Limited Feedback Double Directional Massive Mimo Channel Estimation: From Low-Rank Modeling To Deep Learning
2018 IEEE 19TH INTERNATIONAL WORKSHOP ON SIGNAL PROCESSING ADVANCES IN WIRELESS COMMUNICATIONS (SPAWC)(2018)
摘要
In frequency division duplex massive MIMO systems, one critical challenge is that the mobiles need to feed back a large downlink channel matrix to the base station, creating large signaling overhead. Estimating a large downlink channel matrix at the mobile may also be costly in terms of power and memory consumption. Prior work addresses these issues using appropriate angle parameterization and compressed sensing techniques, but this approach involves solving a challenging, and sometimes extremely large, sparse inverse problem-which is difficult to solve to global optimality, and often leads to unaffordable memory and computational costs.In this work, we propose an alternative framework that explores the fact that double directional channels for mmWave massive MIMO usually have low rank. The base station estimates the downlink channel via recovering a low-rank matrix, utilizing samples of the channel matrix compressed and fed back from the mobiles. This way, the mobile users can avoid performing resource-consuming tasks. In addition, the number of feedback measurements can be much smaller than the size of the channel matrix without losing channel recovery guarantees. Further, the low-rank estimation problem at the base station has a manageable size that scales gracefully with the channel size. Based on the new model, we propose two methods for channel estimation, which are based on iterative optimization and deep learning, respectively. Compared with the state-of-the-art, the optimization method obtains 10x improvement and the deep learning approach achieves up to 1000x improvement in computational complexity, while achieving high estimation quality in very low sample region.
更多查看译文
关键词
memory consumption,computational costs,mmWave massive MIMO,base station,low-rank matrix,channel recovery guarantees,low-rank estimation problem,channel size,deep learning approach,high estimation quality,low sample region,limited feedback double directional massive MIMO channel estimation,low-rank modeling,frequency division duplex massive MIMO systems,inverse problem,angle parameterization,signaling overhead,downlink channel matrix estimation,compressed sensing techniques,feedback measurements,iterative optimization,optimization method,computational complexity
AI 理解论文
溯源树
样例
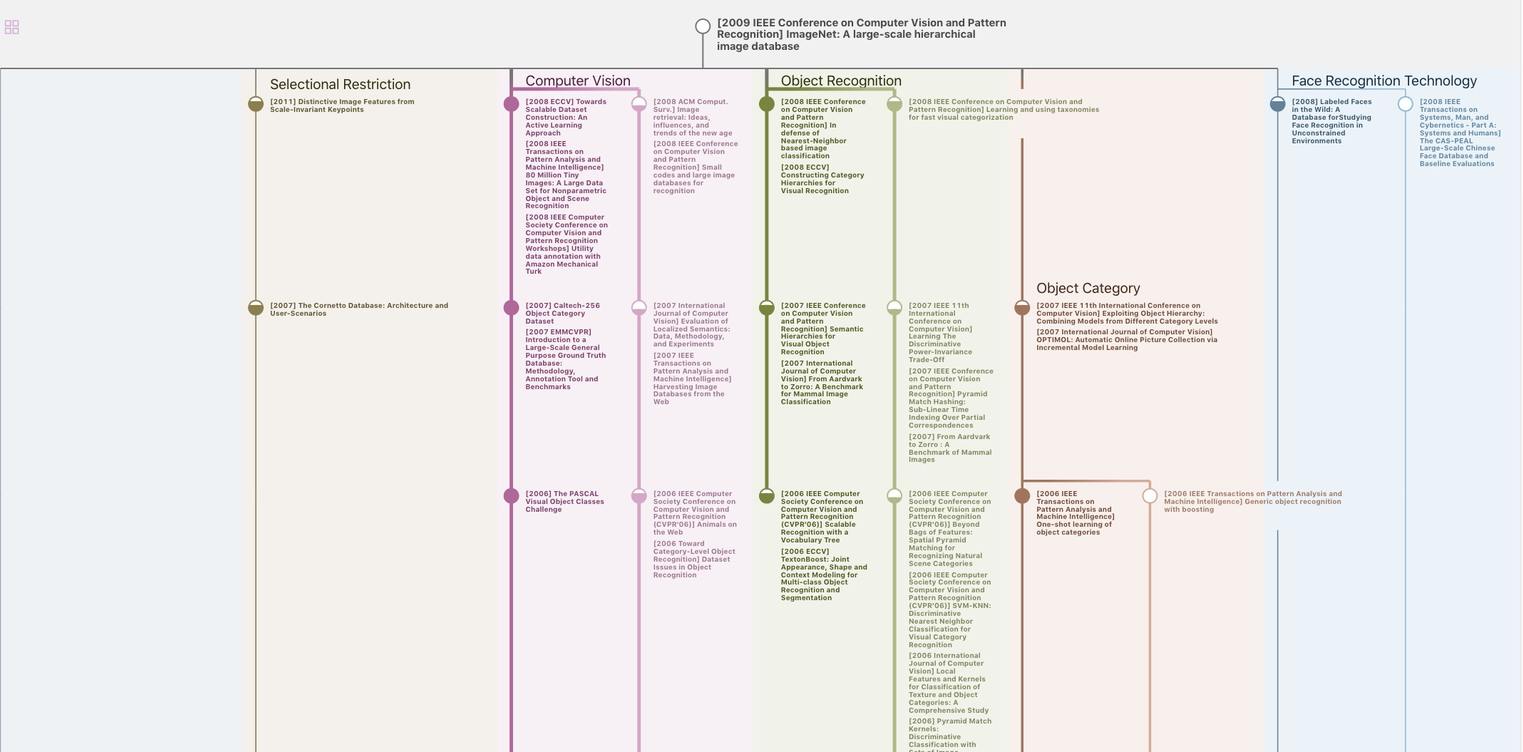
生成溯源树,研究论文发展脉络
Chat Paper
正在生成论文摘要