Hybrid Gbest-guided Artificial Bee Colony for hard partitional clustering
Int. J. Systems Assurance Engineering and Management(2017)
摘要
Clustering is an unsupervised classification method in the field of data mining and plays an essential role in applications in diverse fields. The K-means and K-Medoids are popular examples of conventional partitional clustering methods, which have been prominently applied in various applications. However, they possess several disadvantages, e.g., final solution is dependent on the initial solution, they easily struck into a local optimum solution. The nature-inspired swarm intelligence (SI) methods are global search optimization methods, which offer to be effective to overcome deficiencies of the conventional methods as they possess several desired key features like upgrading the candidate solutions iteratively, decentralization, parallel nature, and a self organizing behavior. The Artificial Bee Colony (ABC) algorithm is one of the recent and well-known SI method, which has been shown effective in various real-world problems. However, it exhibits lack of balance in the exploration and exploitation and shows a poor convergence speed when the number of features (dimensions) increases. Therefore, we make two modifications in it to enhance its exploration and exploitation capabilities to improve quality of the clustering. First, we introduce a gbest-guided search procedure for the fast convergence, which works effectively in large number of features also as it considers all the dimensions simultaneously. Second, in order to avoid being trapped in a local optima and to enhance the information exchange (social learning) between bees for improved search, we incorporate a crossover operator of the genetic algorithm (GA) into it. The proposed strategy is named as Hybrid Gbest-guided Artificial Bee Colony (HGABC) algorithm. We compare clustering results of the HGABC with ABC, variants of the ABC and other recent competitive methods in the swarm and evolutionary intelligence domain on ten real and two synthetic data sets using external quality measures F-measure and Rand-index. The obtained results demonstrate superiority of the proposed method over its competitors in terms of efficiency and effectiveness.
更多查看译文
关键词
Artificial Bee Colony,Data clustering,Swarm intelligence,Hybrid methods,Crossover
AI 理解论文
溯源树
样例
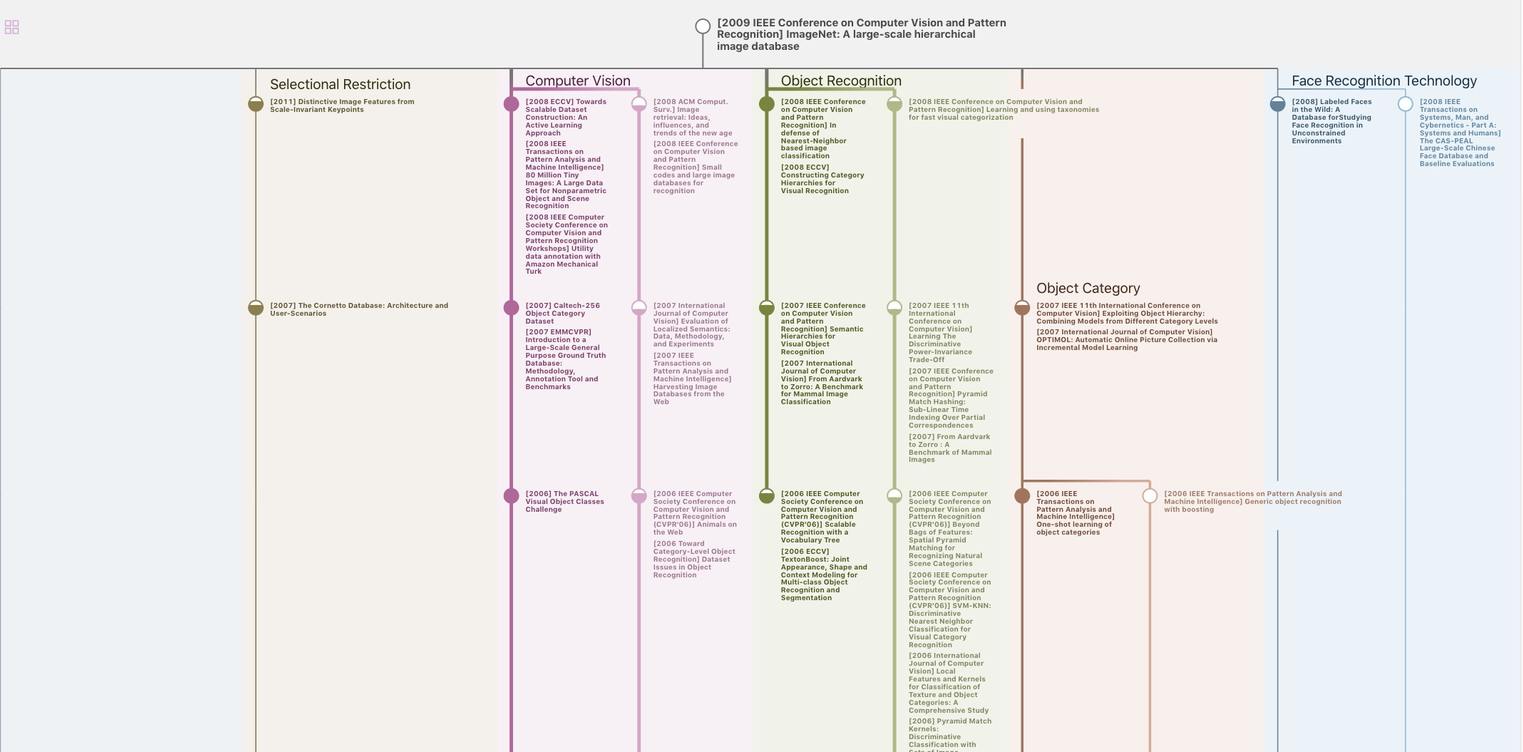
生成溯源树,研究论文发展脉络
Chat Paper
正在生成论文摘要