Deep Recurrent And Convolutional Networks For Accelerated Fault Tolerant Adaptive Flight Control Under Severe Failures
2018 ANNUAL AMERICAN CONTROL CONFERENCE (ACC)(2018)
摘要
Design of fault tolerant systems is a popular subject in flight control system design. In particular, adaptive control approach has been successful in recovering aircraft in a wide variety of different actuator/sensor failure scenarios. However, if the aircraft goes under a severe actuator failure, control system might not be able to adapt fast enough to changes in the dynamics, which would result in performance degradation or even loss of the aircraft. Inspired by the recent success of deep learning applications, this work builds a hybrid recurrent/convolutional neural network model to estimate adaptation parameters for aircraft dynamics under actuator/engine faults. The model is trained offline from a database of different failure scenarios. In case of an actuator/engine failure, the model identifies adaptation parameters and feeds this information to the adaptive control system, which results in significantly faster convergence of the controller coefficients. Developed control system is implemented on a nonlinear 6-DOF F-16 aircraft, and the results show that the proposed architecture is especially beneficial in severe failure scenarios.
更多查看译文
关键词
controller coefficients,nonlinear 6-DOF F-16 aircraft,accelerated fault tolerant adaptive flight control,flight control system design,deep learning applications,aircraft dynamics,adaptation parameters estimation,recurrent neural network model,actuator-engine faults,convolutional neural network model,actuator-sensor failure scenarios,adaptive control system design
AI 理解论文
溯源树
样例
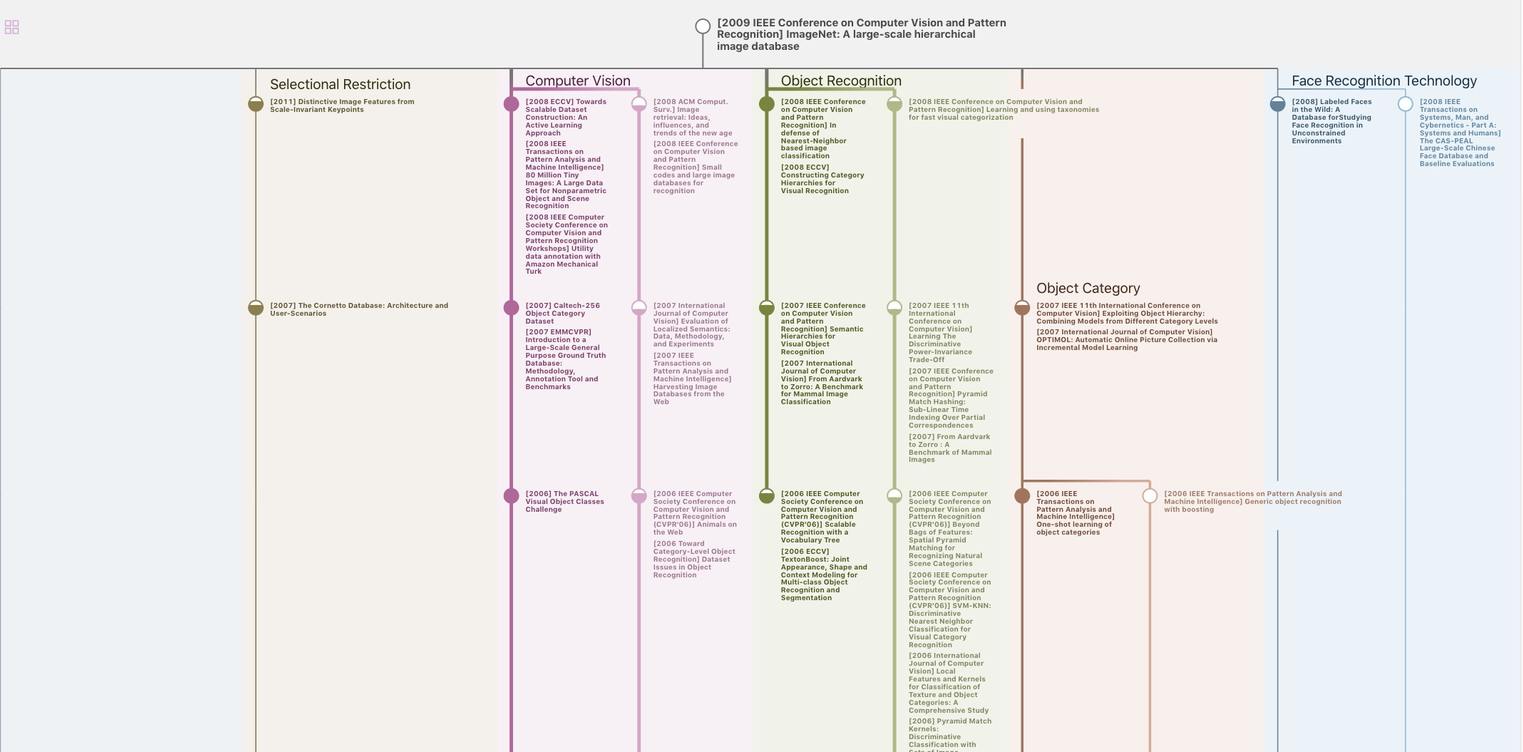
生成溯源树,研究论文发展脉络
Chat Paper
正在生成论文摘要