Procedural Level Generation Improves Generality of Deep Reinforcement Learning.
arXiv: Learning(2018)
摘要
Over the last few years, deep reinforcement learning (RL) has shown impressive results in a variety of domains, learning directly from high-dimensional sensory streams. However, when networks are trained in a fixed environment, such as a single level in a video game, it will usually overfit and fail to generalize to new levels. When RL agents overfit, even slight modifications to the environment can result in poor agent performance. In this paper, we present an approach to prevent overfitting by generating more general agent controllers, through training the agent on a completely new and procedurally generated level each episode. The level generator generate levels whose difficulty slowly increases in response to the observed performance of the agent. Our results show that this approach can learn policies that generalize better to other procedurally generated levels, compared to policies trained on fixed levels.
更多查看译文
AI 理解论文
溯源树
样例
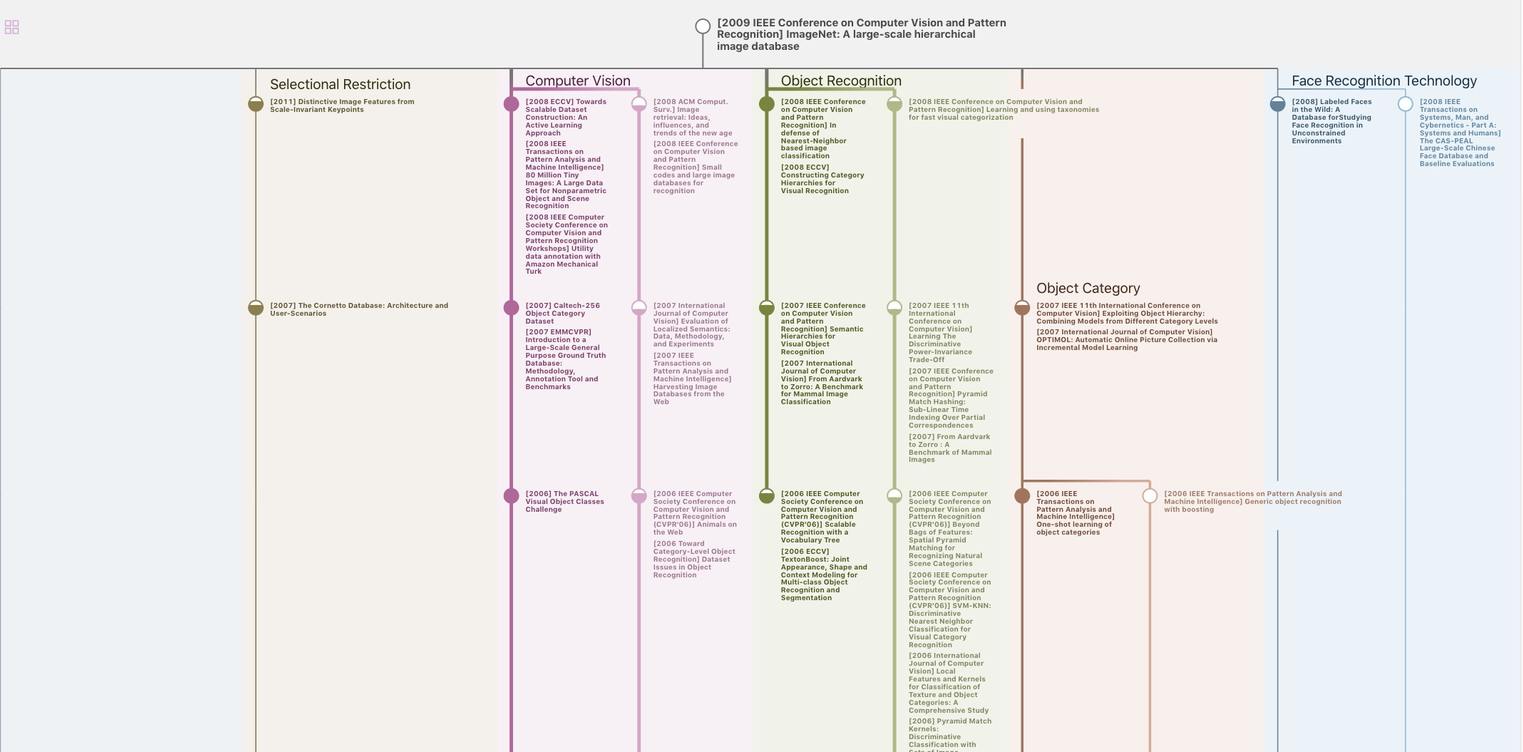
生成溯源树,研究论文发展脉络
Chat Paper
正在生成论文摘要