Deep Homography Estimation with Pairwise Invertibility Constraint.
Lecture Notes in Computer Science(2018)
摘要
Recent works have shown that deep learning methods can improve the performance of the homography estimation due to the better features extracted by convolutional networks. Nevertheless, these works are supervised and rely too much on the labeled training dataset as they aim to make the homography be estimated as close to the ground truth as possible, which may cause overfitting. In this paper, we propose a Siamese network with pairwise invertibility constraint for supervised homography estimation. We utilize spatial pyramid pooling modules to improve the quality of extracted features in each image by exploiting context information. Discovering the fact that there is a pair of homographies from a given image pair which are inverse matrices, we propose the invertibility constraint to avoid overfitting. To employ the constraint, we adopt the matrix representation of the homography rather than the commonly used 4-point parameterization in other methods. Experiments on the synthetic dataset generated from MSCOCO dataset show that our proposed method outperforms several state-of-the-art approaches.
更多查看译文
关键词
Homography estimation,Supervised deep learning,Invertibility constraint,Spatial pyramid pooling
AI 理解论文
溯源树
样例
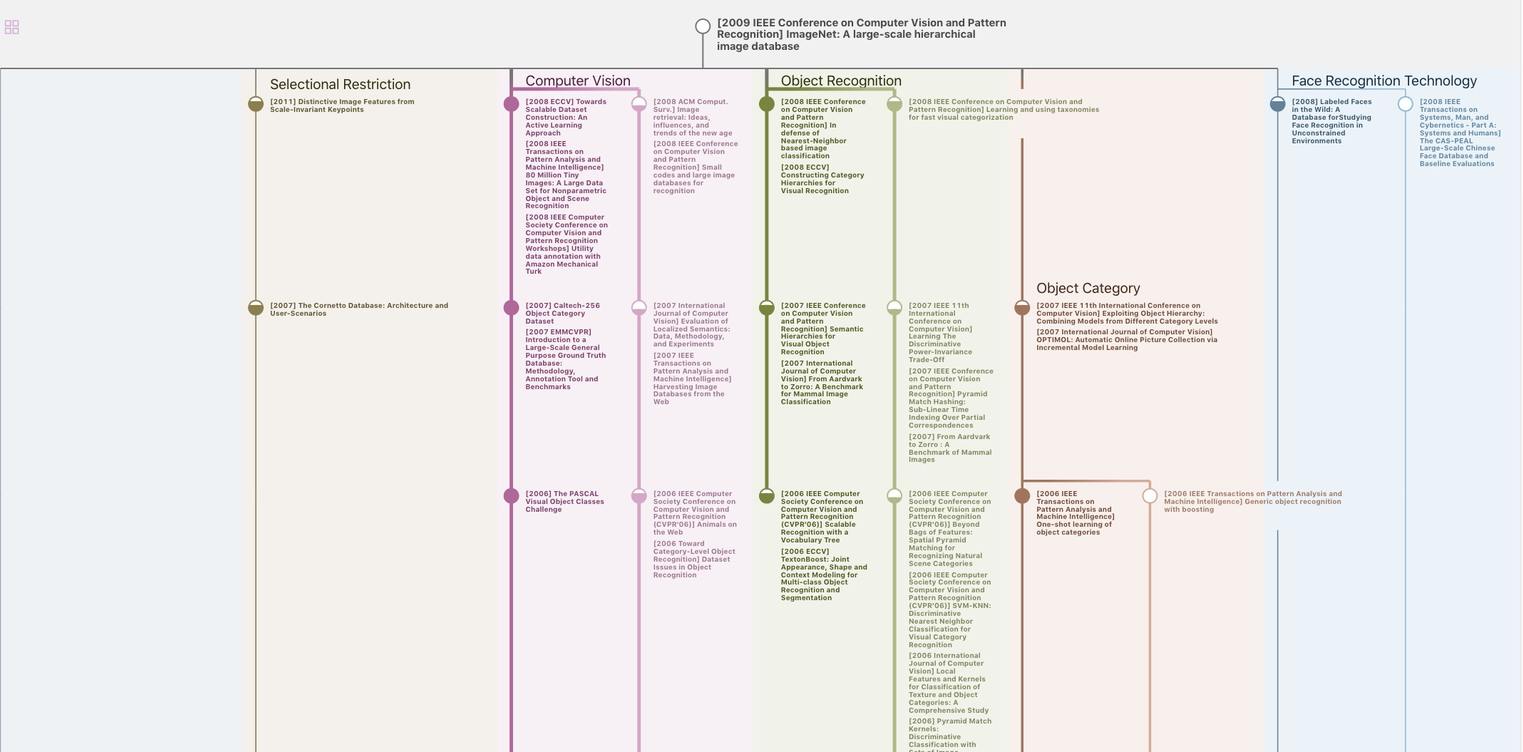
生成溯源树,研究论文发展脉络
Chat Paper
正在生成论文摘要