Matching Convolutional Neural Networks without Priors about Data
2018 IEEE Data Science Workshop (DSW)(2018)
摘要
We propose an extension of Convolutional Neural Networks (CNNs) to graph-structured data, including strided convolutions and data augmentation on graphs. Our method matches the accuracy of state-of-the-art CNNs when applied on images, without any prior about their 2D regular structure. On fMRI data, we obtain a significant gain in accuracy compared with existing graph-based alternatives.
更多查看译文
关键词
priors,strided convolutions,inferred graph translations,2D regular structure,fMRI data,graph-based alternatives,convolutional neural networks matching,data augmentation,graph-structured data
AI 理解论文
溯源树
样例
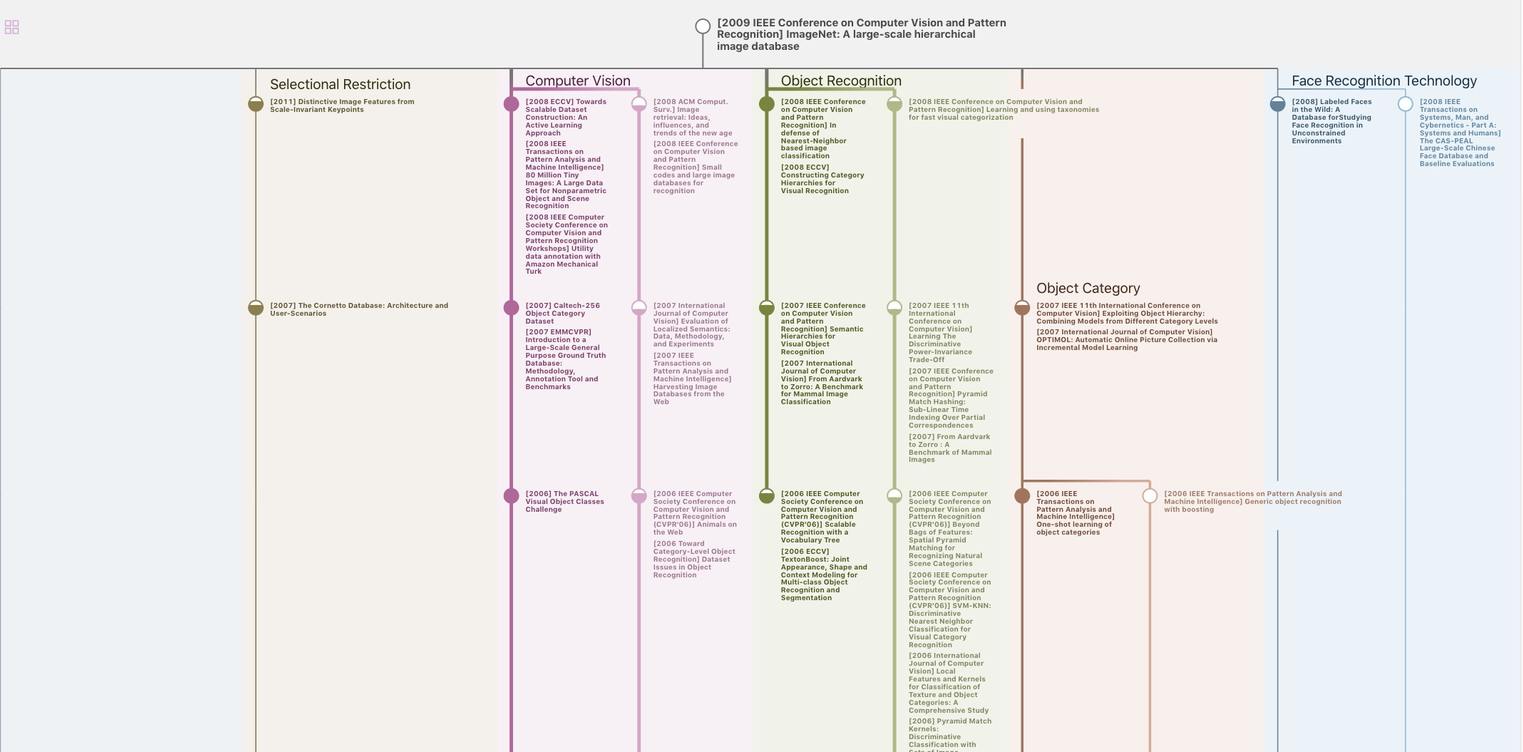
生成溯源树,研究论文发展脉络
Chat Paper
正在生成论文摘要