A GPU-Outperforming FPGA Accelerator Architecture for Binary Convolutional Neural Networks.
JETC(2018)
摘要
FPGA-based hardware accelerators for convolutional neural networks (CNNs) have received attention due to their higher energy efficiency than GPUs. However, it is challenging for FPGA-based solutions to achieve a higher throughput than GPU counterparts. In this article, we demonstrate that FPGA acceleration can be a superior solution in terms of both throughput and energy efficiency when a CNN is trained with binary constraints on weights and activations. Specifically, we propose an optimized fully mapped FPGA accelerator architecture tailored for bitwise convolution and normalization that features massive spatial parallelism with deep pipelines stages. A key advantage of the FPGA accelerator is that its performance is insensitive to data batch size, while the performance of GPU acceleration varies largely depending on the batch size of the data. Experiment results show that the proposed accelerator architecture for binary CNNs running on a Virtex-7 FPGA is 8.3× faster and 75× more energy-efficient than a Titan X GPU for processing online individual requests in small batch sizes. For processing static data in large batch sizes, the proposed solution is on a par with a Titan X GPU in terms of throughput while delivering 9.5× higher energy efficiency.
更多查看译文
关键词
FPGA, binary neural network, convolutional neural network, deep learning, energy efficiency, hardware acceleration, high-throughput
AI 理解论文
溯源树
样例
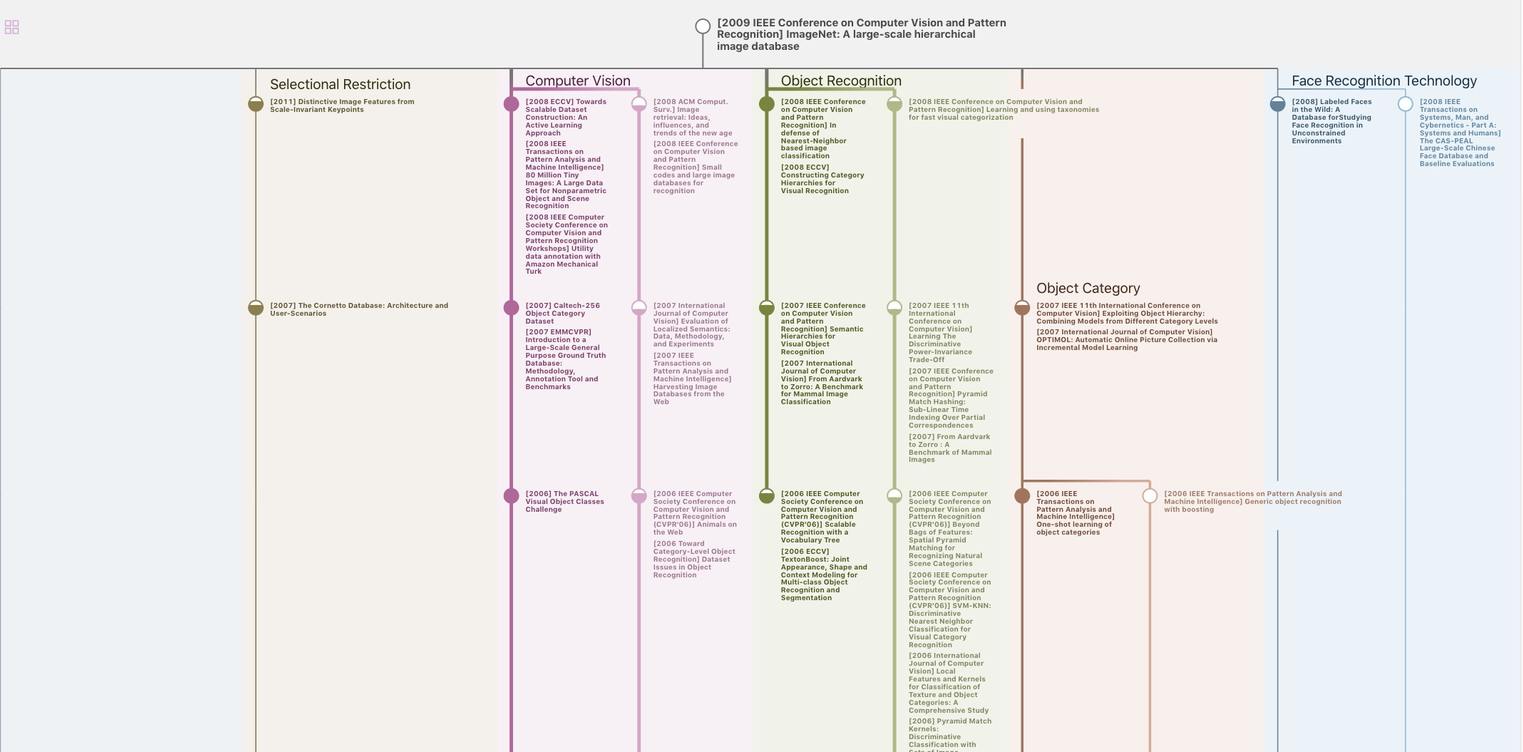
生成溯源树,研究论文发展脉络
Chat Paper
正在生成论文摘要