Task oriented 3D sampling via genetic algorithms.
Signal Processing and Communications Applications Conference(2018)
摘要
With the profiliferation of 3D sensors, 3D data analysis has become an important tool. Due to its capability of data summarization, sampling has taken a critical part of this analysis. In this paper, we argue that finding a universally good sampling is difficult, if not impossible and rather propose a task-tailored solution. In the scope of this work, we address the task of Iterative Closest Point (ICP) registration and cast the point selection procedure as an optimization problem over the samples, minimizing the ICP energy. Because the parametrization over 3D points results in a discrete and large optimization problem, we benefit from genetic algorithms, which are proven to evolve into better solutions, at each time-step. The quantitative and qualitative results clearly demonstrate that we are able to obtain better results than the state-of-the-art.
更多查看译文
关键词
3D sampling,iterative closest point (ICP),genetic algorithms
AI 理解论文
溯源树
样例
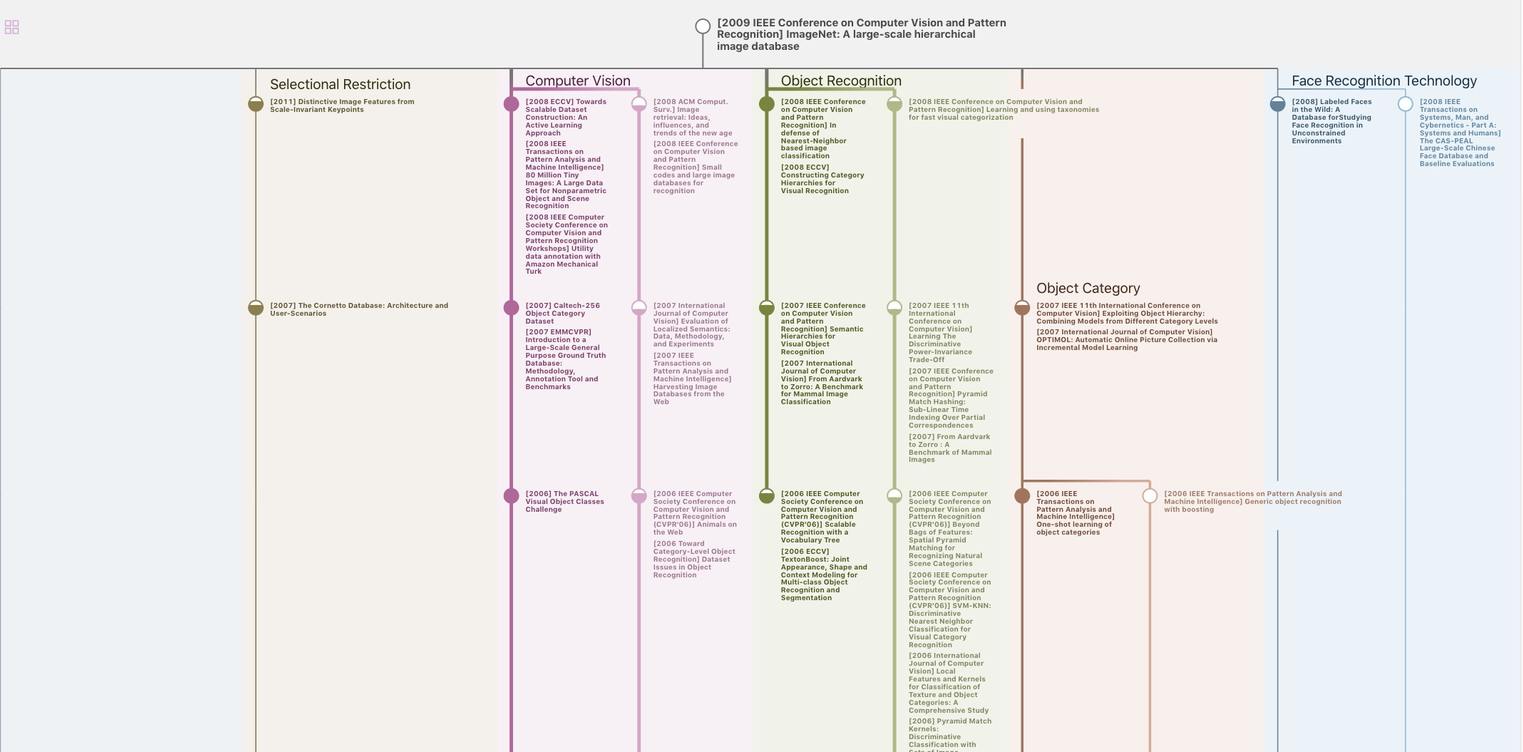
生成溯源树,研究论文发展脉络
Chat Paper
正在生成论文摘要