Model-based Hand Pose Estimation for Generalized Hand Shape with Appearance Normalization
arXiv (Cornell University)(2018)
摘要
Since the emergence of large annotated datasets, state-of-the-art hand pose estimation methods have been mostly based on discriminative learning. Recently, a hybrid approach has embedded a kinematic layer into the deep learning structure in such a way that the pose estimates obey the physical constraints of human hand kinematics. However, the existing approach relies on a single person's hand shape parameters, which are fixed constants. Therefore, the existing hybrid method has problems to generalize to new, unseen hands. In this work, we extend the kinematic layer to make the hand shape parameters learnable. In this way, the learnt network can generalize towards arbitrary hand shapes. Furthermore, inspired by the idea of Spatial Transformer Networks, we apply a cascade of appearance normalization networks to decrease the variance in the input data. The input images are shifted, rotated, and globally scaled to a similar appearance. The effectiveness and limitations of our proposed approach are extensively evaluated on the Hands 2017 challenge dataset and the NYU dataset.
更多查看译文
关键词
hand pose estimation,generalized hand shape,appearance normalization,model-based
AI 理解论文
溯源树
样例
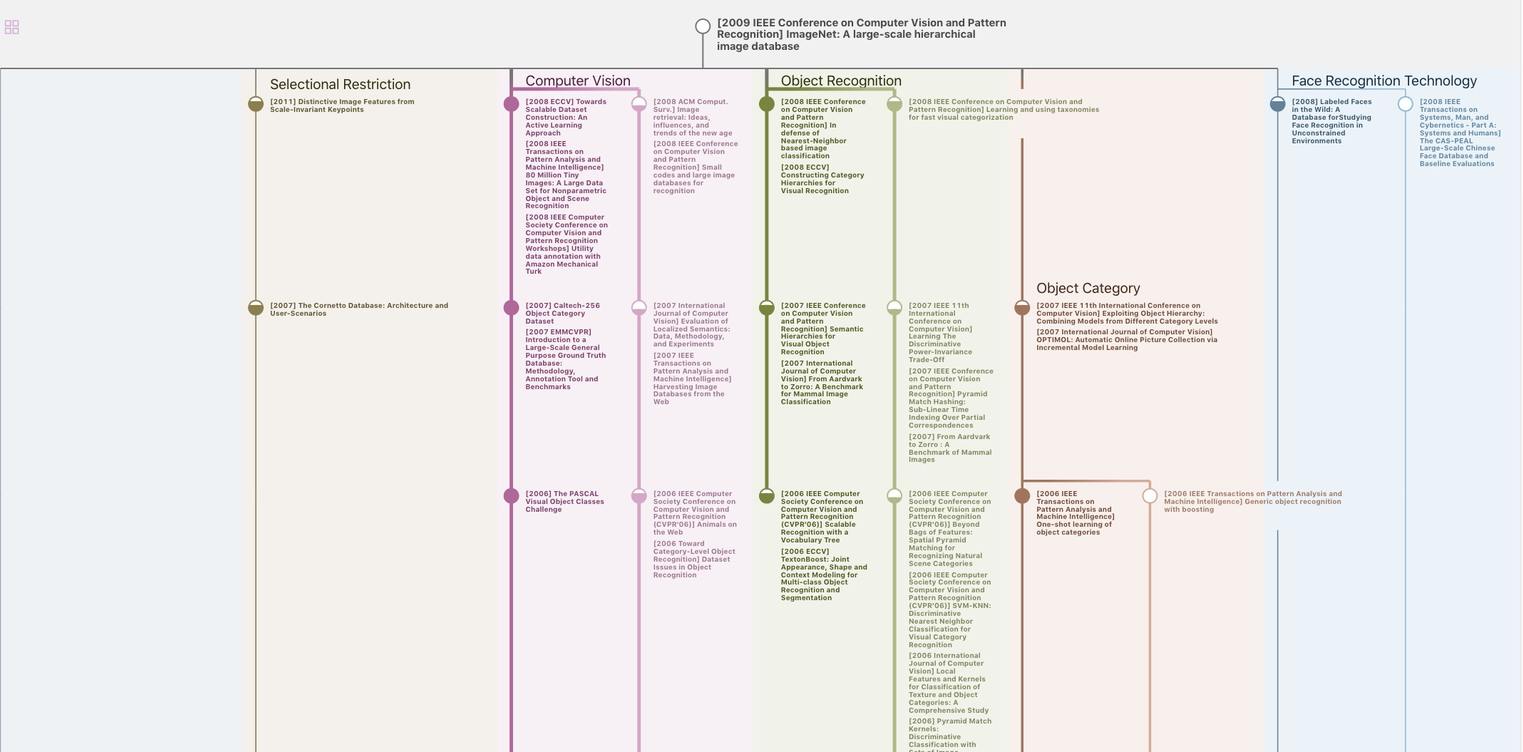
生成溯源树,研究论文发展脉络
Chat Paper
正在生成论文摘要