Learning Automata Based Feature Selection for Network Traffic Intrusion Detection
2018 IEEE Third International Conference on Data Science in Cyberspace (DSC)(2018)
摘要
Considering the sharp increasing flow of network traffic, it is extremely emergency to detect malicious and anomalous network traffic to protect network security. Network Intrusion Detection Systems (NIDS) can be used to detect normal and anomalous behavior by analyzing network traffic. However, network traffic data consist of large quantities of features, increasing the difficulty of detection. These features contain many redundant features, which can affect the effectiveness of detection. In this paper, a method of learning automata is proposed to select the optimal and significant features for network traffic intrusion detection. Multiple LAs collaboratively remove redundant features and fully consider the redundancy of single feature and multiple combined features. The experiments on KDD99 show that the proposed feature selection scheme can effectively reduce feature dimension and select the optimal feature subset. It can also achieves higher detection accuracy compared with other feature selection methods.
更多查看译文
关键词
network traffic intrusion detection,feature selection,learning automata
AI 理解论文
溯源树
样例
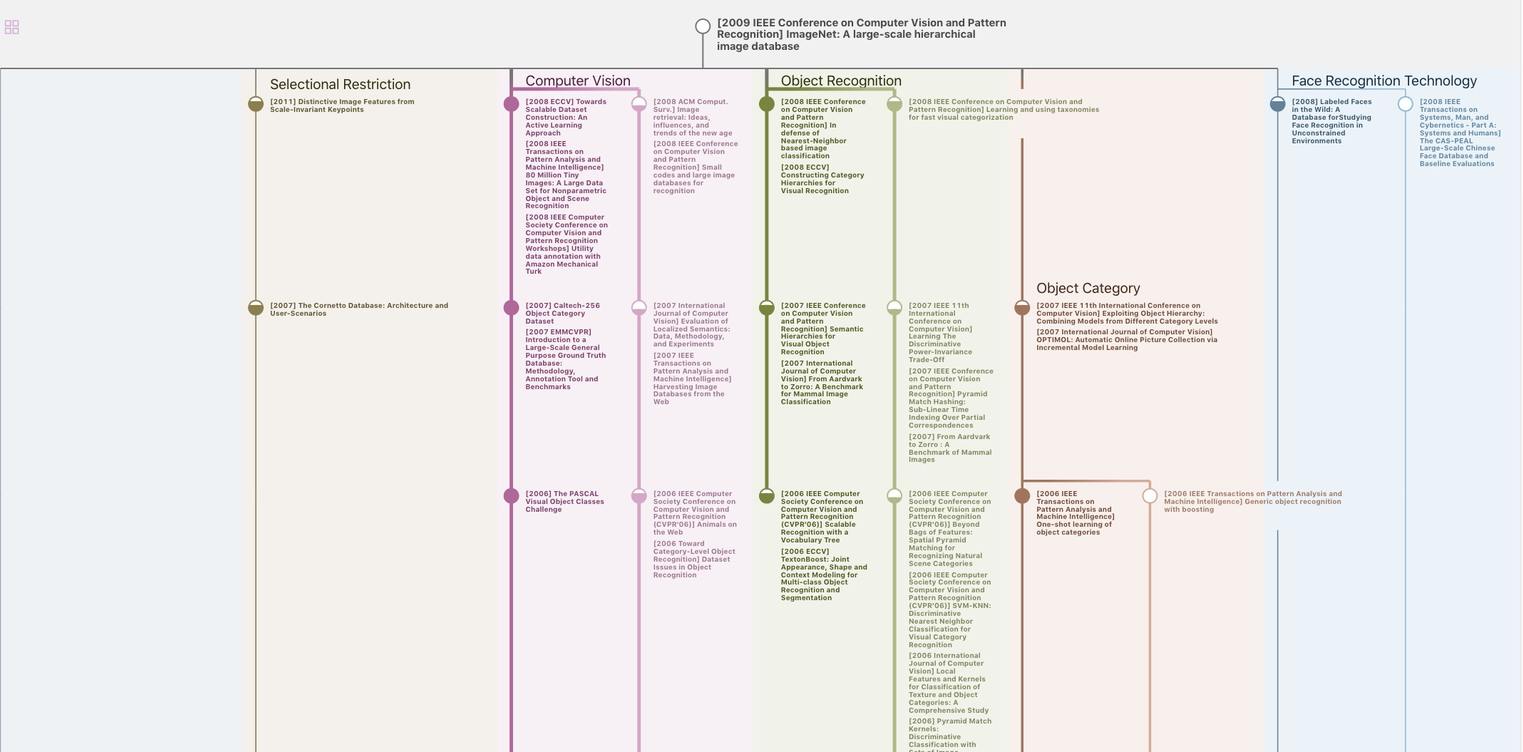
生成溯源树,研究论文发展脉络
Chat Paper
正在生成论文摘要