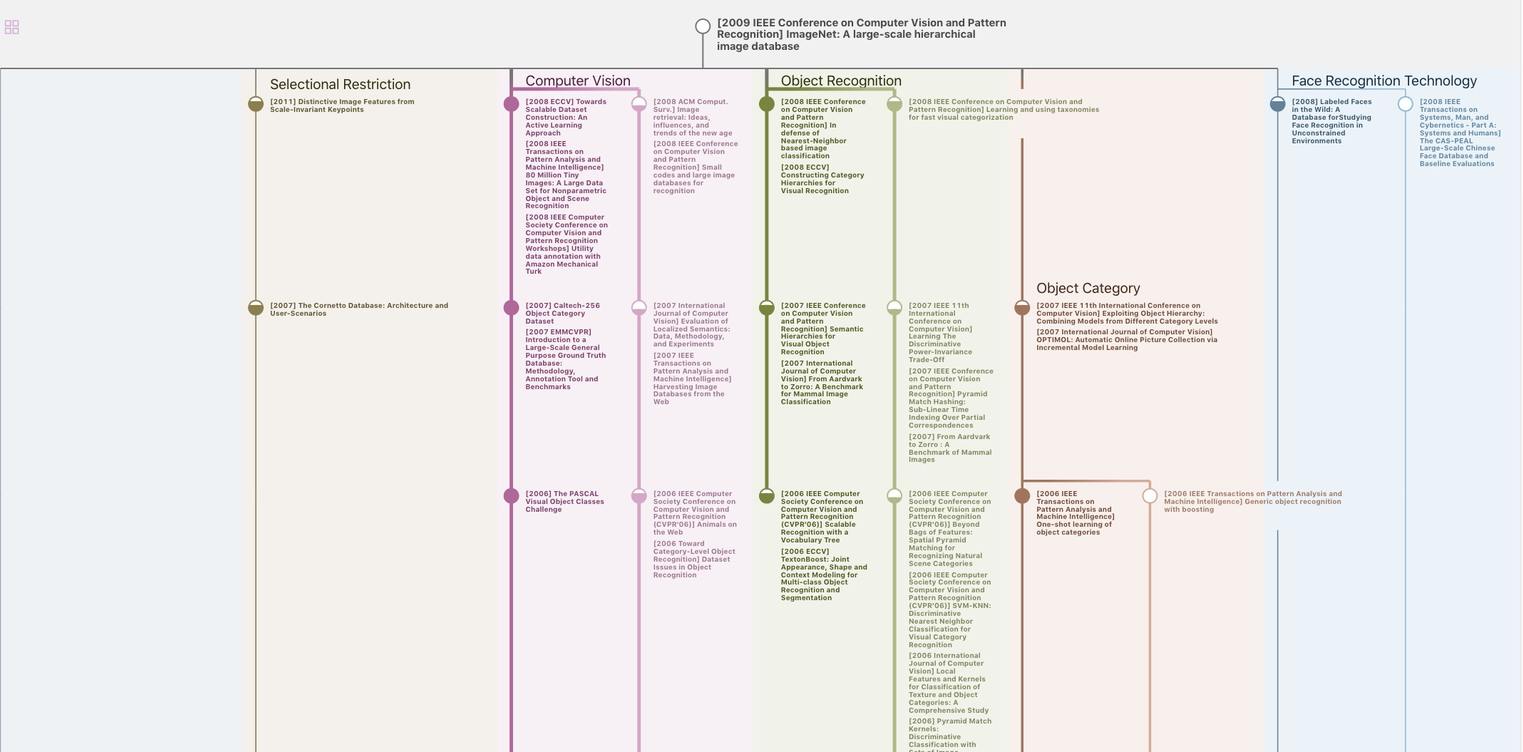
生成溯源树,研究论文发展脉络
Chat Paper
正在生成论文摘要