Learning Answer Embeddings for Visual Question Answering
2018 IEEE/CVF Conference on Computer Vision and Pattern Recognition(2018)
摘要
We propose a novel probabilistic model for visual question answering (Visual QA). The key idea is to infer two sets of embeddings: one for the image and the question jointly and the other for the answers. The learning objective is to learn the best parameterization of those embeddings such that the correct answer has higher likelihood among all possible answers. In contrast to several existing approaches of treating Visual QA as multi-way classification, the proposed approach takes the semantic relationships (as characterized by the embeddings) among answers into consideration, instead of viewing them as independent ordinal numbers. Thus, the learned embedded function can be used to embed unseen answers (in the training dataset). These properties make the approach particularly appealing for transfer learning for open-ended Visual QA, where the source dataset on which the model is learned has limited overlapping with the target dataset in the space of answers. We have also developed large-scale optimization techniques for applying the model to datasets with a large number of answers, where the challenge is to properly normalize the proposed probabilistic models. We validate our approach on several Visual QA datasets and investigate its utility for transferring models across datasets. The empirical results have shown that the approach performs well not only on in-domain learning but also on transfer learning.
更多查看译文
关键词
answer embeddings,visual question answering,probabilistic model,learning objective,possible answers,learned embedded function,unseen answers,transfer learning,in-domain learning,visual QA datasets,open-ended visual QA
AI 理解论文
溯源树
样例
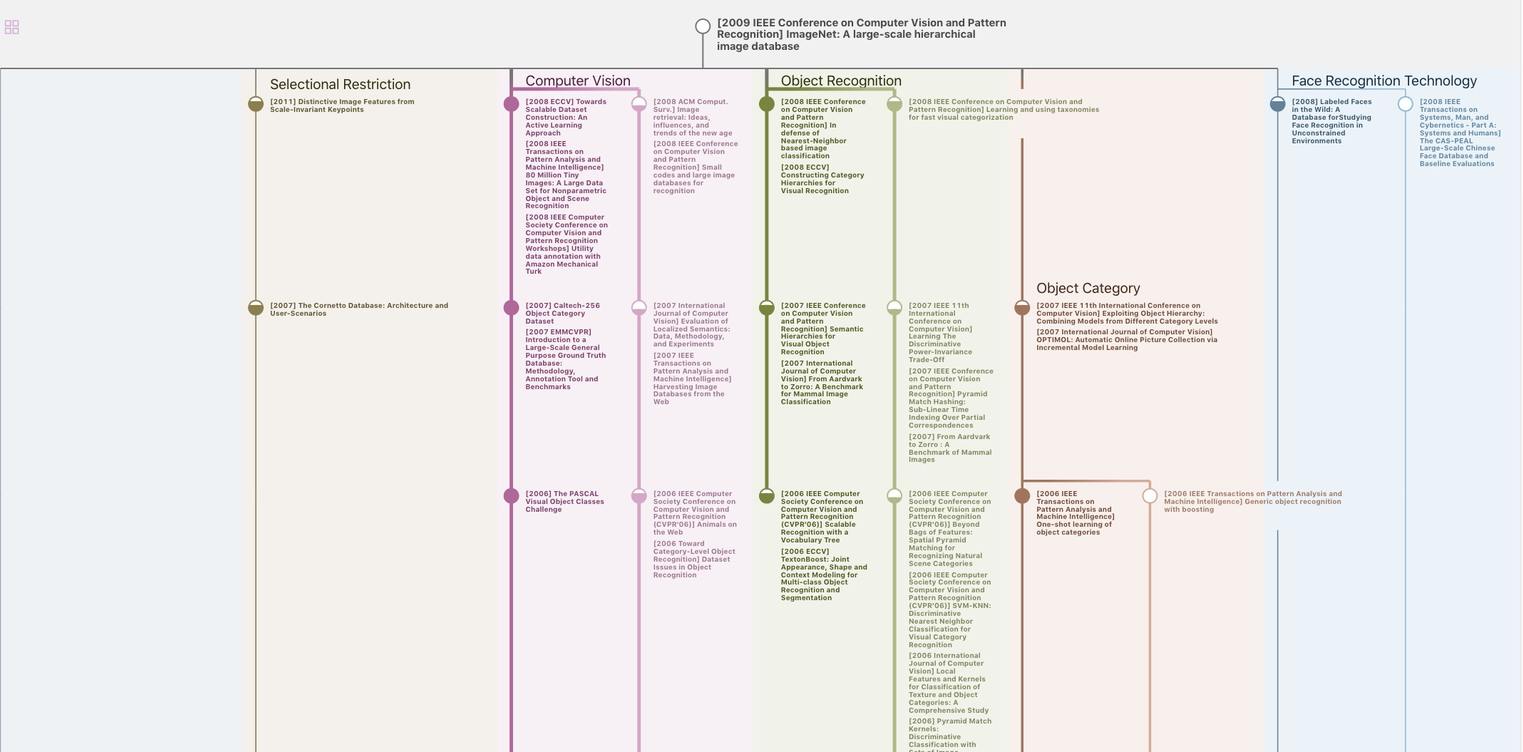
生成溯源树,研究论文发展脉络
Chat Paper
正在生成论文摘要