Online Versus Offline Reinforcement Learning for False Target Control Against Known Threat
INTELLIGENT ROBOTICS AND APPLICATIONS (ICIRA 2018), PT II(2018)
摘要
In this article, we investigate the performances of different learning approaches for decentralised non-cooperative multi-agent system applied to defend a high-value target from multiple aerial threats for an air defence application. We focus mainly on reinforcement learning (RL) techniques for protection against known fully observable threats with high mobility. We implement two well-known algorithms from two different approaches, including the regret matching (online learning) and the Q-learning with artificial neural networks (offline learning), and compare them to understand their efficiency. Numerical experiments are provided to illustrate the performances of the different learning algorithms under various approaching directions of the threat as well as under collision avoidance with both static and moving obstacles. Finally, discussions for further improvements of these RL techniques are also provided.
更多查看译文
关键词
Decentralised algorithms,Reinforcement learning,Multi-agent distributed control,Intelligent autonomous systems
AI 理解论文
溯源树
样例
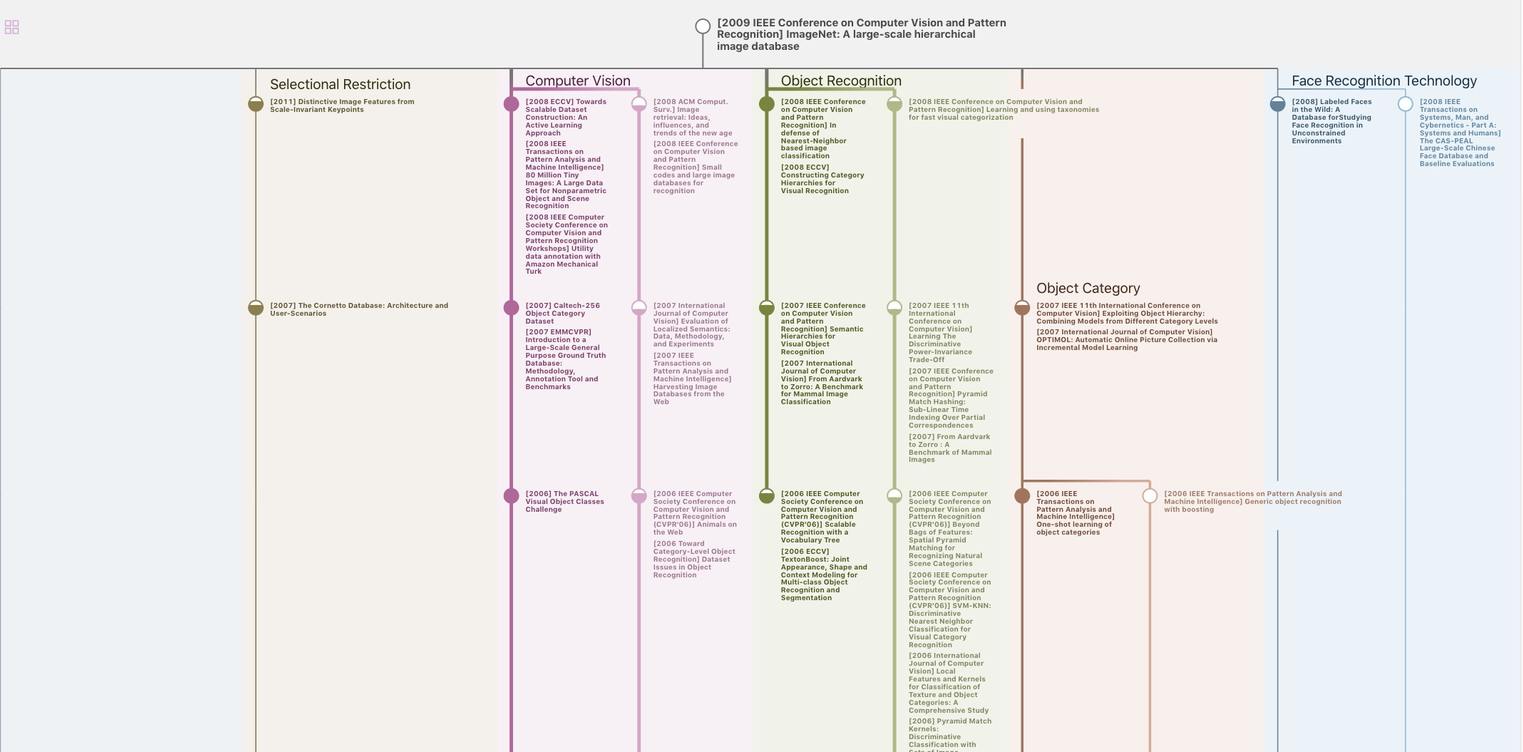
生成溯源树,研究论文发展脉络
Chat Paper
正在生成论文摘要