Autoscaling scientific workflows on the cloud by combining on-demand and spot instances.
COMPUTER SYSTEMS SCIENCE AND ENGINEERING(2017)
摘要
Autoscaling strategies achieve efficient and cheap executions of scientific workflows running in the cloud by determining appropriate type and amount of virtual machine instances to use while scheduling the tasks/data. Current strategies only consider on-demand instances ignoring the advantages of a mixed cloud infrastructure comprising also spot instances. Although the latter type of instances are subject to failures and therefore provide an unreliable infrastructure, they potentially offer significant cost and time improvements if used wisely. This paper discusses a novel autoscaling strategy with two features. First, it combines both types of instances to acquire a better cost-performance balance in the infrastructure. And second, it uses heuristic scheduling to deal with the unreliability of spot instances. Simulated experiments based on 4 scientific workflows showed substantial makespan and cost reductions of our strategy when compared with a reference strategy from the state of the art entitled Scaling First. These promising results represent a step towards new and better strategies for workflow autoscaling in the cloud.
更多查看译文
关键词
scientific workflows,cloud computing,autoscaling,scheduling,spot instances
AI 理解论文
溯源树
样例
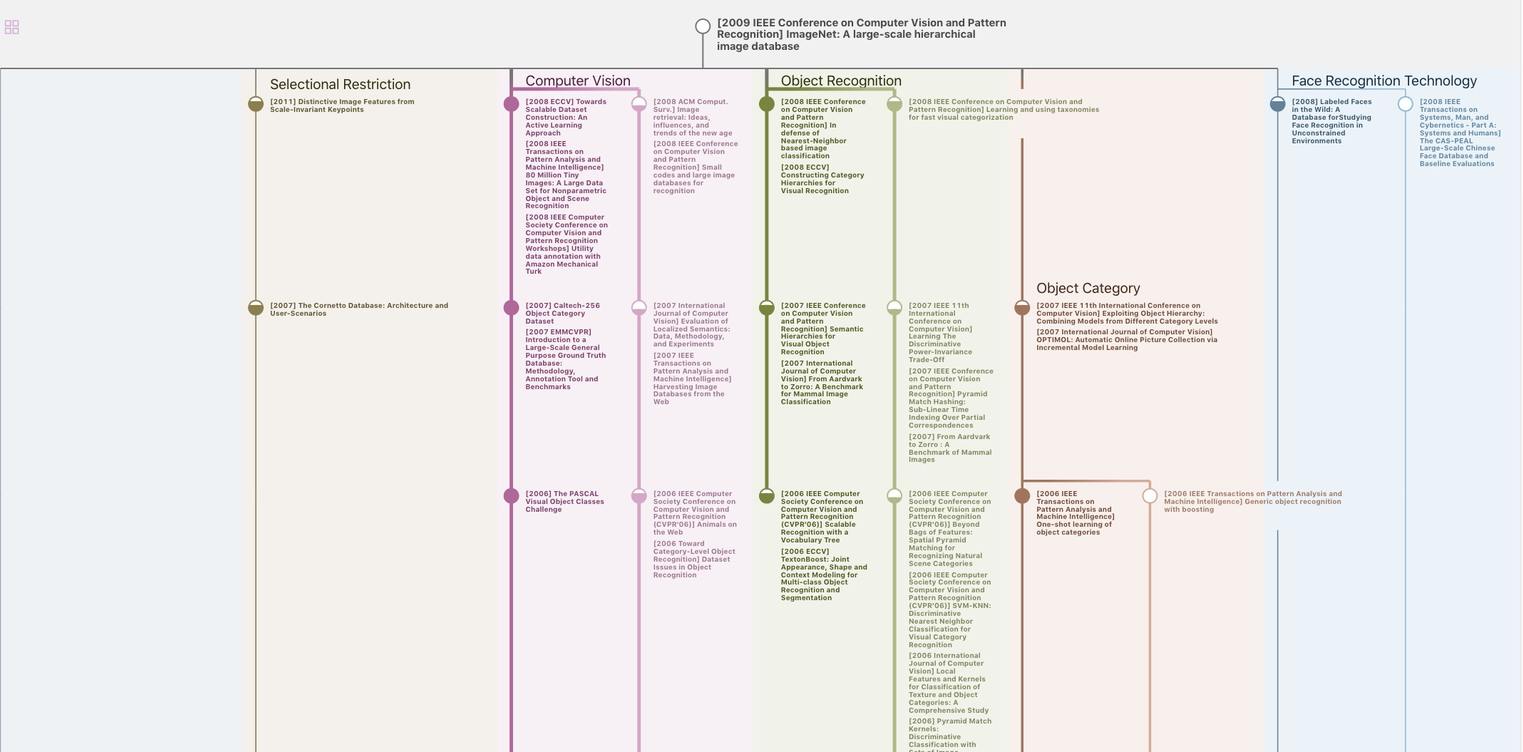
生成溯源树,研究论文发展脉络
Chat Paper
正在生成论文摘要