A Stacking Model For Variation Prediction Of Public Bicycle Traffic Flow
INTELLIGENT DATA ANALYSIS(2018)
摘要
Public bicycle system can improve the public transport travel efficiency and reduce environmental pollution, which has been deployed in many cities all over the world. However, the bicycle usages become quite skewed and imbalanced in different stations. A system which could recommend the nearest available stations for passengers whether they are looking for a dock or a bike, is of great importance. Monitoring the current number of docks or bikes at each station cannot tackle this problem because it's too late to recommend the station for passengers to rent or return bikes after the imbalance has occurred. To address this issue, we propose a stacking model for variation prediction of public bicycle traffic flow called SMVP based on the real-world datasets. The stacking model integrates multiple base models which we trained by different combinations of features so that it could get better performance. We adopt a machine learning system called XGBoost [25] to train the models and construct the multiple complex factors which impact the public bicycle traffic flow. The traditional factors, such as temporal, spatial, historical and meteorological factors are taken into consideration. A new clustering factor which considers both the geographical positions and transition patterns of stations is also proposed in this framework and then we use the K-Medoids algorithm [12] to cluster stations into groups by constructing a new different station relation matrix which considers these two factors as the distance between different stations. The performance of SMVP is improved on the datasets of Hangzhou and New York City, especially in terms of Coefficient of Determination improved by 25.58% in Hangzhou, compared with the traditional stacking [5] and single model respectively.
更多查看译文
关键词
Public bicycle, traffic flow variation prediction, stacking, xgboost, k-medoids, data mining, machine learning
AI 理解论文
溯源树
样例
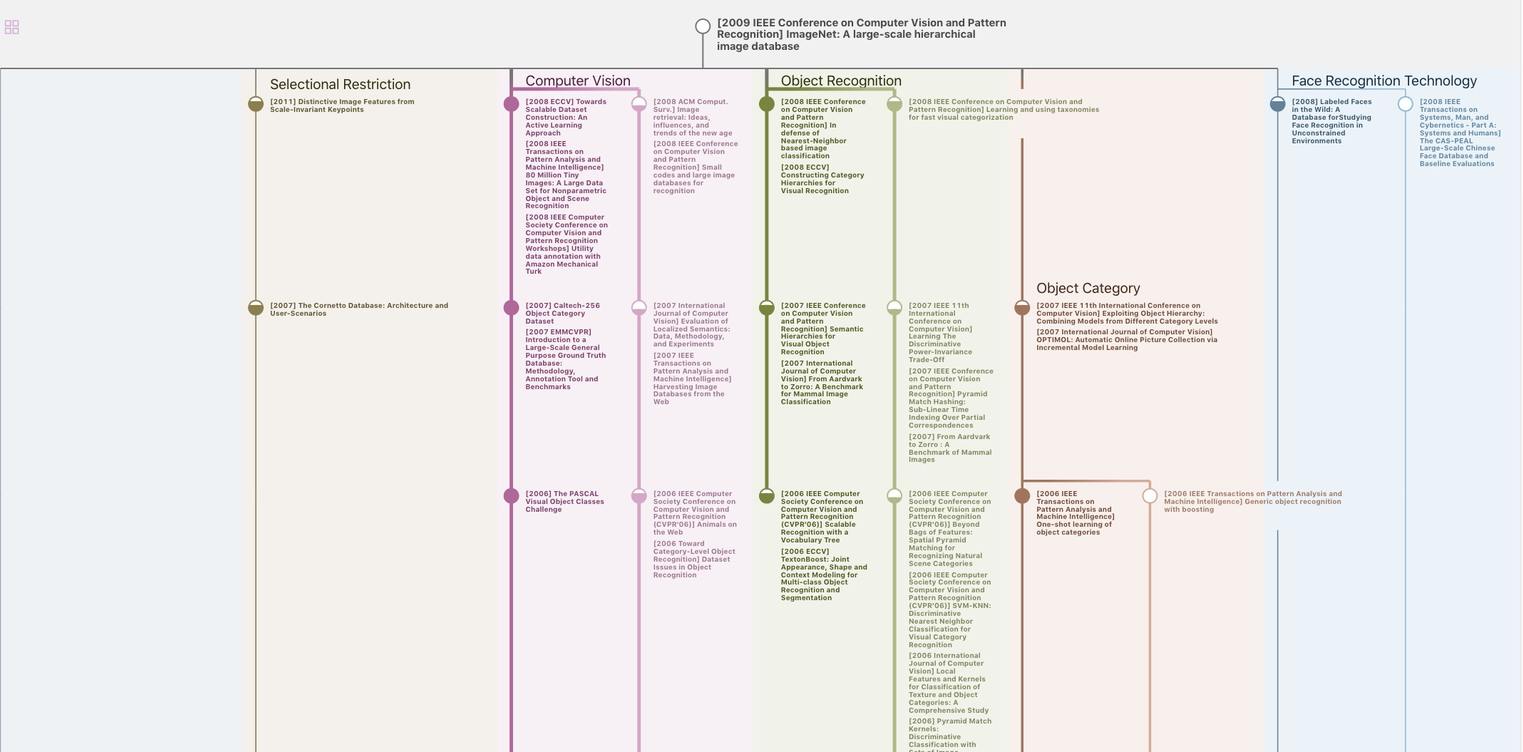
生成溯源树,研究论文发展脉络
Chat Paper
正在生成论文摘要