RAM: A Region-Aware Deep Model for Vehicle Re-Identification
2018 IEEE International Conference on Multimedia and Expo (ICME)(2018)
摘要
Previous works on vehicle Re-ID mainly focus on extracting global features and learning distance metrics. Because some vehicles commonly share same model and maker, it is hard to distinguish them based on their global appearances. Compared with the global appearance, local regions such as decorations and inspection stickers attached to the windshield, may be more distinctive for vehicle Re-ID. To embed the detailed visual cues in those local regions, we propose a Region-Aware deep Model (RAM). Specifically, in addition to extracting global features, RAM also extracts features from a series of local regions. As each local region conveys more distinctive visual cues, RAM encourages the deep model to learn discriminative features. We also introduce a novel learning algorithm to jointly use vehicle IDs, types/models, and colors to train the RAM. This strategy fuses more cues for training and results in more discriminative global and regional features. We evaluate our methods on two large-scale vehicle Re-ID datasets, i.e., VeRi and VehicleID. Experimental results show our methods achieve promising performance in comparison with recent works.
更多查看译文
关键词
Vehicle Re-ID,Deep Convolutional Neural Network (DCNN),Region-Aware Deep Model
AI 理解论文
溯源树
样例
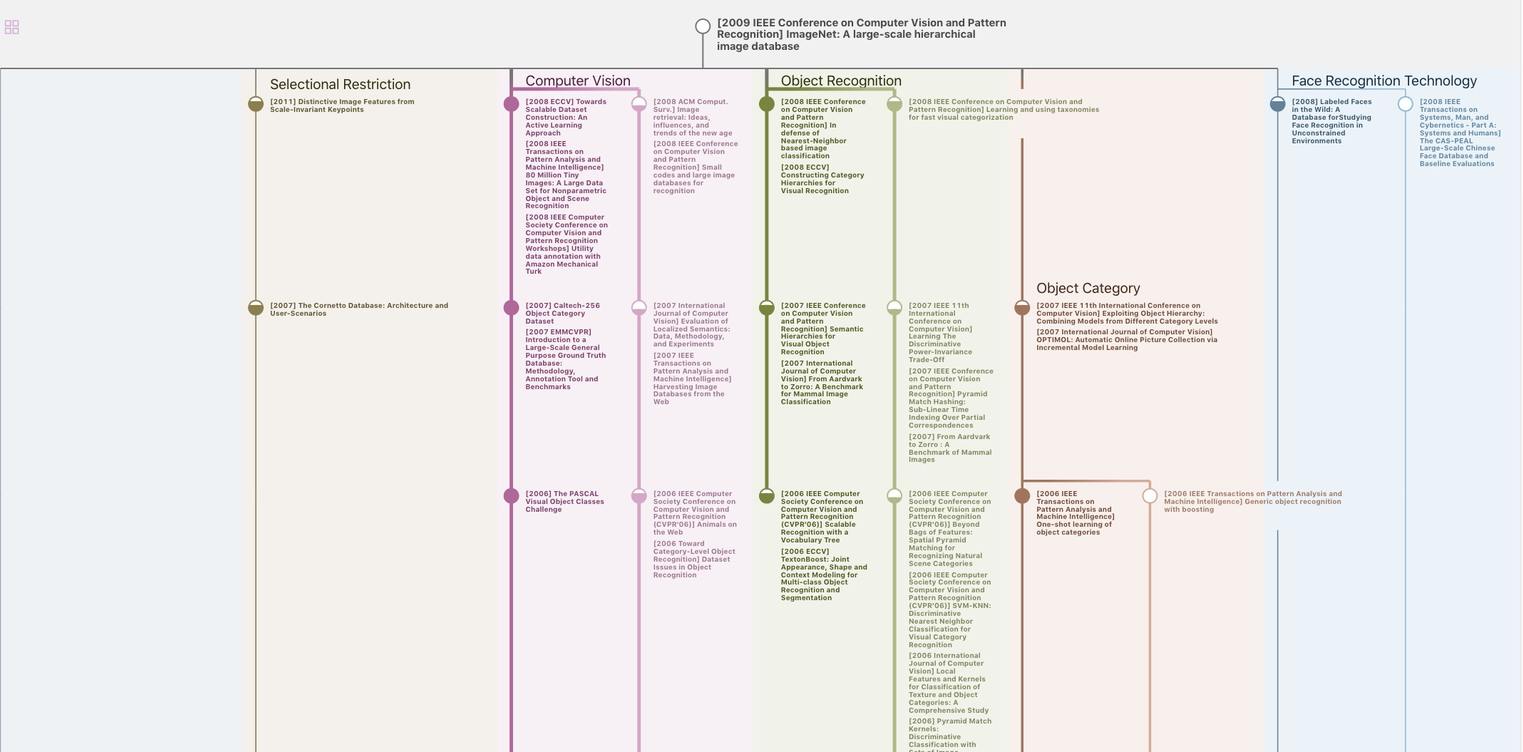
生成溯源树,研究论文发展脉络
Chat Paper
正在生成论文摘要