Data-based reinforcement learning approximate optimal control for an uncertain nonlinear system with control effectiveness faults.
2018 ANNUAL AMERICAN CONTROL CONFERENCE (ACC)(2020)
摘要
An infinite horizon approximate optimal control problem is developed for a system with unknown drift parameters and control effectiveness faults. A data-based filtered parameter estimator with a novel dynamic gain structure is developed to simultaneously estimate the unknown drift dynamics and control effectiveness fault. A local state-following approximate dynamic programming method is used to approximate the unknown optimal value function for an uncertain system. Using a relaxed persistence of excitation condition, a Lyapunov-based stability analysis shows exponential convergence to a residual error for the parameter estimation and uniformly ultimately bounded convergence for the closed-loop system. Simulation results are presented which demonstrate the effectiveness of the developed method.
更多查看译文
关键词
approximate optimal control,uncertain nonlinear system,reinforcement,nonlinear system,data-based
AI 理解论文
溯源树
样例
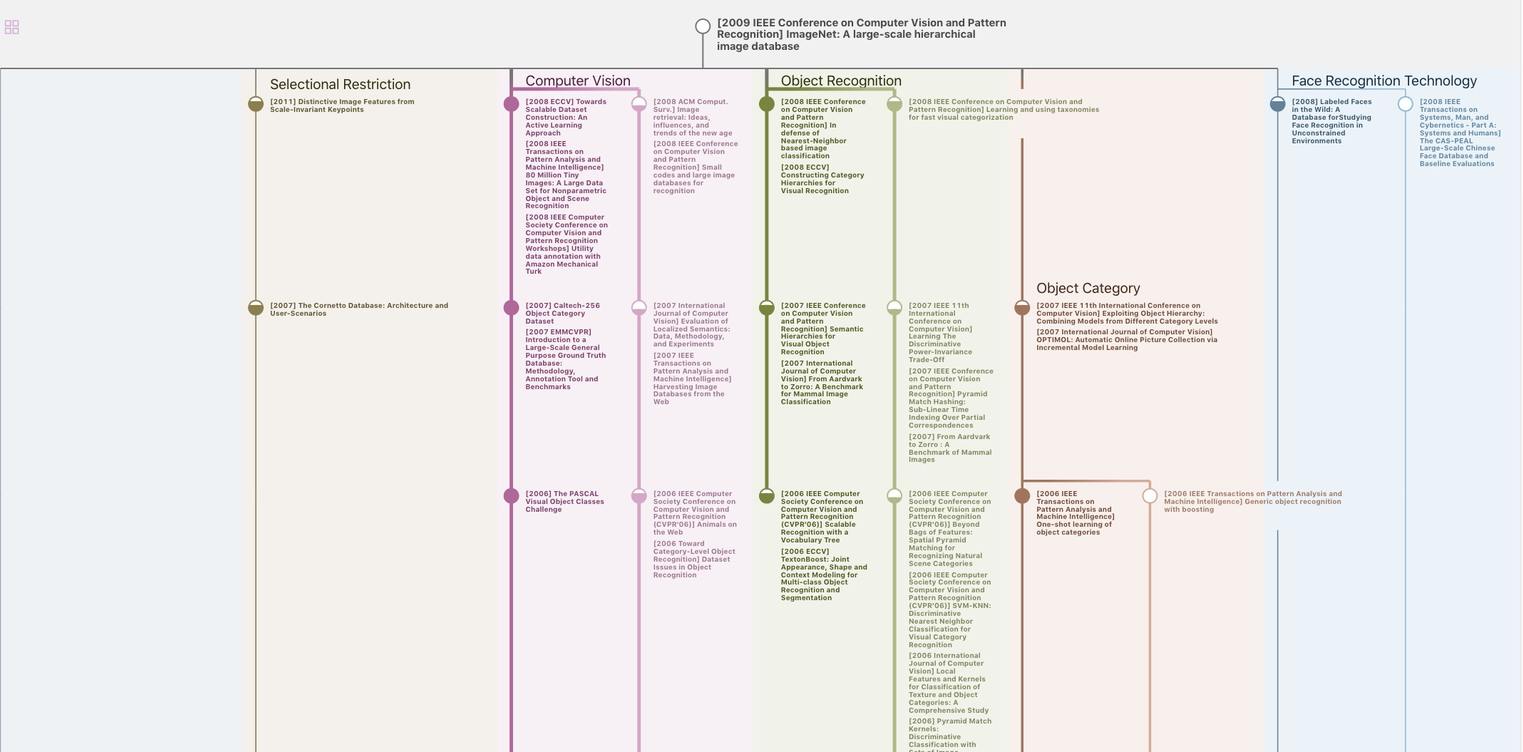
生成溯源树,研究论文发展脉络
Chat Paper
正在生成论文摘要