Off-the-shelf CNN features for 3D object retrieval
Multimedia Tools Appl.(2017)
摘要
Effective feature representation is crucial to view-based 3D object retrieval (V3OR). Most previous works employed hand-crafted features to represent the views of each object. Although deep learning based methods has shown its excellent performance in many vision tasks, it is hard to get excellent performance for unsupervised 3D object retrieval. In this paper, we propose to combine the off-the-shelf deep model and graph model to retrieve unseen objects. By employing the powerful deep classification models which are trained from millions of images, we obtain significant improvements compared with state of the art methods. We validate the effectiveness of the ready CNN from other domains that can greatly facilitate the representative ability of objects’ views. In addition, we analyze the representative abilities of different fully connected layers for V3OR, and propose to employ multigraph learning to fuse the deep features of different layers. The autoencoder is then explored to improve the retrieval speed to a large extent. Experiments on two popular datasets are carried out to demonstrate the effectiveness of the proposed method.
更多查看译文
关键词
3D object retrieval, Deep learning, CNN, Autoencoder, Multigraph learning, Unseen objects
AI 理解论文
溯源树
样例
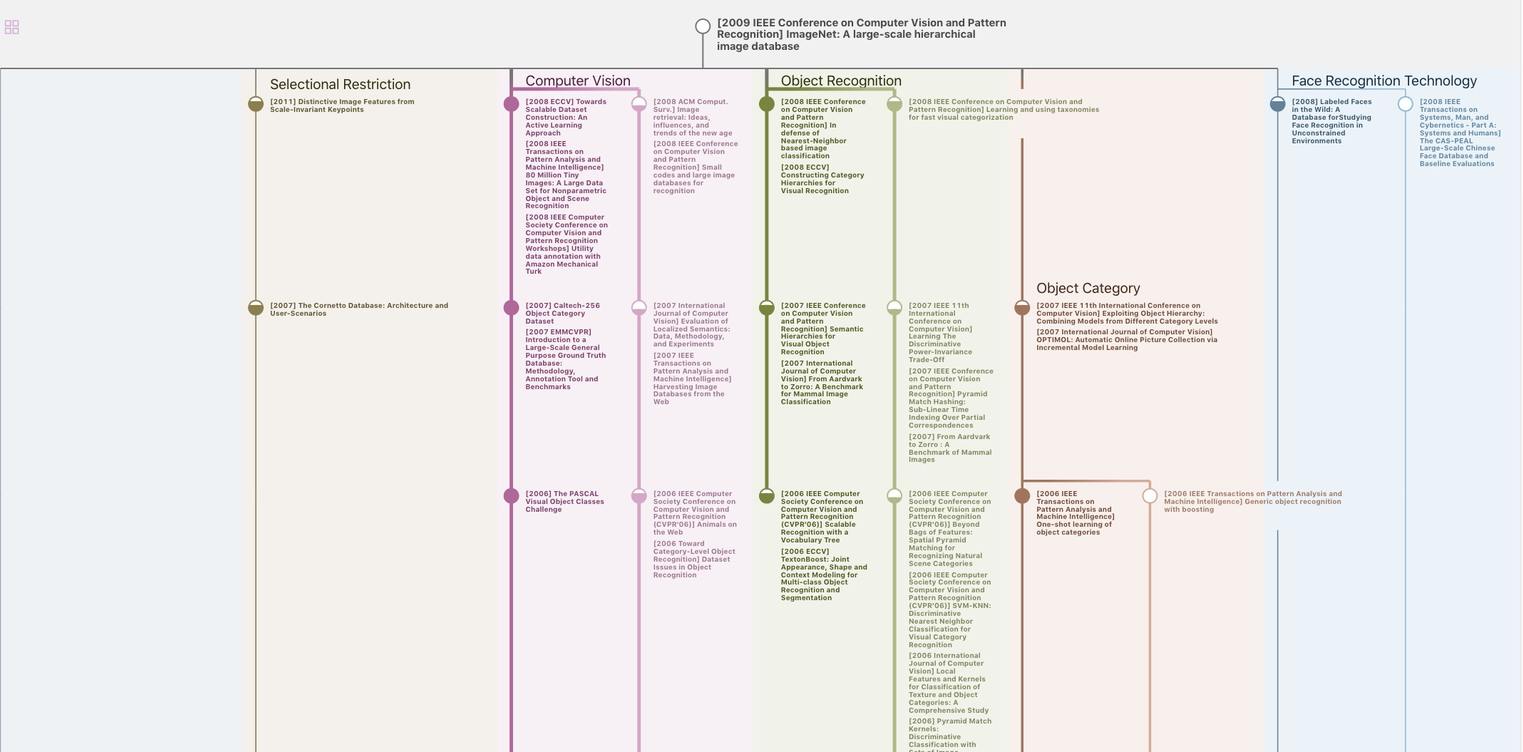
生成溯源树,研究论文发展脉络
Chat Paper
正在生成论文摘要