Selective Zero-Shot Classification with Augmented Attributes
Computer Vision – ECCV 2018: 15th European Conference, Munich, Germany, September 8–14, 2018, Proceedings, Part IX(2018)
摘要
In this paper, we introduce a selective zero-shot classification problem: how can the classifier avoid making dubious predictions? Existing attribute-based zero-shot classification methods are shown to work poorly in the selective classification scenario. We argue the under-complete human defined attribute vocabulary accounts for the poor performance. We propose a selective zero-shot classifier based on both the human defined and the automatically discovered residual attributes. The proposed classifier is constructed by firstly learning the defined and the residual attributes jointly. Then the predictions are conducted within the subspace of the defined attributes. Finally, the prediction confidence is measured by both the defined and the residual attributes. Experiments conducted on several benchmarks demonstrate that our classifier produces a superior performance to other methods under the risk-coverage trade-off metric.
更多查看译文
关键词
Zero-shot classification,Selective classification,Defined attributes,Residual attributes,Risk-coverage trade-off
AI 理解论文
溯源树
样例
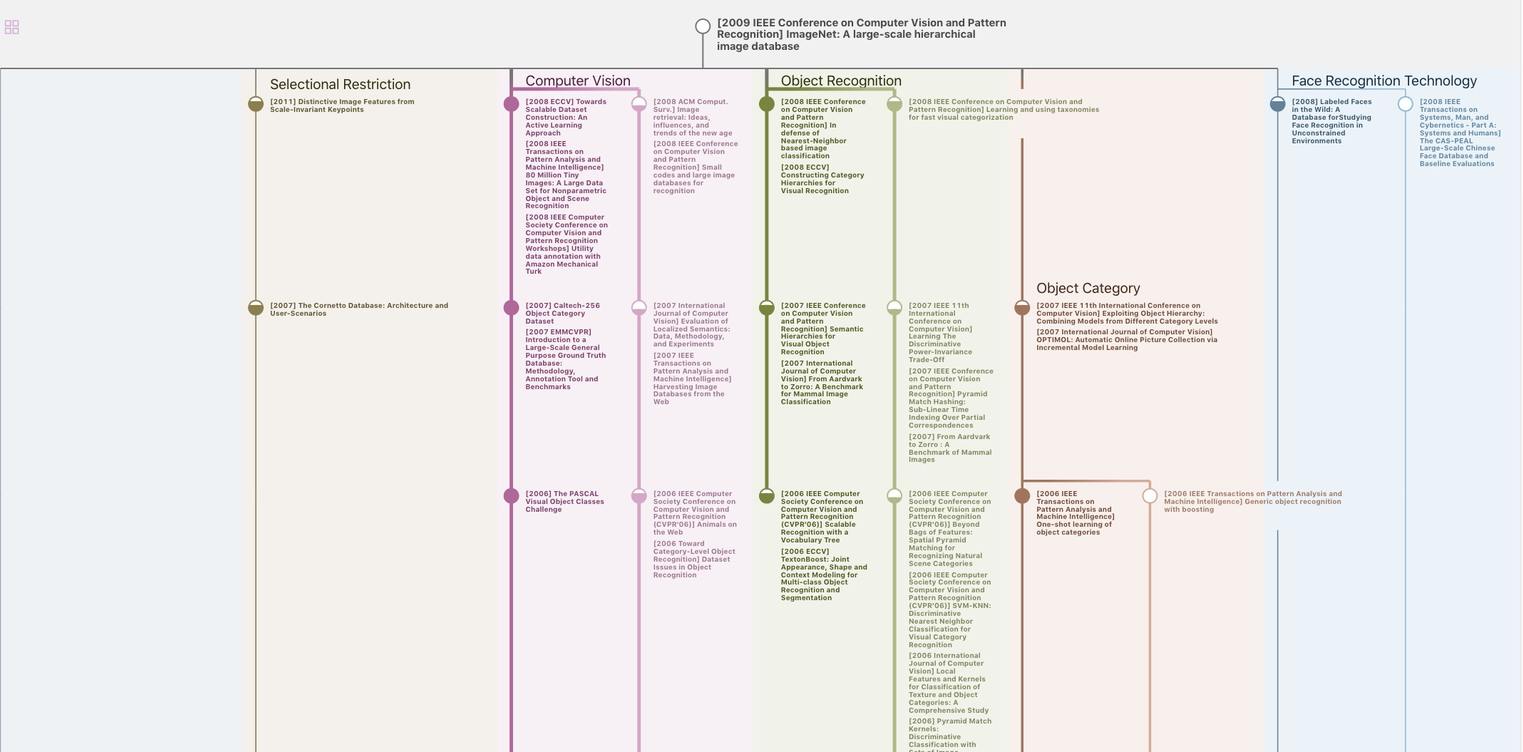
生成溯源树,研究论文发展脉络
Chat Paper
正在生成论文摘要