A Comparative Study Of Synthetic Dataset Generation Techniques
DATABASE AND EXPERT SYSTEMS APPLICATIONS (DEXA 2018), PT II(2018)
摘要
Unrestricted availability of the datasets is important for the researchers to evaluate their strategies to solve the research problems. While publicly releasing the datasets, it is equally important to protect the privacy of the respective data owners. Synthetic datasets that preserve the utility while protecting the privacy of the data owners stands as a midway.There are two ways to synthetically generate the data. Firstly, one can generate a fully synthetic dataset by subsampling it from a synthetically generated population. This technique is known as fully synthetic dataset generation. Secondly, one can generate a partially synthetic dataset by synthesizing the values of sensitive attributes. This technique is known as partially synthetic dataset generation. The datasets generated by these two techniques vary in their utilities as well as in their risks of disclosure.We perform a comparative study of these techniques with the use of different dataset synthesisers such as linear regression, decision tree, random forest and neural network. We evaluate the effectiveness of these techniques towards the amounts of utility that they preserve and the risks of disclosure that they suffer. We find decision tree to be an efficient and a competitively effective dataset synthesiser.
更多查看译文
关键词
Synthetic datasets, Risk of disclosure, Privacy, Utility
AI 理解论文
溯源树
样例
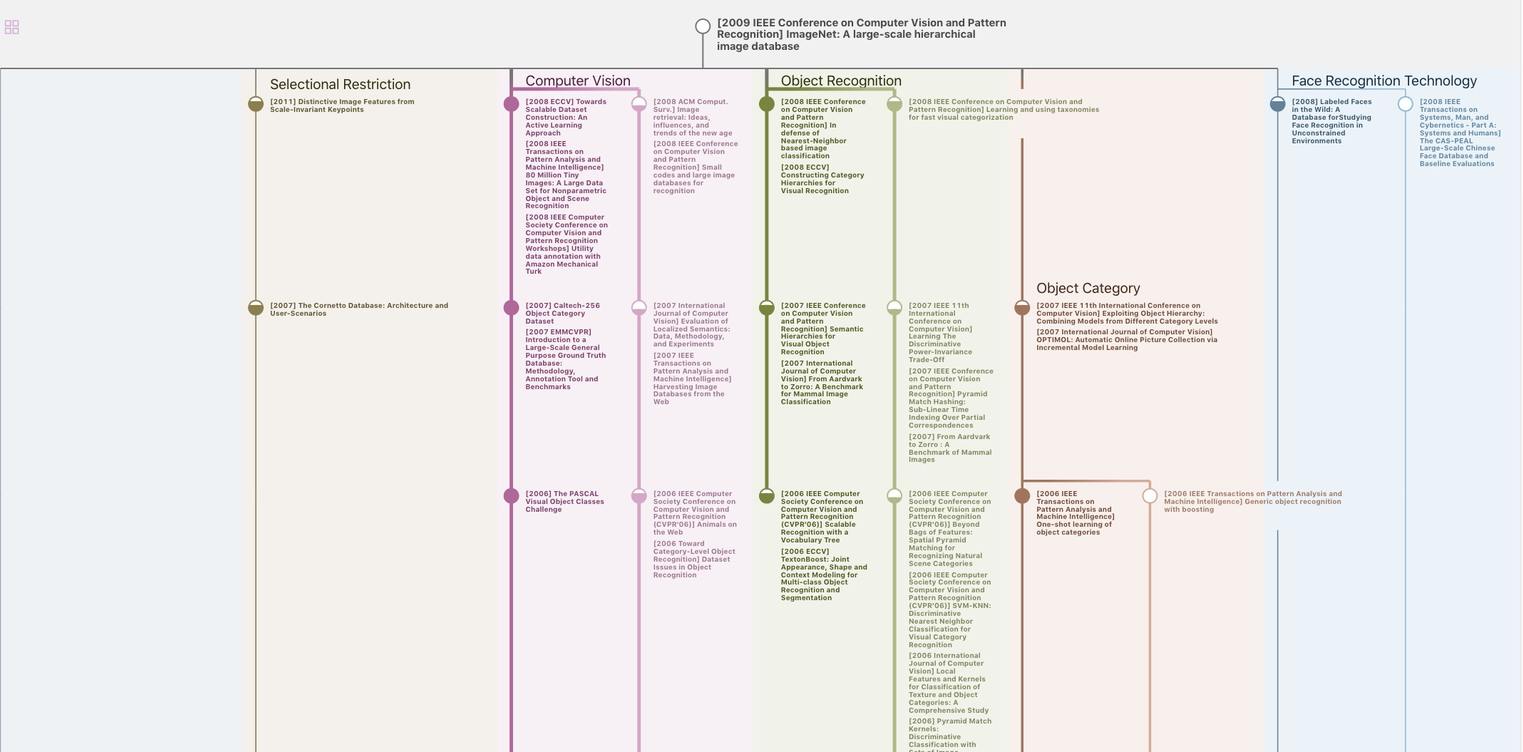
生成溯源树,研究论文发展脉络
Chat Paper
正在生成论文摘要