Sequential Attend, Infer, Repeat: Generative Modelling of Moving Objects.
ADVANCES IN NEURAL INFORMATION PROCESSING SYSTEMS 31 (NIPS 2018)(2018)
摘要
We present Sequential Attend, Infer, Repeat (SQAIR), an interpretable deep generative model for videos of moving objects. It can reliably discover and track objects throughout the sequence of frames, and can also generate future frames conditioning on the current frame, thereby simulating expected motion of objects. This is achieved by explicitly encoding object presence, locations and appearances in the latent variables of the model. SQAIR retains all strengths of its predecessor, Attend, Infer, Repeat (AIR, Eslami et al., 2016), including learning in an unsupervised manner, and addresses its shortcomings. We use a moving multi MNIST dataset to show limitations of AIR in detecting overlapping or partially occluded objects, and show how SQAIR overcomes them by leveraging temporal consistency of objects. Finally, we also apply SQAIR to real world pedestrian CCTV data, where it learns to reliably detect, track and generate walking pedestrians with no supervision.
更多查看译文
关键词
latent variables,moving objects,unsupervised learning,model structure
AI 理解论文
溯源树
样例
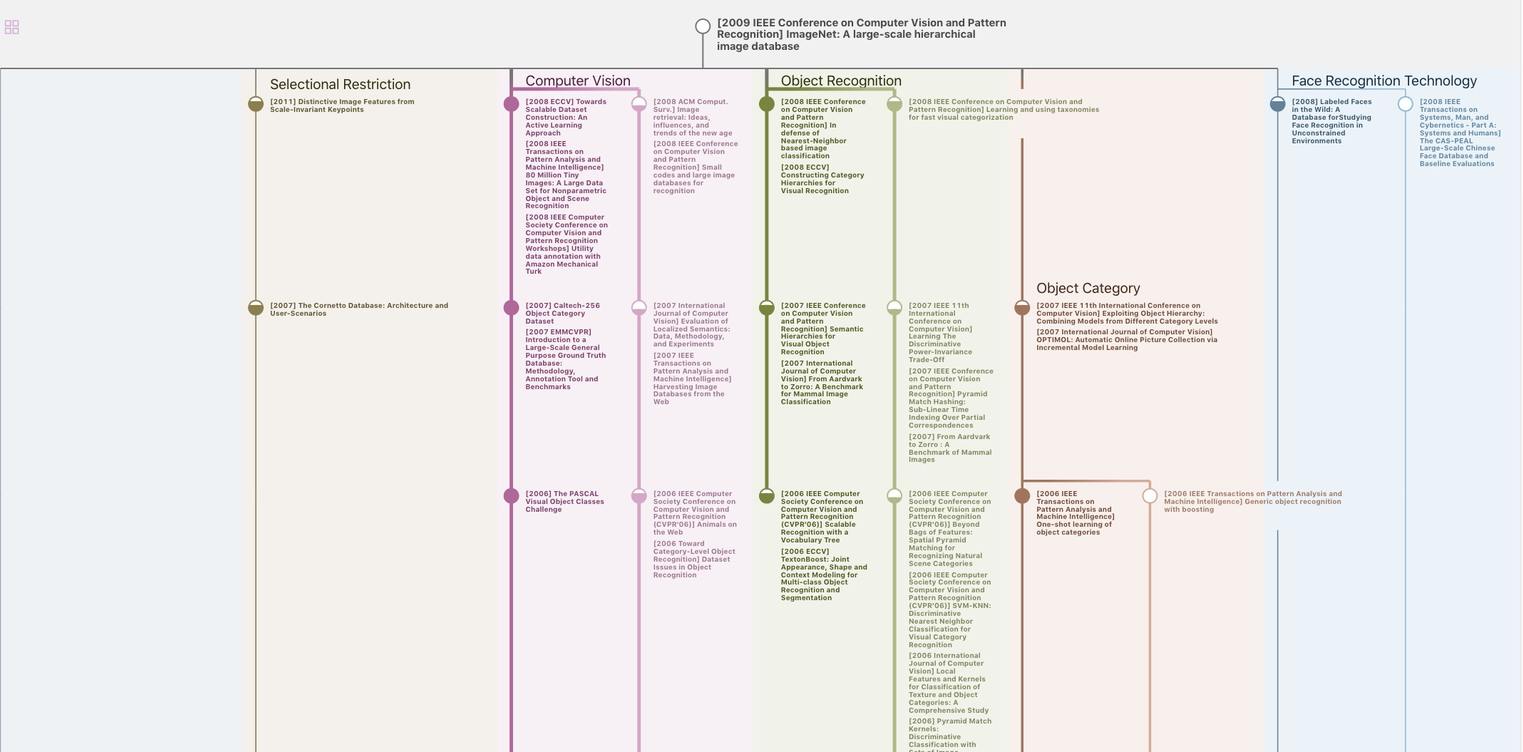
生成溯源树,研究论文发展脉络
Chat Paper
正在生成论文摘要