Low-Overhead Hierarchically-Sparse Channel Estimation for Multiuser Wideband Massive MIMO.
IEEE Transactions on Wireless Communications(2019)
摘要
Numerical evidence suggests that compressive sensing (CS) approaches for wideband massive MIMO channel estimation can achieve very good performance with limited training overhead by exploiting the sparsity of the physical channel. However, analytical characterization of the (minimum) training overhead requirements is still an open issue. By observing that the wideband massive MIMO channel can be represented by a vector that is not simply sparse but has well defined structural properties, referred to as hierarchical sparsity, we propose low complexity channel estimators for the uplink multiuser scenario that take this property into account. By employing the framework of the hierarchical restricted isometry property, rigorous performance guarantees for these algorithms are provided suggesting concrete design goals for the user pilot sequences. For a specific design, we analytically characterize the scaling of the required pilot overhead with increasing number of antennas and bandwidth, revealing that, as long as the number of antennas is sufficiently large, it is independent of the per user channel sparsity level as well as the number of active users. These analytical insights are verified by simulations demonstrating also the superiority of the proposed algorithm over conventional CS algorithms that ignore the hierarchical sparsity property.
更多查看译文
关键词
Channel estimation,Training,MIMO communication,Antennas,OFDM,Wideband
AI 理解论文
溯源树
样例
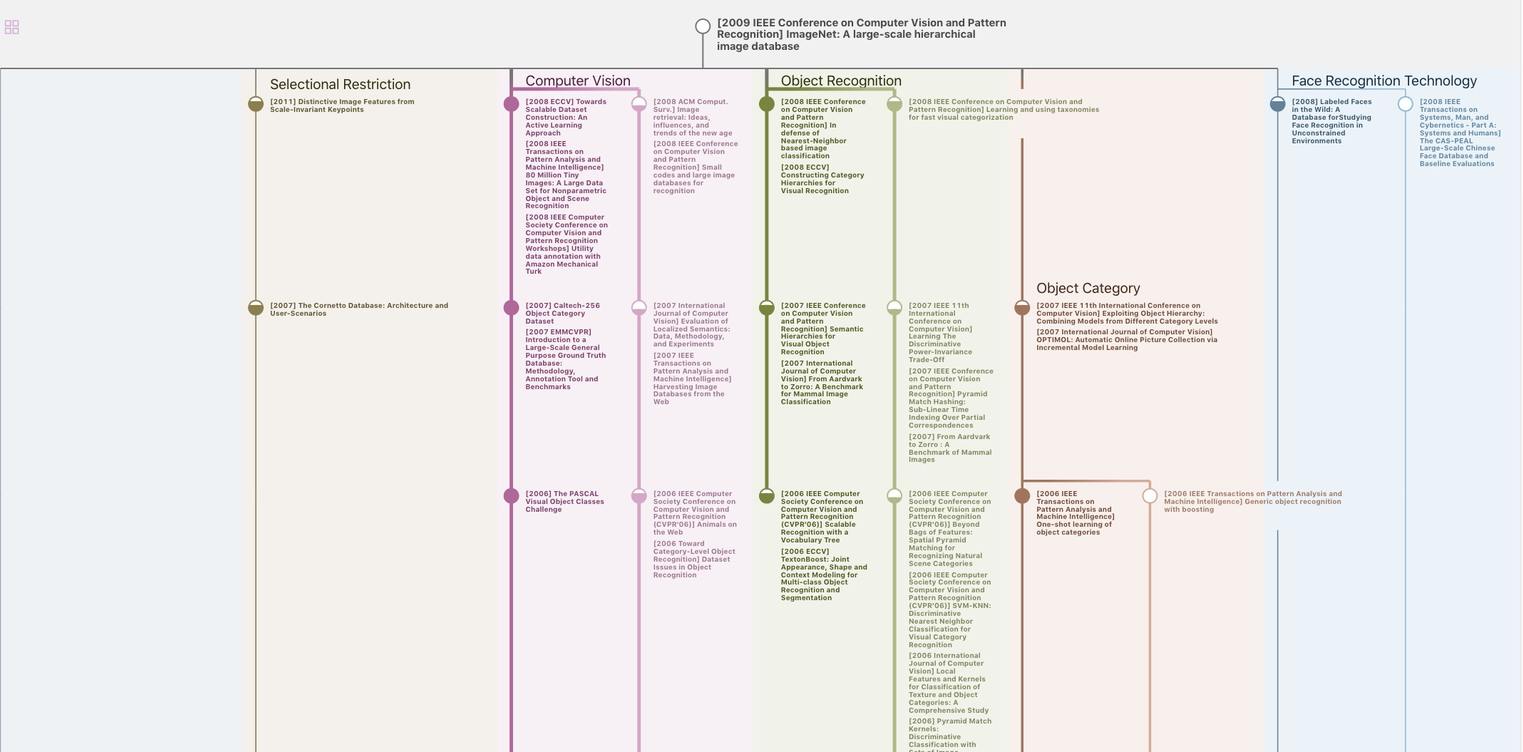
生成溯源树,研究论文发展脉络
Chat Paper
正在生成论文摘要